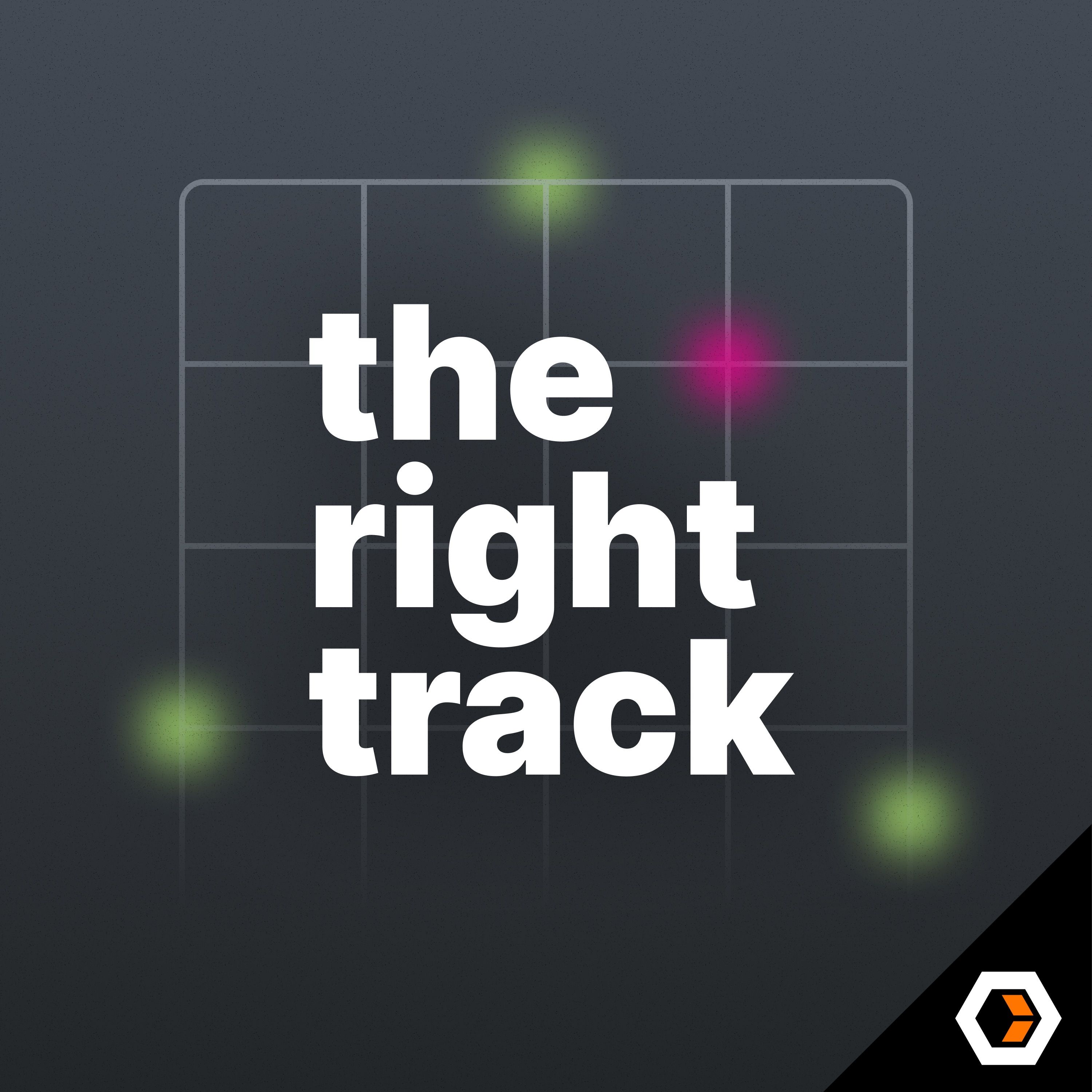
Ep. #1, Trusting Data with John Cutler of Amplitude
In this debut episode of The Right Track, Stef Olafsdottir of Avo speaks with John Cutler of Amplitude. They discuss the growing proximity of product teams to customers, managing organizational inertia, and building better data cultures.
John Cutler is Head of Product Research & Education at Amplitude. He was previously senior product manager at Zendesk and senior product manager at Pendo.
In this debut episode of The Right Track, Stef Olafsdottir of Avo speaks with John Cutler of Amplitude. They discuss the growing proximity of product teams to customers, managing organizational inertia, and building better data cultures.
transcript
Stef Olafsdottir: Thank you for joining me here today, John Cutler.
John Cutler: I appreciate it, and I've been sort of secretly stalking your company so I'm extremely excited to be chatting with you.
Stef: Ah, I love it, thank you.
Likewise, obviously, so it's a mutual stalking. That's perfect.
To kick things off, could you tell us a little bit about who you are, what you do, and how you got there.
John: Sure, so my John is John Cutler. I work at a company called Amplitude.
Amplitude focuses on product analytics, product intelligence.
We're still sort of trying to figure out what to call it.
And I head up our product education team.
It's interesting because I report into our product organization.
I report to Justin Bauer who's the head of product and I have a background in product management in UX research, not necessarily an education, but you could argue in one way or another for the last five, six years either through my writing or through workshops or different things.
I'm sort of-- I obviously like teaching in some ways.
So that's my role at the moment we're growing our team.
We're pretty small at the moment.
I think we're going to grow to maybe eight or 10 folks by the end of the year.
And the mission of the team is to help people achieve their Amplitude related goals.
But also their career goals and their company's goals.
So it's the super interesting thing about this space is that Amplitude is a great product, but if a company doesn't change anything or do anything or act or whatever they want, it's like a tree falling in the forest.
So some B2B products it's like literally the product does it for you and you don't need to pay attention but in analytics or using measurement in general there's this interaction between the company and the product.
So we take a pretty broad scope in what we do.
It means we do workshops, I meet with product leaders all around the world which is really fun.
Before that I was the product evangelist.
Although I wasn't very good at it because I mean, again like I think the product sells itself.
I love our product, but I would focus on the culture the data culture, the impact stuff.
And I think that benefited the company, but they probably should hire someone who will promote Amplitude more.
And then before that had background in product management, UX research, some music and different things.
So that's how I got here.
The last maybe eight years have been sort of more known companies like Zendesk and Pendo, which I worked at and a B2B SaaS company called AppFolio that I worked at.
So that's a little bit about me. I got kind of a late startups doing music for most of my twenties.
I feel really lucky to be doing what I'm doing right now. So I'm excited.
Stef: Amazing, thank you for sharing that context, John.
I actually didn't know that you spent a lot of your early days in music.
John: Yeah.
Stef: I looked forward to grabbing beer and hearing more about that one day.
John: More related we think that world.
Stef: I actually agree with that.
And you are not the first highly skilled product person that I have met that also have a very good sort of musical passion, I guess.
John: Just ask if they're drummer or you figure out what instrument they play and then you'll learn a lot about them before you know when you invite them into your team, anyway.
Stef: Okay, it was a personality test there, I see.
John: Every team needs a drummer, anyway.
Stef: Exactly, that foundation. I love that.
So to sort of get things moving into some of the really exciting stuff I'm hoping to hear you talk about here today.
I wanted to maybe trigger like a thought around--
I've heard stories that your role was created at Amplitude because you were such a phenomenal product person and sort of inspiring to many.
So ultimately what the Amplitude team was trying to do is like, okay, "This person needs to teach the world how to do product analytics."
So that sparked me to think, you know a lot about building data culture and how to use data.
So I just wanted to maybe trigger some thoughts there.
John: You know, it's going to be interesting 'cause I think people come at this from different angles.
I think people might come from like an academic background or might have been involved in stats, so they come from a data engineering back.
But what I love about this space is that there's so many what I would call like literacies and backgrounds.
And my entry into this was purely from, I was that product manager sort of self-teaching all sorts of things.
I was always like begging for the keys to the production databases.
Like I was always that person. I remember crazy effort where I was like, "Oh, I'm going to make huge network graphs of how everyone is moving around the product."
Like I was always a tinkerer for doing this particular thing.
But to be honest, I wasn't particularly skilled in stats or data engineering.
I really had that angle as a product manager as a UX researcher who was just very eager to improve decisions.
And then I'm really passionate about the team understanding the impact of their work.
And I think that I can always sum that up with a story in the sense that I always had an engineer friend who said that, "Well, at the end of the day I can find something to get interested in.
If I'm at a company where I'm separated from the impact of my work, there's always like a new framework or a new program. I can keep myself busy for awhile."
But they said your every two, three, four years, I finally pinch myself and I said, "What am I doing? And is it working? And are we learning about our customers and doing things?"
So that always stuck with me.
I think as this product manager kind of hacking away and doing these particular things and kind of getting better and better at this, that aspect of the team as a team of problem solvers not like factory typers, like that always appealed to me.
And so I think it is interesting though even at Amplitude and in the Bay Area, there's certainly people who go to bed every night, thinking about data, you know data or data-driven or data, things like that.
And I have to be honest like I'm pretty utilitarian about it. I'm sort of like, what the hell are we doing here? Where do we need to reduce uncertainty? What's the why? Who's the human being on the other side?
So I think that some people approach the data culture thing from because they're like extremely passionate about building experimentation frameworks or doing other things.
And I hate saying it, I think there's a lot, like, for example, at Amplitude I think the whole company is the product.
It's like, everything we're doing for people is the product.
But I have this sort of product managers mindset of things like Amplitude is the tools that we use.
Like why are we using these things?
So, anyway, I don't know if this relates to your question but I think that I became interested in data culture and I became interested in this kind of being more impact, focused or using measurement to learn versus to control teams that like these sort of themes that run in my work.
I became interested in that just over time by being in the trenches and figuring out more about the human beings that were around me and then figuring more about the products and then always trying to figure out just a better way.
So it was very organic my approach to doing it or the way that I got into doing this.
I don't know if that helps clarify.
I think some people, they just come out of some grad program and they're like, "This is what I'm going to do my whole life."
But I was certainly not in that camp, whatsoever.
Stef: You're touching on, might be a little bit on the fact that, exactly--
There have been Google has initiated this product manager training culture, and we've seen companies do that since then Lyft, for example.
But what I find interesting in what you're also touching on is we're talking about this from the angle of the data culture, but what we're effectively talking about also is how companies are now building products differently.
And I know we've talked about this a little bit before, but so maybe I'll leave you with this question, as a follow-up of like, data culture and product culture, how are those changing?
How has the industry changed?
I want to say in the last five years and then in the last two years.
John: It's interesting because this is you learn this very quickly in Amplitude.
We have customers that are operating like it's 2006 competing against companies that are operating like 2004 or five, six, seven, and eight.
And we have customers operating like it's 2014 competing against people between 13, 14, 15, 16.
And we have our customers who are building the bleeding edge thing.
And then maybe that effort failed internally and they realized like, "This is not really what we want our core competency to be."
You all are really passionate and focused about this, and they're pushing the practice.
They're operating like it's 2021. So I think that, yeah, so questions about this is always difficult because it's like windowing or you to think about the different companies to do it.
But I think that there's a couple trends that we see that I think are the underlying trends here.
And one is obviously consumers have a lot more choice.
They have a lot more devices and teams are moving super quickly.
And the combination of those things of teams moving quickly and a lot of competition over customer loyalty and then the proliferation of devices, and then the proliferation of the complexity of the experiences are at, are just throwing off an amazing amount of sort of complex behavioral data.
And this is not necessarily a new thing, but even historically it's funny, I was looking at like a Google trends thing.
Even the word digital transformation was sort of a consultancy hack in 2016.
And it was pretty flat until then like Gangnam Style is like 2015. You think about these things.
Stef: Anyone who is building anything digital in 2016 would be allergic and throw a little bit up in their mouth when anyone else used digital transformation.
I think that was happening then.
John: Yeah, exactly. That's really funny too.
And probably to this day, they still do that.
So I think it's a roundabout way of saying these things that like I think that the way that this relates to how the product teams are working is it's just sort of a natural evolution as teams are getting pushed closer and closer and closer to the edge.
So they're the closer and closer to customers. They're closer and closer to these experiences.
They're expected. We ask it, most of our workshops at Amplitude are you shipping faster than you learn and learning faster than you ship.
And it's gotten to the point where about 80% of the people say, "We're shipping faster than we learn."
Now, if you go and talk to a bunch of companies in the regulated industry, it will actually be flipped.
80% of them were saying, "Actually we're learning way faster than we can ship."
So I think that, anyway I'm drawing all these threads together, but you get all this complex data, you get teams operating very close to the edge with customers.
You get this the flipping of the problem where now a problem is more about how quickly can we learn versus how quickly can we ship to do these things.
And I think that those trends proliferated across organizations have all kinds of ramifications.
And so the spectrum I see and I'm sure you see it too, at Amplitude is crazy.
The idea that you'll have a team in that is completely empowered to decide what it's tracking and how it's tracking and governing kind of their own data and doing and managing their own data and shipping in hours and days on one side.
And then on the other side, a massive company that takes three to six months to prioritize the estimation to prioritize the implementation of implementing 100 events somewhere.
I mean, that's crazy, right? I mean, this is literally on team one.
We'll meet with them and say, "Hey can we just get into a test environment going? Let's just run some events. Let's do a hello world. Let's get this do you see how events work? Do you see this?"
Here's the like rough way to think about event properties and things, engineers, product UX are there design is starting to say, "Well, oh, this is the journey. "Let's start getting this."
So organic, like 60 seconds of or 60 minutes, 60 seconds, 60 minutes where the team were working.
And then on the other side we're talking about 60 to 180 days or even longer.
So that's, anyway, there's a roundabout way of saying, I was trying to bring this story back to the end.
All these trends are just all mashing in together at once.
And it's very difficult to say the key trend because of how big the spectrum and the responses are.
But I don't know if that helps, but this is just what's coming to mind this morning.
That it's just crazy the differences that are out there.
Stef: So let me see if I can pull us together into also what's happening, 'cause I feel like if we're talking about how the industry has shifted --
We have certainly a long time ago shifted away from at least in what's considered best practices not necessarily what actually everyone is practicing but for example, the concept of waterfall product development--
Where just like you were saying people are just implementing what they're told to implement versus the other thing, which you describe beautifully, the teams are closer and closer to the edge close to the actual customers and being autonomous building autonomous teams that actually ship products.
And so that's one major shift for sure.
And the part of what I've felt in that shift is like, okay, you have this direction but then you have the teams they're autonomous to decide what they want to build.
That's sort of moved the wheel in like these fast shipping cycle of products.
And you're sort of saying that's also what identifies good data culture is you don't try to track everything and like massively plan to ship 100 analytics events and you won't ship anything 'cause you have everything versus just like just stop somewhere.
John: I think it's funny because so let's say at Amplitude we have three broad categories of customers.
We have very rapid scale-up startups. And then we have tech companies that are maybe 15, 20 years old.
On their second or third or fourth or fifth or sixth act like they're successful in many ways.
Then you've got the third is these digital transforming companies like Anheuser-Busch as a customer.
I mean, he's got these massive customers.
The interesting thing is that by dealing with that range you see that the key challenge and opportunity is different among those three different companies related to it.
So now the rapid scale-up startups some of them are these very well known companies that may be launched in 2010 or 2008 or 2012.
They promise chaos. The chaos that's created.
They don't have a problem with shipping quickly.
They're launching new teams every day.
They got "Oh, we've got a new, oh, we have to launch "a whole new business in South America tomorrow."
They're moving so quickly.
So their problem, and I know some folks who do data product management for those particular companies it's like data discoverability. It's managing the chaos, it's managing the entropy. The experiences are changing so quickly that becomes the problem.
Now the second the funny thing is the ones that have the most trouble with are the second category, because they are stubborn.
They believe that they're the best in the world.
There's like a level of hubris because they've been successful at something and they're a tech first company.
So it's not like they don't have skills.
And so that second category they're very stubborn in their ways.
It's like, "Well, we're going to warehouse things this way. Or we could build that. That's we've got this, we were a big company."
Do these things. Now, interestingly, the third category the digital transformers, let's be clear.
There's so much inertia in those companies.
Those are the ones that it takes three to six months to get things done.
But at least at Amplitude what's interesting is, they're also the most passionate about getting it right.
Even though it's more political and bureaucratic organizations they are truly being disrupted.
For them it's do or die at the moment for them to flip the switch.
And yeah, we can joke about digital transformation or we can joke about these things.
But these are the companies where this is legitimate. This is the real world.
They have to flip what they're doing.
And so I think that's maybe this helps folks listening kind of frame where you're at in those things is, where your company is at is probably the first one is about scale and chaos and entropy and new just all the data sources and everything coming.
The second one is almost it's organizational inertia but it's also just people don't want it.
They are really overconfident because they're in a successful business.
They don't want to really adopt new ways. It can be hard to work in that environment.
It's now the third one, if you're in this big digital disruptor situation is it is about managing the politics.
But really your goal is a change agent to think about a data culture is latching into the big business shifts and the disruption.
If you can do that, you can actually make a lot of really interesting things happen.
So I don't know if that helps people listening like pick which of those environments you're in and then what you have to do in each of those probably changes a little bit.
Stef: I love this classification that you've sort of allowed companies to identify, self identify with. I'm sure then also within each of them, we have maturity levels basically.
And then probably also then each of them we have different types of data cultures.
So I love that we have already cohorted the companies that we're analyzing data cultures for.
But I would love to hear you maybe talk a little bit about what are the things that make the good data cultures at each one of those companies and what are the things to look out for also?
John: I love that you pointed out I'm a really big advocate of contextual maturity models or sort of non-linear maturity models, because I always joke that like every company will have a maturity model and every customer is a 2.5 and only if you buy that product, can you be a five?
And they've got one customer who's a five and that's your story really.
And if you come from science or whatever, the maturity models aren't even a model, they're literally a list of observable buckets that a couple of most of them are not, there's no sort of like science or thought behind them.
It's just like, "What do we observe in a spectrum "once through five and we'll string it out "and we'll bucket it to do these things."
The real question is what is the underlying model?
Like what is the model of improvement contextually for those companies?
So for example, I think that in that first example the rapid scale-up startup with a lot of chaos and usually in those companies there's no doubt about the power of data.
And usually they don't suffer from having really smart people there.
They're swimming with data scientists. "Oh we have to hire all."
Those companies are much more likely to have hired the 50 data scientists you're sitting around in a room, twiddling their thumbs being like, "Yeah we're just cleaning up data all day. And when is the real work?"
And then the CEOs who we've got to reinvent the industry and it's like, "I'm like, Oh, okay "I'll just do model for that."
And then you never productionalize it.
And it's just, so that's the kind of anti-pattern there.
It's like people pop up you don't even know who they are because it's going so fast.
So the positive data culture in that is very thoughtful approach that accommodates the complexity and scaling of the businesses and really understands that it's always about trade-offs.
Like that type of company, if they implement any type of highly controlled linear process around governance the teams are just going to kick it to the side.
There's no, you're not going to impose a heavyweight process in that rapidly scaling environment.
So when I see the companies that are doing well there you see these very thoughtful leaders who understand that nothing needs to be 100%.
If things degrade by 10% but we can still make good decisions, this is good.
They're very thoughtful about how different platforms are emerging in their companies.
But they realize in the beginning, you don't over-manage those things.
You have to let them they're just very thoughtful that in a rapidly scaling environment you have to use a very light touch.
And then when it's important to make important decision you have to make it very quickly and very decisively because there's just so much chaos in doing it.
And so I think as it relates to the data. Let me give like a very, very specific example.
You much more likely in those environments to meet like a thoughtful analytics leader who says, "My role is about scaling data literacy. And I have a partner on the data engineering team, who's really passionate about giving teams the tools they need to."
I always use the as a service technique which is, what is it as a service?
Are you data literacy as a service?
Is this like ingestion patterns as a service?
Is this roll your own ETL as a service?
And so in those rapid scale of companies that are successful, you find these very sort of thoughtful leaders taking a systems approach that doesn't clamp down too much on the culture.
And that accommodates the growth of these particular things.
I know that's pretty conceptual and hard to see, but that's it's almost all you can do in those environments.
I think Maura from Patreon, Maura Church is like a very good example of this because when we did a whole interview with her, but she has people who own things, like their own data quality to some extent.
But the tactics used by the person who owns data quality are very systems thinking oriented.
So if the goal is to get some level of consistency or get some level of things the person who owns it does not sit there and create a bottleneck for everyone.
They actually think about the broad way of using tools and stuff to enable people. They own it.
So I love that balance of like, there is clear ownership on the quality, but the tactics are not heavy handed.
Anyway, so that's an example.
So the second thing, second one like I said, are really, really tough because those companies are successful companies. And they're almost a victim of their success.
We could think of some of these tech companies that are just extremely good at a set of things.
And maybe a positive example of how hard this is, if you think about Microsoft, I mean Microsoft, even though there's this shift over to this thing they really are the best in the world at quality feature factories for a lot of their software.
And they now they're embracing a more customer centric type of thinking.
And I think that's an example in my work with Microsoft.
That's an example of where you see this very thoughtful.
I mean, obviously they've been around for more than 15 years.
But you see this very thoughtful transition from a company that's doing really well with things about moving over.
I don't want to spend too long on this 'cause I feel like we could talk forever about the thing.
The final one, the digital transformers thing. I can give a specific example.
Like we were talking with one of our customers and who's way more successful than others in that category.
And it really is a lot about building a whole like mesh and framework of leaders across the organization to buy into the idea of a more sort of positive data culture and do it.
So when I do UX research with those teams, you find they're pretty bureaucratic and political and things like that, but they've found a way to sort of successfully network all these leaders to be able to do it.
But sometimes for those big digital transformers it's the smallest win in the beginning.
And I think that that's what people don't realize is that this massive company, this healthcare company or whatever may have never had a team, ship something and then have access to the data for something that they shipped that very moment that they were doing it.
So for a lot of those big companies that the positive data cultures are the ones that start very small and then kind of grow organically in the middle of the thing.
Long question we can go any which way you want.
Stef: Yeah, thank you so much. So I'm glad, first of all, I wanted to mention I'm really glad that you mentioned Maura there. I agree, she is a fantastic thoughtful data leader. And I was going to ask you to mention some of your sort of favorite thoughtful data leaders that we should look towards. So I'm going to leave that question hanging until a bit later in the show but also wanted to mention Maura will be on the show soon, so that--
John: Oh, that's great,
Stef: About it.
John: That's cool.
Stef: And we can learn more about I'll pick up some of the stuff that you are mentioning here and make sure she covers all of that stuff.
But I want to maybe or you've talked about the traits of companies.
I think I want to maybe particularly focus on like the first category and the third one, the rapid startups and digital transformers.
The reason why I'm interested in talking about those is while you've sort of highlighted about category two, there is a little bit of a resistance in going in this direction.
John: Yeah.
Stef: So I do want to I am interested in maybe highlighting a little bit further the companies that are sort of on the verge of getting there, which sounds like they're more likely to be in category one and three.
So what is the thing that helps a company that is sort of curious about that or a little bit, doesn't know about it or there's somewhere close to getting there?
What is the thing that really helps them buy into this?
Who can be the champion? Who needs to be the champion?
Or are there external factors that can help? Does it have to be internal?
What are the things that you've seen where people make the decision to actually.
John: I think that one in the rapid scale up example, this is going to sound kind of funny but the one pattern I've noticed is the people who let it be boring for a little bit.
And I wrote a blog post recently, like "Let's just start "by counting some things."
The anti-pattern that environment is that there's so much chaos, there's so much things going on that they don't slow down and realize that it's a journey.
You know, it's like a journey of building some trusted sources of things.
And then you have, it's almost like categories of the day.
You've got things that are really shifting, you may never need.
And you've got kind of source of like GroundTruth type things and you've got that.
And so the pattern that it seems like it's a very like humble and pragmatic approach of slowing down. And there's two things.
One, they don't get caught up on all their historical data. Especially in that environment.
So it's good actually comparing these because often in like that big enterprise example this is very hard for them to where this is sort of like obsession with all their prior data, oh you know what they're doing. And so I'd say, but especially in that first example they don't stress too hard about the historical data. They allow things to be simple for a little bit.
We find this a lot at Amplitude is that I was talking to a customer recently and they said, "For a full year, we were basically counting things and building trust with what we were counting things."
So I think that leader in the first environment knows they've got all these really smart people around who want to do all these advanced things, and they know there's so much chaos and they know all that.
But they just say, "Let's build the muscle to move from data snacking to thinking about how we work."
Like, let's build that muscle so that, a team has the heuristics in place and that they're just kind of instrumenting as they're working.
It's like the shift to test driven development.
It's like, you've got to build that muscle to think about it before you can't over intellectualize it.
Like you just have to do it and you have to build that.
So I think that's like the pattern. I'll pause for a second to see if that's resonating.
But I think that like that ability to slow down, start counting things and understand that this is like a journey is really the companies that aren't successful are the ones that are just running around like crazy people.
And they see these types of tools that some sort of quick fix to prove their strategy or whatever they want to do.
And then they get distracted by something else the next day and do it.
Lets say that's like a general pattern, and I don't know if you've observed the same thing, but it's kind of like level of humility and patience that is can be really hard in a really rapid scale up environment, but it pays dividends, six months 12 months sometime later after to do.
Stef: Yes, so there's definitely absolutely does resonate.
And I think there are two things that I'm interested in bringing up.
I just want to highlight and echo and sort of build on your analogy for the test driven development, which I very much agree with this.
This was a transformation in software development where we wanted to make sure that our code had consistency and quality.
That's something that we're also seeing more and more in the desire off and just data.
But the other analogy was test driven development which I like to use is the companies that I've been consulting throughout my lifetime.
And just when we were building features of quizzes and all that stuff, my most important message was always exactly what you've been saying, like the snack bites are so important. Do not go for a flag day switch over and trying to fix everything at once.
You would never do it.
For example, if you were adding tests to your it's very rare that you just take and stop and pause your code writing for six months to write tests for all of the cases that you would never do it.
You would never do it.
You like, maybe you start with a two, three weeks sprint initiative where you write tests for some of the highest leverage cases and then you build it into the culture.
Exactly what you were saying.
John: Yes, similar to refactoring in that sense that I do think that big bang refactoring is necessary in some small percentage of cases, but the, this is the classic example, especially in the Bay Area.
That the standard thing is new leader comes in, and then they just want to change everything.
So they have political capital at that moment or social capital.
And then they we're shifting to Kafka for this so we're doing something.
And literally the first 12 to 18 months of their time in a company is about some big bang thing.
"Oh, we need to rewrite search completely."
You know, this will never do.
And I think that that same thing happens in these transformations related to the data stuff or the data culture thing is that if the leader comes in and it's like, they pitch this stuff in the first 90 days and they get everyone kind of this is their chance to do it.
And they go in, in this sort of, like I said, the do or die we're going to rip everything out and do all these things.
It's like a real sort of career booster move but it works in way fewer cases than people think it is.
It's like the big bang refactor.
I'm friends with Josh Kirovsky and other folks who were really into the idea that there's many models for refactoring that can be done more incrementally.
They take patients and ones like called the strangle method where you kind of you start abstracting calls and you start refactoring and you kind of break up the model can break this whole thing up.
It takes more patience.
And it's less it's like sexy than saying, you're going to just rip and replace and do this whole system.
But for the people who do it, the results are a lot better.
So I think that there's something related to that.
Like, I really do believe that measurement and metrics.
And I think that there's like almost like a measurement.
I love that your podcast is called like, "You're on The Right Track," 'cause like just how in the quantified self movement there's this idea of like, there's an almost meditation to measurement.
And I think that that actually exists almost, this is one thing the last year I've flipped my opinion on completely.
I used to be as a product manager and I'm like, what do we need to decide?
What decision do we need to make? What do we, everything's going to flow from that.
And now what I realized is that like it's helpful to have those thoughts.
It's helpful to be very pragmatic about that thing.
But there's a certain meditation with just tracking things.
And I love how when you have more of from Patreon, again, like we were chatting with and she was like, "Yeah, we just try to track as much as we can."
That makes sense. Like people are generally know what makes sense to do it.
So I've kind of flipped the, my belief on that in the sense that I think that you should build the muscle to track things.
Whether or not like, I think once you've got some practice you build the probability that what you track will be useful.
But what if it isn't? Or you just get, you don't need to keep it around.
And so I think that that's one of the big shifts.
But prior to products like Amplitude, you really did need to start with a question and then think about how you were going to do the transformations and how you're going to do these things to prepare to answer that particular question was very heavy.
And then you deliver what was called, the self-service dashboard.
But it was really just one table that took a lot of work and took a lot of stuff, and then you can poke around that table.
The big shift in analytics is this idea that like a team making reasonably good decisions about what are domain, relevant events and using a really good system of doing that can kind of proceed with that muscle.
And then it does not need to think about the question in advance.
It's similar to the observability movement in many ways, that stuff.
And so I think that's the seismic shift in our thing which is hardest for people to understand who come from a traditional analytics background where they say, "Well, no, of course this is work flow."
We're going to start with the question or we're going to do that.
And I'm going to track this and then do that.
And a week later or however long you going to do it.
And it shifted to this kind of like you build the muscle for measurement and for tracking and do that reasonably well.
Not everything, but like you just build a muscle for like realizing that's going to probably be helpful.
And then being able to get what we call, at Amplitude, the long tail of insights.
It's like, if you have 100 well instrumented events each with five, 10, 15 properties and some user properties or whatever you can answer a lot of questions.
Like there are tons of insights you could get from them.
So anyway, I'm talking a lot about it, but it's something that I'm passionate about.
Like, I think that that's one of the big shifts that people may not realize exist.
Stef: I really appreciate you going in this direction.
And I think it sort of touches on a lot.
Another thing that you were just talking about in the previous conversation about what do the teams need to do, when you were sort of effectively saying, it's a little bit about starting small.
And I think I want to stitch that together with, okay, yes, I absolutely agree.
It's so important to start small and there are multiple reasons for it.
It is one of them is just like, if you don't prioritize what product you're going to build and start just doing all of them, you won't necessarily end up with the most important thing I don't just time has passed and you have to share.
And then you just like have some tracking maybe.
And so I think that is one of the most important things to keep in mind for when you're deciding what to track it.
Even if you haven't fully formalized the question in your mind, it's just such a helpful way to decide what is most important thing for me to measure at this point in time, and then stop there to track results fast to be able to sort of do some internal marketing of how valuable it is to use your insights low effort insights to make big decisions.
John: I have this very simple formula that I call, the product outcome formula.
It's basically like outcomes are a function of how much data you have, the usability of that data, the quality of your insights, the rate of your insights, the rate of action and the quality of action equals outcomes.
And if you look at the particular challenges that companies have some companies just have never collected the data.
So they struggle with even having it.
Other companies are swimming in data, but none of it is very usable.
Some of them have actually a fair amount of data and it's pretty usable.
And they even have really skilled analysts and people.
So their quality of insights is high.
But the rate of insights moving those insights and the impact that has on decision quality is low. And then finally you get out to the end where you have some teams that are making incredibly quick decisions and acting very quickly but making really bad decisions. And then you have other companies that fret for six to 12 months about making perfect decisions, but they take forever to make them that particular decision.
But back to this idea of how I see that as kind of a virtuous loop and back to this idea of starting small if you're a change agent in one of these environments you need to think about that full loop.
Because without thinking through that full loop it's going to be very difficult for you to have those like you said, those things that are very recognizable to the organization and do that.
So if you spend forever trying to collect everything and just making it reasonably usable, but don't follow through to quality of insights, rate of insights, rate of action and quality of action, and then bring the loop through to outcomes, you're going to have a very hard time.
So just like how we do in product development with the idea of like a thin slice across the problem space, one way to think about being a change agent for data culture stuff is take a thin slice across the landscape of what you need to do.
And don't get overly obsessed with one of those particular categories until you've gone end to end through the loop and then continue to do that.
I don't know if that made sense, but it's the way that I think that.
Stef: Yeah, exactly. Basically creating a full unit of value to present.
John: Right? Yeah, yeah, exactly.
Stef: So this is a really good I think it's a good segue, like turning it back into like what do actually companies need to do to get there?
What is a good example of a successful change management into this type of culture that you remember.
A favorite example of a change agent?
John: Yeah, I think I'm sort of combining an aggregate of a couple of customers I'm thinking about at Amplitude.
But some of the best examples are what I've observed that the pattern was that they did is that, they took a specific value stream in the company.
And they didn't try to answer every question about that value stream, but they didn't try to solve everything for everything like a specific opportunity for the company on a high level, which is--
And I'm talking to a pretty high level, like let's say the company just the number one elephant in the room for that company was around retention or the number one problem in that company was understanding.
In a lot of B2B, for example, it might be the complexity of the product as you go up market.
Like they latched into something that had a powerful story. But they kept the details a little bit vague.
This is the thing if you're a change agent, you need to realize that like I always say separate the why from the way.
The way will change, but the why is really, really, really, really important to do these things.
So the first thing is they found that powerful narrative that really made sense for the particular company.
So maybe the narrative would be something like, "We are being disrupted by other ways of delivering media and like monetization in this new framework is our number one challenge for the company."
Or even sometimes way, way smaller.
What I noticed, it's not about solving the biggest problem for the company but it's just something that you can tell a story around.
So that becomes your why. So they had a why.
And then the second thing is I think that one thing they did really well was create the expectation around the journey.
Well internally. So they didn't go in guns blazing about everything and anything that's going to do.
They really did set the expectation that this was going to be a muscle that was going to be built over a period of time.
So that'd be number two.
So the why not the way they set the expectation of what they're going to do.
I've noticed in general, this is a really funny story.
So we have a customer who some reason product wasn't really even involved in purchasing Amplitude, or let's say they wanted it but then they just gave it to an engineering leader.
And then the engineering lead so I'm on the phone.
And this poor engineer is amazing.
Like they literally have every single event describing where it happens in the product.
Then they give a link to a dashboard in Amplitude.
They've already, they gave sample questions you could answer with it.
And I said, well, what does product think of this?
They're like," I don't know. "They keep saying, they'll check in but they keep delaying the meeting. And I've been working in this particular thing."
And you know, this person had come up with a way of like abstracting a little bit our code to be really usable in their environment.
And they might've even actually related to it where you're all product is.
I think they might even check their like event definitions into repo or something.
So they were doing everything right but they were disconnected.
So the number one thing you can do is to create and this is the pattern we see the Amplitude is that, cross-functional sponsor group with a kind of like a passionate person from design, from engineering, from product management.
And if it's not a traditional digital product company where there's like GM's or it's like other business things like that group of four or five people, they just beat the drum.
You know, it's like that group of people I was on one of these meetings yesterday and it's the same five people who've met every two weeks for the last year.
And you can just see it in the way that they were talking.
Like it wasn't a bullshit meeting. It was really about like, where are we at right now?
What's the one little thing that we need to move.
They treated it like almost like a team treats a retrospective and they're prioritizing new experiments to do it.
So I think that's those are the things that you can do.
The technical details are important, but if you can build and start like that why not the way, you know, if you can set the expectations of the journey and then you can get that crew together, or say you're not operating like this company when the engineers and product were working in completely different worlds.
And then you can set I think it's about setting the expectation for them.
Like, "Hey, we will be meeting for a year every two weeks," and in their headspace doing that.
So, and related to governance and like what they track and do those things, I think that the pattern I've seen is basically this I'll use the example actually, this seems unrelated.
But there's a company that I know that wanted to do redesign for their company.
And they had a choice. They could stop everything and then hire consultants and then try to redesign their whole product.
But they went with is they created like two research librarians in the company.
And they basically said that, like "If you have research about design, submit it to the library. If you submit research, you can take research out."
And from the top of the company, they said, "We will have our product redesigned in two years."
Like if we're going to do it incrementally you think it's crazy, but over time we're going to organically get to these things.
So I think I mentioned that story because that idea of like being a really savvy process designer or work designer, when you come up with your methods of governance to think about like you could argue that scaling is fundamentally a question of what must remain the same and how much you were willing to spend to keep it the same.
A lot of companies fail because they think everything needs to re-stain the same and they lose all their money, trying to keep it the same instead of the thing.
The same thing on a little micro level with governance it's kind of like, what's the lightest amount?
How can we achieve the desired level of consistency not perfect consistency because then that would cost too much.
How can we get the desired level of consistency for the lowest amount of negative effects and costs on the company?
So for example, that could mean shifting from like taxonomy owner must get sign off A, B, and C and things to that, and being like, "You know what, we're 90% right."
So why don't we just write something or have someone like check in, like, we have some tools in the Amplitude that help you do this, that like every week or two just checks in on the state of things and we'll catch the 10% of things that didn't go right.
That could be way, way, way more effective and have fewer, fewer downfalls for the team.
Then we have a clear governor and they give the thumbs up for anything you do.
So you need to be a savvy systems thinking process designer in your company and realize there's no free lunch.
You don't get everything you want from consistency for zero money.
You like, you need to think about how to get that in a creative way.
Stef: I love that you said that both the what you've talked about and particularly getting the right group together and then also in this, how can you optimize for impact versus effort in data governance?
And so I just quickly want to touch on that getting the right group together.
So that engineering story is such a beautiful story from the perspective of the engineer, in my opinion because it's my favorite engineer to work with is the engineer that really has this desire to really be also a data consumer.
It's the engineer that has leveled up from the level of thinking that analytics is useless because it's why would I spend time on it?
It's useless for the end user.
They've leveled up from that into realizing analytics actually fundamental for the end-user.
The reason we can build the best products for our customers and make the best user experience.
And so this is a personal passion of mine in bringing the data consumers and data producers closer together.
I would love to hear your thoughts on that a little bit, and then on the data governance piece just quickly wanted to say, like I think you really hit the nail on the head right there.
This is something that is I don't trust this data is such a common statement.
It's something that people hear verbatim just all day every day, and you're touching on the path to really get things to a better place.
And it's again, just like with general product analytics it's not a flag day switchover but the journey to getting there is really intriguing with us for some journey.
I know we've talked about this.
John: Yeah, a couple of thoughts. So the data trust thing is very interesting.
And a couple of things come to mind.
I'm even thinking about Amplitude in this case as quote and unquote like self-service analytics or something like that, is that one thing analysts have who are sitting there, when you learn how to query data and how they're querying it, you touch it.
You start with the table and you get 1 million records and then you add one clause and you get 992,000, then you can figure out what happened to the remaining things.
And so I think that one of the interesting things is how to build data trust also when there's different people with different skill sets and often who are not sitting there touching the thing.
I think that people underestimate that as an important thing.
So we actually, that's a product challenge for us, for example, at Amplitude.
Is that because we're offering these self-service analytics you get this kind of business user and they've never touched it.
They don't even know in their mind what event stream data looks like.
Their version of data is an Excel spreadsheet with columns on the top.
And they've probably never even had an Excel spreadsheet with a timestamp on it.
Someone has already said, "This happened on January 15th."
You know, like they've never even they wouldn't even know what to do with that in Excel.
It's actually pretty difficult to go into Excel. At time series data, and do something that's sensible with.
So I think that, so say you're an engineer or you're product manager or someone who has had that experience.
The first thing is empathy. And I've had this a lot in Amplitude is realizing that so much of my trust in data is having in the past worked with it is having a mental model for what it looks like under the hood.
So when I go to companies and say, "Oh yeah, you know just we'll do this quick workshop."
And you just instrument things that make sense from a domain perspective and everything will be okay.
It's interesting 'cause engineers often realize that because they, especially if they've worked with more event based data or they've worked in an event sourcing model they immediately understand, okay, so we get something if we know when it happened and we know what it is and we have a timestamp and we know how it's related to other things, there's many things we can do.
We can figure that out.
So the first thing I think so many thoughts about this, but this was the one I was thinking about this morning is data trust is there's a psychological element to it. And there's this tactical element.
And in these environments with a lot of users who have never touched the data, done these things.
A, first is empathy, you know, do that.
And then second is being very, very, very deliberate.
The various products that help you do this about letting them visualize what events are made is so we added this kind of like event explorer thing in Amplitude recently.
And the pickup has been really amazing.
And so you basically like single out your user and then you use your product and you see the stream of events.
And one of the interesting things is the number one value of that my mind is that is trust.
Like people understand that if a human being does this in the app, me, the human being did this and the app it sends this payload of things.
And here's what the payload looks like. And it does these things.
So that would be one suggestion in your environment.
If you're one of these leaders to build that big data trust thing.
You asked like there was a couple of questions in the one but the one around the data trust thing is important.
I think also the other tip I have for the data trust thing is acknowledging that we don't need to trust all data equally.
And so part of the problem is this environment where you're not coaching the external folks, you're not coaching the other members of the team to realize that there's different classes of things like it's okay to be in an environment where you have some, 40% of this stuff, you're just kind of dumping it somewhere and it's more exploratory and you're figuring it out.
And then you shift it into being.
So one thing you can do is that we see a lot in our customers is some customers really working hard to bless a series of events once they've crossed some threshold of trust internally and then that helps other people.
So then they teach the other people who are using the product that like, we've given this a second thought we've done this.
And the final one is related to the first a little bit is empowering people to explore the data themselves, which is kind of related to what I said about, running the event experts.
So I know there's a lot around data trusted. Did any of that help?
But like those are the more sort of like tactical things that I've noticed around data trust.
That it's easy to be like, they don't trust it. We haven't trusted our data forever.
But the hacks you see successful companies do it's all sorts of cool experiments like that.
Like running different classes of data, teaching people how to explore the data themselves, letting them actually see what payloads of data look like, doing sample charts that start broad and then allow people to go successively down all the way to some other numbers.
So those are a variety of techniques I think.
Stef: Thank you for sharing those. Those are actually a really good tactical advice.
I mean it, and I think one of the things that I've also enjoyed doing with my team personally, is having data taking sessions with some missions.
John: Yeah, I mean, that goes actually to this thing about cadence and rituals, which I think is important.
So whenever I work with the product team and we do this at Amplitude too, you have to set up a regular learning review where you're kind of like the data jams where people are a safe environment to kind of muck through the data themselves and see these things.
You also see this is an example actually.
So Amplitude has different integrations but some people, we have a query product which does actually let you query the data.
But what we've found is that there's advanced users of that feature, but then people like set up little workshops to sort of for their team and be like, "Have you ever wondered what goes on underneath the hood?"
And these are like experienced analyst or data engineers or whatever set up these sessions.
And then they like teach people the lightest amount of querying so they can see like, this is actually a table of data.
Like you can look at the stuff underneath the hood.
But the learning reviews and data jams and I do this activity a lot.
I was just actually coaching someone from one of our customers to do that is instead of the analyst kind of going to the kickoff with the business and sitting in the corner and taking notes, I coached the analyst to do an activity with the folks there that was more around like journey mapping or events storming or kind of question elicitation.
So another way that you can kind of build that trust as well is to get the people who are a little bit more experienced in a more facilitatory role, and then like bringing people along with that process, because if you attend one of those kickoffs and the analyst doesn't say anything and then five weeks later, they give you this dashboard and you've not been a part of it.
But if the analyst comes in to facilitates an activity with the group, like another method is design a dashboard.
Like you use markers and say like, what will the mission dashboard look like for this?
And you've got these business users are drawing with marker whatever, but then if you can go from that to, okay, well, here's the events that are going to sit underneath that they were part of it versus just the analyst sitting at the side and the engineer sitting at the side and then they go into a whole other meeting and be like, "I think we're going to need to track these 12 events and to do these things."
So I don't know, those are some ideas.
Stef: I love that, and I think so we are getting close to a good amount of time talking together, but I want to at least frame what you just said because I think it's so important.
And do you imagining again it's about getting the right group of people together.
And I feel like this is my mantra, data producers and data consumers, they need to really work together and ideally become sort of a part of each other's group.
The analysts, the product managers who consume the data.
They also design the data a little bit. And the developers that actually write the code to get the data points into the database.
They also decided that a little bit and then check out some charts. So getting those groups--
John: We did also that when I think in that model that sometimes people forget is you need people with customer domain knowledge.
And so I kind of break the domains into there's the customer or user domain completely agnostic of the product.
And then if you imagine a set of Venn diagrams that like, then there's the overlap between those needs over the domain of the product.
Like the actual sort of physicality, whether it's virtual or not about overlapping the customer's domain and their needs over on the top of the product.
And then interestingly, you have the overlap between the knowledge of the product and what it looks like from a purely customer focus thing to the underlying engineering.
Like what is the code that produces that interface?
And then if you're putting data somewhere you have the domain of like all the guts and the engineering about where you're going to be putting the data or a system like Amplitude or something.
So when you put it that way, what's really important about that distinction is having a tester, or having a support person, or having another person in the room when you're thinking about this stuff. Like a tester who has a great knowledge of the interface and then actually has a fair decent knowledge of the underlying code that produces the interface is an amazing resource because they understand all the like edge cases.
Now having a designer who really understands the human out in the world and understands the interface at least from a conceptual level on a non worst case scenario situation having them in the room really helps.
And then we found this at Zendesk a lot too.
You have a data scientist who's a search involved in search.
I really try to make an effort to try to get them in the room.
And we are kicking off a new effort to listen to the customer interviews because a data scientist who has no exposure to the domain of the customer and the user will not be able to make good decisions about what they need to do.
I think my point is it's even more nuanced than, we need an engineer, we need a designer and we need a product manager and the thing.
It's less about their titles and more about their awareness of those different domains from pure product adversity, to the overlap of product needs over interface to the overlap of interface over the code that produces the interface and then the overlap and the end of like where the data gets put.
So it's almost like you need five hats in the room or something.
And so I don't know if that helps people, but try to get those, how will just invite a customer to I'm like, why am I like do a kickoff with they've got this thing.
So that helps you ground your events in the customer domain.
The biggest error that I see is that it's like instrumenting code paths and you don't really, and to actually that point is, they're measuring code execution.
They're not measuring the interaction of the human domain across the product.
So if you were to show a list of those events to a human being who uses their product, they would say, "I don't click on nav button three? What the hell is nav button three. I'm trying to go and see your careers page."
Or something like "I'm viewing careers."
And of course, nav button three is a sham anyway, 'cause on a responsive layout, nav button three goes to the nav button four and disappears.
So that's why you need all of these hats.
You need all these hats around and then it becomes a more like creative human activity.
Not necessarily like a specification type activity, once you get everyone there.
Stef: I love this. This is so good.
I love that you summarize and highlighted that it's not about titles.
It's about, you're sort of bringing in all of the different knowledge areas into the room to design the best data.
This has been absolutely fantastic, John.
I feel like I want this to be chapter one out of 80 or something?
John: Cool we can do it again and do some other type of event or dig into one of the topics more would be really fun.
Stef: Absolutely, I really appreciate you coming and sharing your knowledge with hopefully some people out there that are trying to get closer to having, insightful data culture that is enabling them to build better experiences for their customers.
On that note what do you want to leave people with?
John: I think really I would leave the two things.
We really discussed a lot about being a change agent.
Like all the different things and give some models for how you might need to change or in a little differently in those organizations.
But I think that type of thinking that we ended with, with thinking about the domain of the humans out in the world and trying to make that a part of what you're doing is probably a topic I'd love to explore with other folks here.
I'm basically, it's a request like that's what I really think is the important missing link in a lot of these.
Even when you have very skilled people, et cetera, to do it.
So yeah, I'd leave people with that question is like, how are you going to bring the various literacies and bits of domain knowledge into those things?
And I think once you take care of that, it actually solves a lot of the problems that we have with data trust and other things you're doing.
Stef: Love it, yes, I do recommend following John on Twitter.
He's a very interactive and engaging person.
Lot of jotting down thoughts and getting people to inspiringly answer them which I love it.
A lot of interactive communications.
But thank you again so much for joining us today, John.
John: Sure.
Stef: I really appreciate it.
John: My pleasure.
Subscribe to Heavybit Updates
You don’t have to build on your own. We help you stay ahead with the hottest resources, latest product updates, and top job opportunities from the community. Don’t miss out—subscribe now.
Content from the Library
The Right Track Ep. #11, Defining North Star Metrics with Che Sharma of Eppo
In episode 11 of The Right Track, Stef Olafsdottir speaks with Che Sharma, CEO and Founder of Eppo. This conversation includes...
The Right Track Ep. #8, Defining the Data Scientist with Josh Wills of WeaveGrid
In episode 8 of The Right Track, Stef speaks with Josh Wills of WeaveGrid. They address common misconceptions about data and...
The Right Track Ep. #7, Data Translators with Benn Stancil of Mode
In episode 7 of The Right Track, Stef speaks with Benn Stancil of Mode. They discuss the value of analytics for the end user, the...