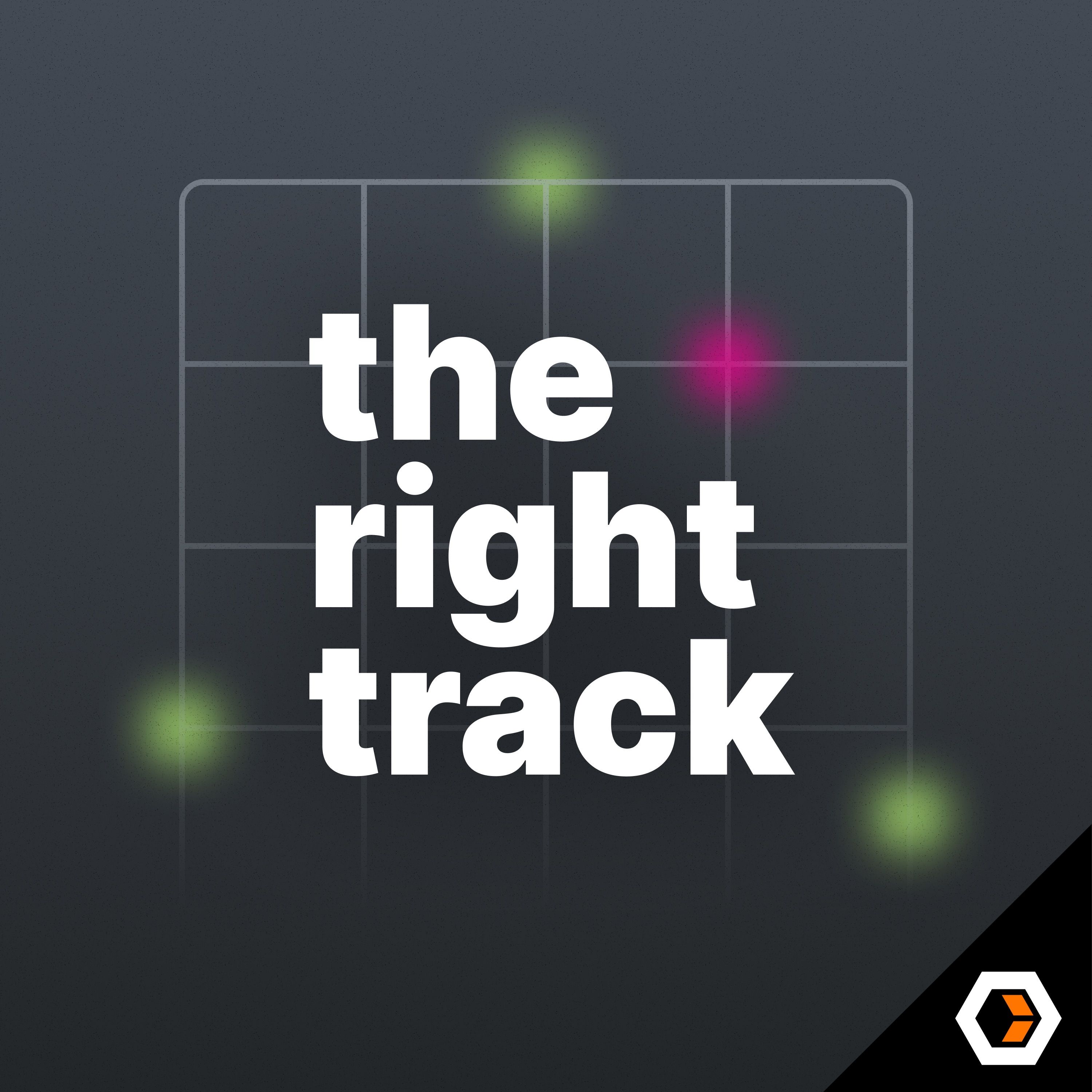
Ep. #11, Defining North Star Metrics with Che Sharma of Eppo
In episode 11 of The Right Track, Stef Olafsdottir speaks with Che Sharma, CEO and Founder of Eppo. This conversation includes many unique insights from Che’s experience scaling Airbnb’s early data culture, as well as lessons on leading data teams, defining a good North Star metric, and leveraging experimentation tools.
Che Sharma is the Founder & CEO at Eppo. Previously Che was one of the first data scientists at Airbnb and the second data scientist at Webflow.
transcript
Stefania Olafsdottir: Hello, Che, and welcome to the Right Track. Great to have you here.
Che Sharma: It's great to be here. Thanks, Stef.
Stefania: Super exciting. Could you tell us a little bit who you are, what you do and how you got there?
Che: Sure thing, we'll cover the whole story. So my name is Che, I'm the Founder and CEO of Eppo.
We're a next generation A/B experimentation platform, one that's really focused on analytics and one that really aims to unlock your culture of entrepreneurial energy.
Previously my background is in data science, I was an early data scientist at Airbnb, I was the fourth on joined in 2012, back before we had most of the infrastructure and capabilities that you see out of that team and saw a lot of the growth journey.
From working on things like production machine learning, analytics infrastructure, actual analytics and data tools.
I also built and open sourced this thing called the Knowledge Repo which was like a collaborative notebook platform for doing strategic analyses.
Then after that worked at some more growth stage companies, most recently Webflow as the second data scientist before starting Eppo.
Stefania: Huge. Thank you so much for sharing this. So for anyone listening, I am sure many of you have followed the Airbnb blog for data science online.
That was at least a bible for myself when I was Head of Data Science at QuizUp. Che and I have previously talked about this feeling when you are feeling a little bit alone as a data scientist, you don't know what you're doing and it was just such a huge validation that what we were doing at QuizUp, we weren't just the only people doing it.
I so often enjoyed blog posts from the data science team at Airbnb where you were sharing that you were currently doing and I was like, "Oh my god, that's exactly what we're doing. That's great. If they're doing it, it must be good."
Che: Yeah, exactly. It's a really common experience. I remember when I was at Airbnb, we would talk to data teams from... I remember at Square they had a really good fraud detection machine learning team that we were talking to.
We looked at Facebook and LinkedIn, some of these places that are much further along the journey and, yeah, it was a real cathartic moment once we started pushing out stuff that these places didn't have and acknowledged problems that were being long ignored.
So yeah, very common when you look at people who are closer to the end of the book than the beginning, and you can hopefully learn from that.
Stefania: Exactly. So important. One of the things really that was inspiring for me was the Knowledge Repo, so thank you for taking the time for open sourcing that. That was a great contribution.
Che: Yeah, definitely. And that was exactly an example of something where we kept facing this problem of people having to repeat the same thing over and over, of not being able to broadcast results effectively, the shareability gap, all that.
And as we started working out our own little, hacky solutions for it, and the early stages were very, very hacky, it was just interesting to notice that all of these companies that we looked up to also didn't have great solutions for it. So the world of data, it's always changing, there's always new problems to solve.
Stefania: Yeah, awesome. I will touch on so many different parts of your really quick intro later, hopefully later in the episode.
But to kick us off into the world of data and proof we are humans that have dealt with terrible things and great things in data, I would love for you to kick us off with a data story. An inspiring one and a frustrating one.
Che: Absolutely. I definitely have my set of frustrating and inspiring ones.
I would say the most frustrating data stories to me, one of them was around getting the very, very basic analytics and instrumentation, and I think this is something that obviously you at Avo think a lot around.
But one of the things about being in data is you're keenly aware that some data is more valuable than other data, there are some data sources that actually tell you the story of the business in this really core way.
For example, if you're Airbnb, the data of when a booking gets confirmed is very important. It underlies a lot of things you do.
Then also there's some things like whenever you look at a listing, that's also an important piece of data.
So I think a really frustrating thing is when the org doesn't treat that data as seriously as you, and so that means do they gather it?
Do they make sure it's resilient and that it's always going to survive? And so at Airbnb, we actually ended up building a system that I think has a lot in common with what you're building in Avo, just because this problem was so big.
I've worked at other growth stage companies where they were early on in their journey, they didn't have a stood up set of interim processes to gather this data properly.
It's just very frustrating when you have to essentially wage a political campaign to get basic data tracks.
Stefania: Yeah. And you're touching a lot on data culture which is my favorite thing to talk about, so I'll dive definitely a lot into that in this episode.
But a fun fact also on that, that you had to solve this at Airbnb.
That was one of the things that we did before we were starting Avo, was talk to hundreds of people about this problem and it was really only the hugely successful organizations that had had time to build something like this.
And when Avo went through a Y combinator, a big part of that was that Gustav Alstrumer, a fantastic Swedish person living in San Francisco for-
Che: I know Gus very well.
Stefania: Yeah. Exactly, right? You probably worked closely with him. Shout out to Gustie.
Che: Yeah, that's right. Gustav's the man, love that guy.
Stefania: He is the man, yeah. I remember when we did the Y combinator interview, I should do a video that represents that experience. That was hilarious.
We ran in there and you only have like a few minutes and we were trying to tell a story around what we were trying to solve, and Gustav's first spoken sentence in the interview, just a few seconds in he was like, "I've had that problem." It was so good.
Che: Yeah. In terms of the inspiring side of the coin, I think the moment you as a data person know that you're starting to win is when you start to see individual product teams naturally start to build data into a first class system, metrics as a first class system.
And so for me at Airbnb, a really cathartic moment was we had this one team that was starting to run experiments.
It was the search ranking team, and they made progress here and there and they started building up the infrastructure along the way.
Then they started showing a lot more wins and that spread to their immediate adjacent teams, the search marketplace team, and they started launching at a lot of things that now we take for granted.
Like the removing hosts that always say no to bookings, or creating messaging that says this is a rare find and that it's actually good to book it now, a lot of marketplace friction things. In the end we actually were able to reaccelerate Airbnb's growth.
So many companies on Airbnb's space, they are growing like rocket ships but at a steadily decreasing pace, so they start off 3X, 2.8X, 2.5X, something like that.
We actually reaccelerated that growth and this one team basically proved that it was because their experiment centric strategy.
The really inspiring thing then is that if you look at how that team operated, everything they do in terms of how they prioritize, in terms of how they justify was so data centric, where suddenly whenever you're talking around a product idea you'd say, "We can do some basic heuristics and understand how many bookings we get."
And so suddenly data's no longer this lagging, chasing function to try and get people into a metric worldview, it's been just built into the core DNA of a team, and then to see that show up. So this in our overall metrics was very cool.
Stefania: Yeah, that's awesome. You've talked both about the frustrating part having been getting the right data in place and then a little bit on establishing an experimentation culture.
I guess this is a little bit related to that, the inspiring story was when you finally got to that stage, right?
Che: Yeah, exactly. There's really a moment when experimentation becomes a core workflow that just unlocks all of the culture a data team strives for.
Something I always say, and it's obviously very correlated to my career decisions, is that data teams really, really need to establish experimentation because it builds this intimate connection between product teams and metrics.
If you think of all these data teams that are not running experiments, all they have is a product team has to look at the overall number of bookings, the overall number amount of revenue, maybe use a few user segmentations.
But they don't really know how much they contributed. They don't actually know if they actually improved anything.
Experiments build that connection and there's a lot of product teams that really embrace that.
They don't want to waste time, they don't want to waste all these resources on something that might not actually be a good direction.
And so given an ability to validate their own decision making and then build the right things afterwards, they're going to embrace that, and so as a data team I always say the core mission of what we're trying to do is to improve everyone's decision making and the best way to do that is actually with experimentation because then you can actually evaluate whether good decisions or bad decisions are happening.
Stefania: Yeah. And obviously quality data is a really important input into that sort of decision making.
Che: Yeah. One of the things I think is fascinating here is that the way I think that a company starts to invest in their underlying data foundation is they first have to feel the value of it on the other end, and so that can happen with really top line things like revenue and you often see these companies get a hold of that sort of metric earlier on.
But if, for example, you want logging of instrumentation to be a big deal then you need more teams using that data to actually use it in their daily process.
So I always think that certain projects like experimentation, like machine learning, any sort of data product, their benefit isn't just the literal thing they're doing.
It's all the upstream data quality improvements that come immediately after, where just by building these kind of interfaces, these workflows right in front of people, they can say like, "I would really love to have better data underneath it and I'm going to start investing."
Stefania: Oh my God, I could not agree more, Che.
I know we're really aligned on this, so I hope it won't be too much us preaching to the choir a little bit this entire session. Yeah, I couldn't agree more with this and I think you have also talked a little bit about that.
I mean, you've released some great content on how people should think about metrics and your thoughts on how some of the metrics Airbnb chose were high level enough but specific enough that it helped various different teams at Airbnb, from the growth team to the product teams, to the marketing teams making really good, impactful, long term decisions for Airbnb. Can you talk a little bit about that?
Che: Yeah, definitely. I at Airbnb we had certain advantages as a business model, and I think most marketplaces end up with this advantage for a data team which is you have this metric which is purchases or bookings, marketplace clearances writ large, that are just really great North Star metrics because when you have a transaction happens you can know that the marketplace did what it's supposed to do.
You know you need to have some downstream checks on the quality of that transaction, like review scores or whatever at Airbnb.
But by and large you have these great North Star metrics and for a lot of other companies, like if you're a SaaS business or some other type of business, you don't have that sort of clarity on North Stars necessarily.
You might have something MRR, something that links in to monetization, but you don't have the same like, "Man, if our users did a lot of this we would all know they're getting value out of our product."
So I actually think this is one of the important things that data teams have to invest in. That's actually why I think you should start a data team, is to help you arrive at this answers.
So for example, at Webflow, Webflow is not a marketplace. It's a SaaS business to help you build a website from scratch with this powerful, no code editor. We often measured things with AR/MRR, but there is a lot of people using the free product who are getting value and it's good for us to increase value in the system.
We eventually found these metrics which worked really well. One of them was how often are people publishing and getting views on their website?
This takes a perhaps opinionated, but I think generally acceptable view, that websites exist for people to view them and so once people have actually shared it out then you know that it's a good website, they thought it was worth sharing.
So these are the sort of metrics that once you have something that is both connected to business outcomes and also viscerally shows customer value, you can do so much better data work.
You can talk about the behaviors that predict it, you can talk about experiments that boost it. Again, understanding user segmentations that more naturally get there.
There's just such a more harmonious thing that happens, but yeah, a good first place to start is to say, "What are the metrics that we care about?"
And I think once you do that, as you can tell I often flit between the organizational problems and technological problems, but once you actually nail down these metrics then all these other kind of modern data stack choices that are happening where those metrics are probably going to be modeled in Bitquery or Snowflake or Redshift, because these data warehouses are the natural place to combine different data sources.
So suppose you have Stripe data and you're trying to combine it with your internal user tables and maybe some clicks, there's only place you can do that and that's in these data warehouses and that increases the desire for workflows that put Snowflake or whatever at the center of the world.
Stefania: Absolutely. And like this, the discussion of the Metric Slayer really fits in here.
But I want to also just because you've brought up really great examples of metrics and I think this is an interesting subject matter for this audience.
So you mentioned nights booked for Airbnb and then you mentioned pages published for Webflow, right?
I don't remember if you mentioned it in your intro this time, but you were there as well, Webflow, so you have vast experience with some really fantastic data teams there and Webflow is one of them.
Which is interesting because DoorDash, would we consider that a marketplace? Yes, probably. And they have transaction metrics which sound similar to the nights booked.
I think at some point at least I stumbled upon something and hopefully someone from DoorDash can confirm this or reject it, so they have something like weekly or daily deliveries without issues.
So they have deliveries and then they have measurement on whether there was any issues like delays in the delivery or something missing from the order, or something that, or a complaint about the deliverer.
Then they have input on metrics into all of these that are very top of funnel oriented, but also number of issues surfaced and things like that that are aimed to optimize this.
So I think that type of metric, and then also with the Webflow metric of weekly published pages or sites, and then having some sort of a qualitative indicator for whether it was a valuable publish is a really good strategy for designing metrics like these.
Che: Completely. The DoorDash example is actually interesting for a few reasons.
One is that they actually are a three party stakeholder exchange, where you've got the restaurant, the orderer and the driver. One of the things, the nights booked thing is a nice, easy metric for Airbnb.
It can take some effort to come up with these metrics, how do you interweave them? So for example at DoorDash, you would have these situations where orders goes up and revenue goes down or something like that.
You have to care, what are you going to do with that? What happens if a product increases orders and decreases revenue? Again, I think this is actually what data teams exist to do, is to craft the metric space, figure out the stuff that matters, and help guide off the trade offs between them.
This is tough work that also ties to the business model. Where I think data teams are losing a lot of productivity is once you've defined a lot of these metrics and everything that's under them, rebuilding the same downstream workflows and analysis tools that exist in every single company.
For example, I don't think many people say you should build the Eye Tool at every company, and so people get Tableau and Lookr or whatever, but you still see that type of thinking applied to a lot of other spaces and so I think that's probably something that will change.
Stefania: Yeah, this is actually a good segue into that. I know I want to talk a little bit more about the metrics culture just in general with data culture, because what I think is interesting is this discussion of who owns the metrics and I think that touches a lot on basically work structure.
So we'll leave that for later, but this was a really great segue into how the industry has changed, and I think you have a fun take on that. Can you talk a little bit about that?
Che: Yeah, definitely. I think a big part of this is just what I have observed from my time.
So when I was at Airbnb we were very much a build over buy culture. It's hard for me to argue whether that's a good decision or not.
It led to things like we created Airflow, we created the Knowledge Repo, we built our own experimentation infrastructure.
It was great for people like me to learn how to build these things, but it's also questionable now where suddenly there's been this explosion of data tools.
I think if you are the leader of a data team this question is a lot more nuanced, it's like what do you build? What do you buy.
Stefania: It's more nuanced today than it was then, you mean?
Che: Dramatically more so. There was no Snowflake when we started and so we had to home roll our own data infra.
It started out with a pig cluster and then we consolidated on Hive, we were running things through Cronjobs and now we have Airflow or whatever.
But nowadays I think it's difficult to justify building your own data infrastructure when you have great products like Snowflake and Bitquery, et cetera, so that's already one example of it.
But even besides that, think of all the pipes you used to have to build to get your transactional data into your data lake.
Now you can just get Fivetran, or Rudderstack or whatever. We had to build our own logging infrastructure, and now there's Segments and so on and so forth.
Like the reverse ETL tools or all the Salesforce pipes. So now there are options to buy really good products for a lot of these things and I think you start to see these data teams start to have larger portfolios of data products which is great, because I think that's actually now a productive behavior.
Then also it brings to mind what are the things that they are rebuilding every time? There's some things like providing business tables for all the metrics, what is an Airbnb booking? What is revenue?
I don't think that stuff is really going to be able to be outsourced because it's all a results of bespoke decisions made by engineering teams.
But stuff like experimentation, it's like, "No, it actually is the same at every place."
It starts becoming increasingly questionable why you have build these experiment infra builders at every single company to have the culture you want.
Stefania: Yeah. It's basically always a question of perceived impact versus perceived effort of adopting a solution like this and what it will give you because it's like you have some estimate of what you might be able to build yourself, and what that could accomplish for you.
Obviously you'll probably always have to multiply that with at least P, Pi is how you say it in English, P is how you say it in Icelandic.
But at least you have some sort of an idea of what you will get out of building yourself, and I think this is probably one of the biggest impacts typically into a build or buy decision.
It's the perceived effort and perceived impact of a solution that can be a little bit more vague than your understanding of what you can build.
Che: Yeah, hopefully. And there's lock in clause and stuff like that.
One of the things that I'm curious if you've also seen, is as a fellow data tools founder, you can always feel good that the people who have actually built them before are usually the ones who are most readily wanting to buy.
The ones who've actually gone through your process of building it, you're like, "Oh man, this is a lot more complicated and I never actually get my headcount back."
Stefania: Exactly. Yeah. Most of Avos' first customers were the people that we had worked together with at QuizUp, who they cried almost when we told them we were building this again, right?
Che: Yeah, completely. So yeah, I'm glad that we, as completely unbiased data tool entrepreneurs, could come together and say everyone should buy our stuff.
But getting back to the metrics conversation, I think that one of the things that I'm starting to see happen, I'm curious if you see it as well, is that when I was at Airbnb we had a data science team and it really owns a lot of the thinking of what were the metrics that mattered? How do you drive them? What are the inputs to them?
Because data was such a foreign thing, it really took someone who was native in that world to actually drive these conversations.
But one of the things I'm noticing now is that the product world is getting so much smarter, where suddenly you have things like Reforge or Lenny's Newsletter, these things that can help you arrive at really good practices, that teach you the language of data.
Some of the classes they have are like, "Most businesses can be understood by activation, engagement, retention, et cetera. We can't tell you what metrics are for your business for those things, but you should think about those and come up with them."
And that's so much what the science of the data science team was, was saying like, "Here are the core ways of measuring business, and now we're going to try to log stuff out."
So I think that this metrics conversation and how you go about moving them is starting to become much more of a collaboration with data and product in a way that I think is very, very cool.
Stefania: Yeah. And because you asked what and said you were curious about what my perception of this was, I definitely agree.
This is also just a part of my philosophy, and you touched on it a little bit in, I think whether it was one of the frustrating or the inspiring stories, just as having managed a data science team before there was any interest or passion in the team about using data.
Then going and changing that culture into people being curious about data and wanting to use data, and just gradually build these internal case studies and basically internal marketing of findings to create curiosity and like, "Ah, okay. So I might be able to do that as well."
And just generally making good decisions using data, it inspires more people to want to do it and one of the most impactful things that we did for the data culture at QuizUp was to make this metrics design a very collaborative process.
What's interesting is it's a skill that you grow, designing metrics and data structures that fulfill those metrics is a skill that you grow and experience is a really fundamental piece in it.
And so first the data team was always just designing the metrics, very commonly after the fact also, like something would get released and a question would be thrown into our lap and be like, "How did it go?"And you'd be like, "I'm sorry, did anyone track anything? How did what go?"
And trying to understand that question and the answer to it after the fact, to really shifting the mindset into like, "Before we release something we want to make sure it is to drive a specific impact. What impact should that be?"
And it was such an incredible journey to watch more and more people within the organization be passionate about pulling in the data team as advisors into that process, like, "I'm about to release this thing and I have an idea that I think I want to measure this thing. Does that makes sense? Hello, data team, can you answer this?"
Then just seeing the personal growth that everyone, from product engineers, iOS developers, Android developers, backend developers to product designers, to product managers grow from thinking about like, "Yeah, I guess we want to measure daily active users or we want to measure signups."
To having something in thought and insightful that would actually provide value.
Then even taking it a step further into when they had then seen those metrics realized, with specific event structures that they needed, they even gained insights into what is important in data structures and what do I actually literally need to track to be able to answer this question?
And it really upleveled the data quality because having a product engineer that cares about is going to be fundamental for the quality of the analytics events that you track, so I really think this is a really important flywheel.
So the reason why I wanted to bring the metrics conversation up again, it's like the org structure discussion and the ownership discussion.
I think we're seeing more and more of product teams owning this a little bit more and more and more, but it's interesting that the data teams still really serves are a really important advisor ongoing, even in the most mature organizations.
Che: Yeah, completely. One of the things I always think is interesting to observe is when you look at product teams in general, how often are they twisting their organization to match their tools instead of having tools that fit their organization?
And this can happen with data teams operating like a service model where it's like, "It turns out the only people who can use the tools are the ones who deeply understand SQL, know where all the tables are on the warehouse and can vet things upstream enough to know that the data is valid."
Well, yeah, in that world of poor infra, poor tooling then yeah, everything involving metrics has to go through the data team, and that's a tough thing because somebody's got to hire a lot of people. You're saving on infra and tools, but you're instead making up for bandwidth.
Then if you look at places like Airbnb, Facebook, et cetera, what you find is that data and metrics and experimentation are like water, it's just very easy to just build them into your process to ask quick questions, to ask deep questions.
That changes the game, where suddenly data teams become one of enablement, where they understand that analytical people exist everywhere and not all of them know SQL, and so you have to let them use their analytical brains with tools that are native to their use cases.
Stefania: Yeah, and it's become more and more a part of the job description for a product manager also to be able to understand these insights as well, and it's incredibly paralyzing for them if they are completely dependent on waiting for the inbox of the data team to clear up before they can answer a question.
Che: Yeah, completely. I think especially in the experimentation world, one of the things that happens a lot is you run this experiment and it doesn't show you what you were hoping to see.
Experimentation in general, it has something like a 30% rate of moving metrics positively, so whenever something doesn't happen the way you expect product teams have a lot of questions.
Why did it not work? Did it work for some people or did it not? And it's actually very logical to do so.
I remember at Airbnb we'd run these experiments that you'd think would be really good, but they would look negative, but if you scrutinized them they were positive everywhere except for Internet Explorer, or something like that.
Or they were positive everywhere except for East Asia because we were messing up some timezone issue, or something like that.
At Webflow, our early experiments they would be generally okay, but we would just have failed to design for Teams or some particular use case like collaboration context.
So you need to introspect experiments in this deeper way to understand how you're going to do better, but the problem is that if all those workflows are gated by data resources then basically different parts of the building are not going to get all of the answers they want.
And that could be the experiments, that could be the finance team, the marketing team or whatever, and then they are just put adrift and have to figure out some proxies or something like that.
Stefania: Yeah. So I think we've established that we agree that it's a game changer for an organization when there's collaboration on these fronts between these different stakeholders.
Let's use that opportunity to segue a little bit into org structures. I know that Eppo is still early, but you have a lot of experience with building data cultures, both at Airbnb and Webflow and other locations as well and so can you talk a little bit about org structures data-wise?
How does the data team work with product and engineering? Are they integrated with your product teams? Are they a separate team? Who owns what and those things?
Che: Yeah, completely. I have a lot of opinions about this, now having seen and dealt with a lot of different data companies.
I think one of the things that actually ends up having a lot of impact is do you bring all of the data functions under one org? And what are all the data functions?
It's the functions that are the full lifecycle, so starting from instrumentation, all the pipes, all the transformations and all the serving, and then on top of that are all the machine learning purposes as well.
So each one of those teams can only... they exist in a world that's completely data centric and they are serving other stakeholders, and reliant on other upstream people working on data. I see a lot of teams that will take the data engineering, data infra side, they'll report that through engineering, they'll have the data analytics, data science side report through marketing or product or something.
The machine learning team might even be their own pod in some way, and these teams are just much slower, I think, to get all the pieces working in harmony for reasons that are probably obvious.
Like the data infra people start doing more broad engineering infra tasks instead of focusing on the data side, the analysts have a, 'Let's make do with what we got,' sort of attitude towards things because they don't have the agency to change things upstream.
It just leads to a general balkanization that I think prevents you from doing really innovative things, so if a company is just getting started I really think that it would behoove them to bring data engineering, data infra, analytics engineering, data science, analytics and ML under one roof.
Now, you can house that roof in engineering, you can put in product, you can put into much places. But I think that being able to combine them has a lot of long term benefits.
Stefania: Yeah, this is really interesting and I think this is generally the trend that we're seeing in the industry.
And, I literally think it's not only the trend that we're seeing in the industry, it's also a journey that I think most companies go through.
This is what we did at QuizUp, and I have interviewed a bunch of people both on The Right Track and also just in the founding journey and consulting with our customers.
It goes from a single data person, that's the first thing. Then that team grows and it's a bunch of people, sometimes they get hired under different parts of the organization.
But oftentimes they start as like a single team, maybe there's a one data person that hires a few other data people and then they get maybe split up in two different organizations or different parts of the organization.
So you go from a centralized into a completely decentralized because you realize, "Okay, this person needs to work with the product team, this person needs to work with this other product team or the marketing team, so let's put them there."
This is literally my experience, most companies learn that they need to do that at some point, decentralize, and then they go back to centralizing because they learn that the individuals and those different functions aren't empowered enough to do the big stone work in the plumbing and the infrastructure and collaborate and knowledge share without being centralized.
And, in some cases, they are able to go directly into a centralized hybrid model where you have people focusing on different areas of the organization because specializing matters a lot. You need to understand the financial terms or the product terms or the marketing terms or things like that. But you have a centralized hub that can work together and collaborate.
Che: Yeah. That's generally the philosophy I agree with as well, it's have a centralized team in terms of reporting structures and careers and enablement, and then have a very high touch, white glove support model where data people live on these other teams day to day.
They are really at that PM's right hip pocket, really figuring out how can they drive outcomes in these places.
One of the things I thought, it was the secret superpower of the Airbnb data team is we were so focused on impact, and broadly defined. We purposely didn't even define it.
We were just like, "What is impact?" Well, it's like you know it when you see it, sort of thing. It was interesting because when we looked through all of the successful initiatives at Airbnb, we could say like, "What were data investments that led to really impactful product launches?"
Things where, like, "This product would not be what it is today without data." And we had some of these things, for instance, we saw that this one analyst looked through the mismatch of peoples'desired prices and the prices on the market seasonally, and proved to the org that just like everyone else in the hospitality industry, hosts need to change their price over time.
They need to charge more in the summer and charge less in the fall, or whatever, stuff like that.
You basically got to hold product champions around pricing recommendations, and so that's one of those you know it when you see it things because now there's a whole wing of the org that has realized that this problem exists and is doing stuff about it.
Other examples are, again, data shows that there are all these hosts who chronically reject people and they're just dragging down the marketplace.
They haven't accepted someone in months, but their house is still somehow popular enough to keep getting people asking, and so start out with an analysis like that and then it leads to a product proposal to demote and eventually take these people off the platform.
Again, one of the most successful product launches. It's not enough to show a powerful analysis, to show some result.
You actually have to use the rest of your holistic communication skills to lead to follow up actions, and that kind of relentless entrepreneurial, impact oriented culture of the Airbnb data team I think led to a lot of our success.
Stefania: Yeah. There are great triggers in what you just said that I want to talk about, but I want to just mention that this pricing recommendation team, that is a really inspirational data story, I think.
And so I would love to follow up with a follow up question, do you remember or know anything about how did that come about? Why was that person looking into that?
Che: Yeah. So this guy named Bart Efroc, who's a brilliant guy, really brilliant. I think he's at an Uber-- right now.
But the way it came up is that when you hire really product minded data people, so people who actually understand what is this product doing, what is Airbnb doing?
It has this pool of listings with hosts who are looking to make a little extra money or have various motivations, and you have guests who want to find appropriate listings.
You can think of the whole thing as like a big marketplace friction problem. I think he really saw that in a way that even maybe a lot of our product people did not.
He has a background in economics in marketplaces, so it was natural. If you start out with this very product sense of things then you can lay out a bunch of hypotheses for what would be most impactful.
You can say things like pricing or host's acceptance rates or supply gaps, or whatever, and just go down that list of hypotheses and see is there a 'there' there for each one?
And so in the case of Bart, he had a bunch of early successes that got him an ability to say, "I want to spend a month or two, whatever, digging into this problem." And then he really dug into it and built the case, so-
Stefania: That's a really good guy.
Che: Yeah. I think the thing you can tease out of that is hire data people, especially early on, early in the culture.
Hire data people who are really good product people because you're going to need those product chops to build and prioritize properly, and then that's going to lead to outcomes you want.
Stefania: Yeah. I remember when I was recruiting for the data team at QuizUp and people were a little bit surprised that there are so many people that have some background in data or BI or something, and I got some surprises or people were surprised internally in the organization that we weren't just filling the candidate funnel with really relevant people all the time.
But one of the things I think I learned and what I have since recommended for people when they are coming to me for advice on who was available as a data scientist for my team or who should I recruit, or how should I position the position?
And I think you really hit the nail on the head there with the entrepreneurial spirit, sometimes I really recommended one of the most important things which is so hard to identify sometimes in our recruiting process is that founder mindset.
Really hunt down alternative ways, not just answer questions, but really find ways to look at the data in an alternative way and be proactive, so just plus one on that.
Then another point about this, this person got a buy in for spending a month on this research question and that's something that most people aren't able to get buy in for, and there are multiple challenges in that.
I remember recently a tweet by TJ , who's a great Twitter account to follow. I'm currently talking about the handle T-E-E-J_M. Anyway, so he tweeted recently, I think it was him, where there can be a difference in how you can evaluate in advance the impact of spending time on data versus building and engineering.
So when you have engineering efforts, you have a deterministic amount of stuff that you want to get out there, the outcome of the stuff that you want to spend some time on building will be fairly deterministic.
You might have to slice it a little bit or change directions depending on your discoveries along the way, and then the amount that you have to put into it might be a little bit more uncertain, and definitely have to multiply it with Pi and all that.
But with research like this, both the input and the outcome can have great uncertainty in advance so you really have to make bets when you work on something like that.
Che: Yeah. You do. Another way you might think about this is data teams have a lot asked of them, how can you make sure that the org has what it needs without consuming all of the data team's bandwidth?
This gets back into some of these buy versus build decisions of are there places where you can reclaim time?
Because there's a lot of really important stuff to invest in, this sort of strategic research, strategic analyses, that's how you would hit the moon shots is when you start to realize these underlying trends.
The other things I'll highlight though is that when we say entrepreneurial people, there's a specific set of skills that I think can go under indexed in early dates in hiring.
One is product thinking, just understanding what do the customers want. Two is communication skills.
It's not like Airbnb was just naturally giving out a month or two to whoever wants to do research projects, right? They were convinced this guy could do it, and so I think there is a certain amount of communication ability around what you're doing, what you're going to deliver, how it's going to go, giving transparency into that process.
Then the last thing is people who are willing to get their hands dirty in the whole data lifecycle. I think there really is something to having the early hires be full stack data scientists where they need to both deliver impact with the final results of this research and analytics, but also mature all the upstream systems simultaneously.
Stefania: Yeah. I love these three pillars, product thinking, communication skills and willing to get your hands dirty in the full data lifecycle.
I think this is actually a really great segue because we talked a little bit about data culture-wise, we talked about org structures and how we are probably a fan here, and you're a fan of that hybrid model.
But it has to be empowered with a centralized collaboration, and personal growth reporting structure, someone who is a data scientist should empower you to grow as a data scientist probably.
But with talking about that data lifecycle, because you also talked about that being one of the most frustrating parts or difficult parts to solve at Airbnb, for example, the instrumentation part which is super, super early.
It's the top of your modern data stack, it's like, "Get that right." Can you talk a little bit about the involvement in analytics for feature releases?
Just planning it and queuing it and analyzing it and prioritizing feature work based on that data, and how that evolved through your tenure at Airbnb and how you've recommended maybe people do this today also?
Che: Yeah. Getting instrumentation right, it's a pretty tough problem obviously, as you know very, very well. I think early on there was not really much of a connection with instrumentation as linked to product success.
I think that's something where there's certainly a lived experience that has to go into it, where either you have really senior product people who know they're going to want to answer these questions and will call out that, "Hey, do we have a tracking for this sort of thing?"
Otherwise it's data teams that have to be early enough in the product process to get the stuff prioritized. I think one of the things is that it's not necessarily the case that engineers are thinking in terms of instrumentation.
I wouldn't call it a core part of their tool belt. There's a certain amount of observability in terms of the healthy of their systems, but analytics use cases, they're not so intimately connected with those.
The way I think about this is going back to what I was saying earlier, every data person knows that there is some data that's a lot more valuable than other data and early on... Understand there's an 80-20 Rule to this stuff where it's very bad if you don't know how many Airbnb bookings there are or how many sign ups or whatever there is.
It's not so bad if you don't know how many Model opens happens. By the way, you should know that, that would be great to have.
But in a world where you're starting from scratch and nothing's been instrumented and even engineers don't fully know how to instrument it because your log in system is new or whatever, just come up with some way to prioritize and get certain pieces of data really, really high quality.
Because there's such a flywheel where, okay, you've got this one data source, this telemetry locked down and now every data project can do it, with that we're able to story tell so much better around what made this product succeed or not, and that increases the desire for future products to know that level of knowledge and that leads to more instrumentation processes down the road.
Stefania: Yeah. This is really great and it's so tied with that discussion on who is the owner of the metric and how you can change that culture.
I think you're also actually touching on... I think this is a great point, when you're starting out you don't want to start with everything, right?
You want to prioritize, just like with any product strategy. I think it's super tied also to experimentation, right? I remember we created this thing that I think most mature teams eventually create and then just call it something.
We called it Purpose Meeting at QuizUp where we sat down with everyone and talked about the purpose of a specific release, how we would measure its success, and then what data sources we would need for it because you were talking about product analytics maybe isn't one of the fundamental piece of an engineer's toolbox and same goes for experimentation.
They want to release something, and it's an overhead to have two versions of your code running at the same time so ideally you want to skip it.
One of the things that I've found worked really well, and I want to throw that question over to you, what do you think works well for that, was when we had that sit down, designing those metrics and I would just ask, "Do you think if we measure this, will that tell us whether this release was a success?"
And then they would dig deeper and figure out metrics that were like, "Now maybe we need to measure this and this and this."
And then I would ask like, "Okay, cool. But what if there is some seasonality that impacts this metric so we won't be able to tie it really to that release? How would we feel about that? If this just goes up 20% as soon as we release it, will we say it's a success or could we tie it to something else?"
And then the engineers were the ones that were brought into, like, "Well, actually it probably would make sense to release this as an A/B test or an experimentation."
And having it come from them being bought into it, was the only times when we pushed out an A/B test. I just stopped pushing it out as like a forcing thing, and just like, "Do you think this will empower you as a team?"
And so it always came from them. So what are your thoughts on that? How you do drive that?
Che: Yeah, I love it, and it's a very common story.
I think one of the things that happens when you get experimentation right is that an engineer should be able to go end to end and do the process themselves.
I think there's so much of what we're getting in terms of data culture is making a statement that people should be able to be part of data culture without having to know a bunch of data infra and stuff like that, right?
You should be able to get all the benefits that engage your curiosity without having to know these systems.
When I think of the experimentation space I really think of what would be the most perfect situation for a company to be in? It would be something where engineers can easily set these things up in code, product people can easily report it up and down, align people on what matters on the story coming out of it. And data people could trust that the way they have architected the metrics is how it's going to be used and that by architecting metrics that's both the single source of truthiness of like, "This is revenue, this is the most important thing, we don't care that your experiment boosted click conversions if it didn't move the metric we care about."
And that it's going to be the correct definition of revenue, so it's revenue as we're using it in CFO dashboards or whatever so that both the hierarchy of importance and the quality of each individual metric is what data teams care about.
I think experimentation is a blessing and a curse. It's very public, it's very cross functional. Engineers, designers, data folks all collaborate it.
The perfect system would give everyone what they want, and then you say, "Okay, if this perfect system is easy to implement, it has really clean, powerful reporting, you don't have to push it into Google Slides or whatever to make it understand them all, and it's using all the things data teams want, then how can we just make that like water? Just make it really easy? And so that you set it up and you go and you get all those things?"
That's how we think of reimagining the experimentation world. It's interesting, because if you look at the experimentation landscape today and it's one that's moving quickly, I think there's broad consensus that there's a lot of opportunity here, I don't think you come across people who think of these other personas in that way.
There's some tools that really think of the engineering persona and the feature flagged use case and all the different clients and the ability to use, but they just pretend like the analytics side and the data side don't exist or it's just not native to PMs. I think to get experimentation right you really need to match what all three parties really need.
Stefania: Yeah, exactly. Again, it's so related to that prioritization so that's the other thing that I wanted to bring up.
We talked a bit before recording the episode about the statement that I don't trust this data, how come that is and why that is and how to solve it?
And I think you're touching on something there like the prioritization, there are some metrics that really need to work and then there's some that can have leeway.
But I think you have an interesting take on how to solve the data trust, so can you talk a little bit about that?
Che: Yeah, definitely. Data has a full lifecycle and has these incoming artifacts from telemetry and from transactional tables, and then you do this translation process, and then you're serving it with all these different types of results.
That means you set up this lineage tree going all the way back to the product that has to all be in concert, and like any other engineering system, this is changing quickly, it's going to break periodically.
It'll fall behind for a period of time and then have a big retrofit and get fast or whatever. This is just the nature of things.
What I think can make data culture tough is when downstream consumers who don't understand what Snowflake is or what core data tables are or what is a log event versus a transaction, they're like, "You don't understand any of these details."
They are forced to make sense of am I looking at a true result or not? When you finally serve up that bar chart or whatever, and they stare at it being like, "Okay, so am I going to hire a bunch of people based on this decision or green light a project based on this decision?"
And they have to say like, "Do I trust the insight and do I trust the data?"
Tools like ourselves, Eppo, and experimentation because experimentation is actually an extreme case of obfuscating the early parts of the data lifecycle, we have to deliver people that knowledge.
It's like, "Is the data good?" Before you actually dig into insights, is the data up to date? And so we create these little data quality scorecards in Eppo that basically say, "Here's the state of your data. It should look clean, it should be up to date, it should match what you see in your other data systems."
You roughly know you get a million purchases a day or whatever, you're going to look at the chart, looks like that sort of thing.
So basically downstream consumers need to build it quickly, cross it off their list that this is not a data quality issue, and thus I can proceed to insights.
Now, if the data quality thing is an issue they at least know. It's like, "Okay, I don't need to. I can go bug my data person about it, I can invest in those systems, I can do these other things."
But where you run into trouble as a data consumption layer is if you don't let people quickly do that fork in the road of like, "Is this data up to date, good, high quality, et cetera, versus not?"
Stefania: Yeah. I think this is such a strong point and I recently relistened to my interview with Moira Church at Patreon because writing a blog post from...
She had such good tactical and strategic advice that I want to summarize, and she had some thoughts on this, and as an additional point I would love what tactics can you share to make sure this is properly documented or that downstream consumers know what they can trust and what they can't?
Che: Yeah. There's a few ways of going about it, and this is something I actually think there aren't established best practices around.
But one of the things that you might see is companies like Airbnb will have a data portal, some sort of data catalog or some system like that, that basically is a one stop shop for you to just go and get these sort of quality scorecards and just say, "Okay, I'm looking at revenue. What is the state of the revenue data?"
And so no matter what other system you're in, whether you're looking at an experimentation result or a BI dashboard or whatever, you can always just go to that spot and get the data quality check.
But it's tough, because then the downstream consumer also has to know that this data catalog thing exists and that the URL is this and here's how you navigate it, and suddenly you have to absorb a whole new tool just to do these basic checks.
Also it doesn't extend into things like a presentation, where you don't have the ability to go to these other places.
Stefania: Or an ad hoc spreadsheet that someone put together.
Che: Well, exactly. So the way I think of these systems, this again gets back into the buy versus build thing.
But because we are a SaaS product that has frontend developers and like a full suite of capabilities, we can actually make a native experience of data quality checks.
I actually think a lot of other consumption layers, whether it's your BI tools or your marketing analysis, your churn modeling, whatever, it behooves them to have a similar thing.
There's almost a ritual to it, if you're a PM you just want to check these things and prove to yourself that the data is good, instead of having to trust that someone cares about your work as much as you care about your work.
Stefania: Yeah. I loved a couple of things that Moira shared. One of them is just religiously label things as Here Be Dragons, like, "If you're going in here, you are potentially entering into an uncharted territory here."
Then the other thing that they did is they literally have pizza and beer or soda night where they just go through all sorts of Google Drives or Dropbox paper folders and just clean out stuff. "This is an old presentation." Or, "This is an old chart or old sheet.
Che: Yeah, totally get it. This is another example of what I would call trying to fit your organization to your tools, instead of your tools to your organization.
It's, we're going to have a pizza night at council, experiment council review, whatever. We're going to just acknowledge that there's a finite set of people who can actually do this vetting and they need to become available on some broad, open public.
So I get it, you got to solve this in some way or another and we did our share of those things too. But in a world where the tools are getting much better very quickly, I think you shouldn't need specialized councils to do this stuff as much.
Stefania: Yeah. I think that's a really good point.
And then it's a matter of the lifecycle you're in as a company, or a maturity stage as a company and as a data team.
I think there are tools or processes that you can apply while you're a little bit early and it doesn't make sense for you to invest in building a lot of automation around all of that stuff and then you take that as input into your down the line strategy.
Che: Yeah, exactly. The thing I will mention is that you still need things like these office hours or whatever, just because suppose you have my perfect world and you can introspect data quality at any moment.
You still need to know who to bug if something's wrong, right? So as companies grow, as they get more remote, even just having the names of the data team be more visible and more accessible, it's a nice catchall process.
But I think what I'm getting at is that it should be really thought of as this general catchall thing and it shouldn't be a core... You should be able to do good work without having to attend office hours.
Stefania: Yeah, exactly. Awesome. So I want to start wrapping up here, this has already been such a fantastic conversation with great takeaways both strategic and tactical, so thank you so much for sharing.
But taking a step back on some data misconceptions and what teams should do to get their data right, so a couple of questions on that note and starting with this one, what are people's biggest misconceptions about how data and product analytics work?
Che: The misconceptions for non data stakeholders, I think, is that honestly it's very easy to get good data.
I think if you are a PM, you probably do not know the full lifecycle of what this data point went through.
It first had to be instrumented here, and even instrumenting properly, it's a small amount of low impact code but you actually have to deeply understand the system to do it well.
So there's that, and all the downstream processes and just the full magnitude of all of the data pipelines that are happening, so I think for downstream users they are starting to appreciate a little bit more of just the amount of data investment you have to make to get this outcome you want.
It's not like a snap your fingers and it's good thing. For data teams themselves, I think the biggest misconception is that the point is better decisions, the point is impact.
It's obvious when you say that out loud, but you don't start a data team to have a data warehouse.
You don't start a data team to have BI tools and to have these data modeled and all this stuff. The whole point of this thing is that the org makes better decisions, so if you are a data team you need to care.
The end to end of a data lifecycle doesn't end with a bar chart, it ends with good decisions being made as a result.
That gets at a little bit what I meant with the Airbnb thing in terms of our culture, it's that we actually saw the world that way.
It wasn't enough that you did this nicely packaged piece of data work, you've got to drive the org in this really important way.
So I would encourage all data teams to think about how are you improving decision quality at your organization?
Stefania: Yeah. I think that's a really good framing.
You mentioned it in passing but one of the things that we did want to cover is literally in this specific interview, it's how do you define data culture? And I think you've now done it.
Che: Yeah, exactly. Decision quality, that's the term I find myself using over and over, and it's also obviously the worldview that leads me to run an experimentation business because it's very, very linked to decision quality.
Stefania: Yeah. And moving off to a starting off question, what's the first thing teams should do to get their analytics right? Because we've talked a lot about experimentation-
Che: Yeah, yeah. I think like a true CEO, I think my answer is hire the right data leader. Again, you need someone.
To be able to get the data file going, you need to get some set of data work that does this end to end thing.
It has all the inputs you need and then it leads to impact on the other side, that impact is something that has a You Know It When You See It quality, where the CEO, the PMs all know that data was really important here.
Now, the thing is to do that you're not going to start off with this fully built out infra so you're going to have to pick your battles.
There's like a strategic sequencing to get there, and that's where I think bringing on the right person who understands how to operate at every level of the stack, who can communicate and evangelize properly and just build a great team. I think that's probably the most important step.
Stefania: Yeah. And so what you mentioned there as like, okay, the first step if I am someone that I am trying to build a data team and it's non existent, then I want to hire the right data leader.
Then in the second part of your answer, you also gave recommendation to that first data person or first couple of data people.
It's get that data flywheel going and pick your battles and build a sequence of things that ultimately are about decision quality.
Che: Yeah, get that flywheel going. I think one thing tactic I end up using all the time, see, if you're a data leader what I highly recommend is mapping out the user journey.
And so all the milestones someone has to hit to get value from your product. So if you think of Airbnb, first you have to understand, you have to be aware that Airbnb exists as a concept, then you have to understand what does Airbnb do, when should I be using Airbnb, the market positioning thing or whatever.
Third is you have to have that use case in mind, so I may want to book a trip, I'm ready, I want to go to the Swiss Alps in the winter or whatever.
Then you have to go and find a listing or a situation that meets your needs, you have to book that listing and then go on a trip.
So if you just stage it out like this, first it brings you into the language of the CEO because every other business person in there who's properly customer obsessed can understand here is all the stages.
Then the natural thing that starts to happen is to say, "Okay, if we look at all these stages, what is our state of data in each one? Couldn't we say what is the level of market awareness? Can we say how many people find listings that match their needs and then how many end up booking?"
That will naturally lead to conversations that are like, "Okay, let's upgrade all of our input data to those things."
And along the way as you eye those stages of the funnel, just if you say like, "If I was to improve the booking conversion thing, I could try to build a search ranking model, I could try to understand these marketplace frictions, whatever. If I was trying to increase the satisfaction of the booking and the 5 star review rate, here's some other levers I could pull."
Then you just gotta prioritize them, right? You've got to say what's most reasonable given the state of data, given the state of teams, given the people I'd work with, like does the team running that have someone who understands how data works?
There's a constellation of factors that go into it, but I would just start there. The user journey, what does that tell us about the state of data and where data messes can be made?
Stefania: This is incredible, I think this is really... it's something you can apply pretty early so I'm hoping this will be helpful for a lot of people.
So we've covered a lot of area, and you've answered already maybe my last question so we can maybe make that a quick one and maybe just switch it over to what's next for you, personally or on the Eppo journey?
Is there anything you want to add? What's one thing you wish more people knew about data and product? I think you've already answered it though.
Che: Yeah, I think I answered that one. But I'll say in terms of for me, obviously I'm going to be building Eppo for the next indefinite future.
We're trying to reimagine the world of experimentation and think about this intersection of technology problems and cultural-organizational problems.
I always say we are really in the business of trying to sell entrepreneurial culture, we're really trying to sell this world where anyone in the building can have an idea and validate the idea against metrics really seamlessly.
So we just started deploying all across the tech industry and hoping to keep growing from there. I think this overall goal of improving organization decision quality is really what we think about.
How can we just enable better decisions across the whole company?
Stefania: What would you say is the right time for a data team or a product organization to start thinking about this?
Che: I really think it's once you have enough sample size to do things with experimentation with data.
And there's a trade off here because even with a small sample size, maybe you can detect a 30% increase in your metric or something.
But if you can detect something like a 5-10%, I think you've already reached the threshold where experimentation is going to yield a lot of benefits to the org, both in terms of business ROI and in terms of culture.
So that's what I would generally say, is you bring on a data person, that data person should have the basics of the modern data stack, Snowflake, DBT, whatever. And then just see if you have a sample size to run an experiment.
Stefania: Exciting. Where do people go to try out Eppo?
Che: Yeah, you can visit our website at www.getEppo.com. Again, that's www.getEppo.com.
Yeah, our contact information is up on there, a lot more details about how we see the world. We also have a Substack which is Eppo.substack.com.
We're putting a lot of thoughts similar to this interview in there in terms of what do data teams and product teams all need to realize to get benefit from their experimentation programs.
Stefania: Nice. And I know that you have historically written really good content. Is that also where they find all of that?
Che: Yes.
Stefania: Perfect. Eppo.substack.com. There is already good content on there, I can verify.
Che: Thank you. Thank you very much.
Stefania: Awesome. So thank you so much for taking the time to join this conversation, Che.
It was a pleasure having you on and I look forward to part two when we can dive into the future of Eppo.
Che: Absolutely. No, this has been fun, Stef.
Stefania: Thank you. See ya!
Subscribe to Heavybit Updates
You don’t have to build on your own. We help you stay ahead with the hottest resources, latest product updates, and top job opportunities from the community. Don’t miss out—subscribe now.
Content from the Library
The Right Track Ep. #12, Building Relationships in Data with Emilie Schario of Amplify Partners
In episode 12 of The Right Track, Stefania Olafsdottir speaks with Emilie Schario of Amplify Partners. Together they discuss...
The Right Track Ep. #10, Getting to Know Your Users with Boris Jabes of Census
In episode 10 of The Right Track, Stef speaks with Boris Jabes, CEO and Co-Founder of Census. They discuss the impact of SaaS on...
The Right Track Ep. #8, Defining the Data Scientist with Josh Wills of WeaveGrid
In episode 8 of The Right Track, Stef speaks with Josh Wills of WeaveGrid. They address common misconceptions about data and...