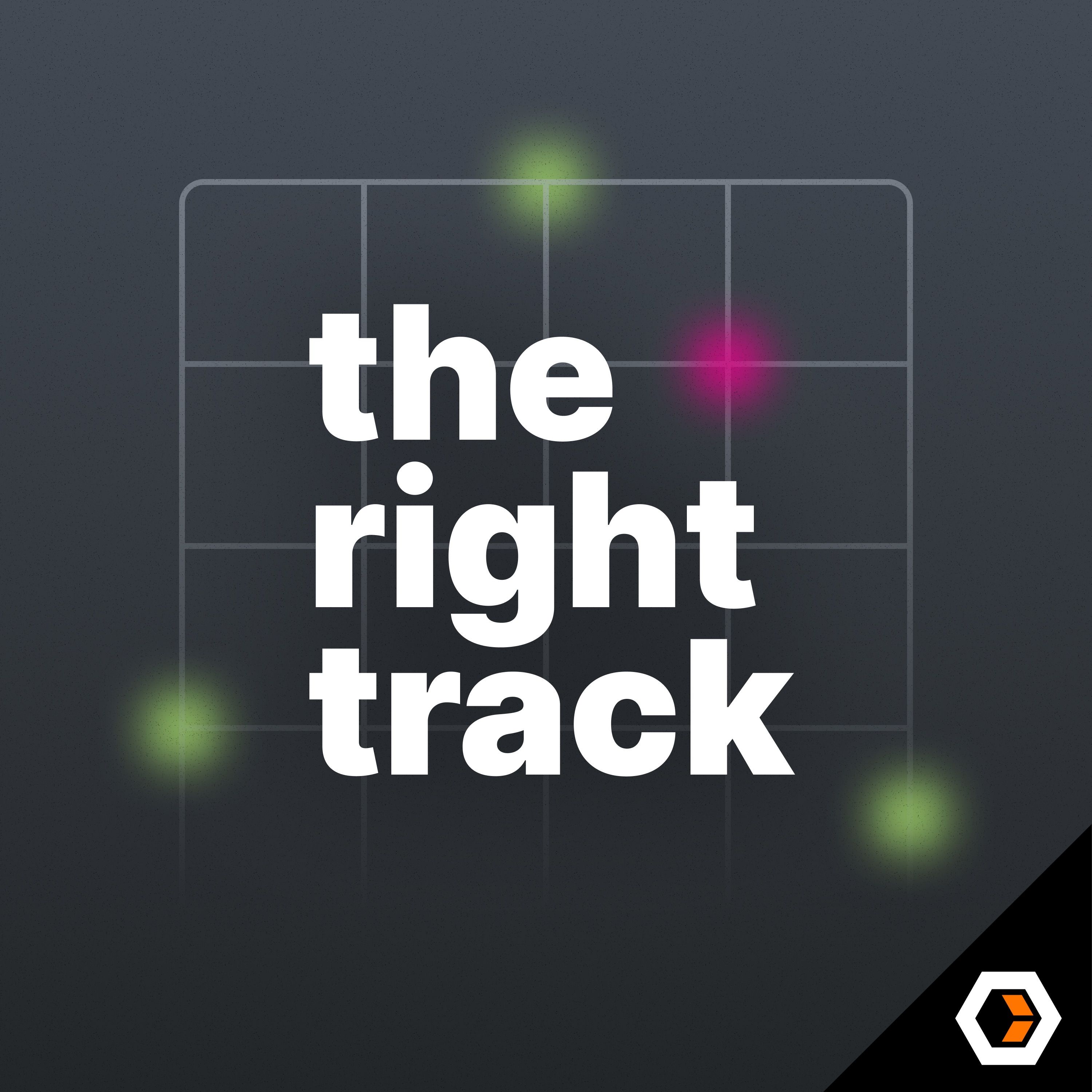
Ep. #3, Data Custodianship with Nick Threapleton of Culture Amp
In episode 3 of The Right Track, Stef is joined by Nick Threapleton of Culture Amp. They discuss tactics for building data cultures, Nick’s journey from marketing analytics to product analytics, and empowering teams to use data in their work.
Nick Threapleton is a Senior Product Analyst at Culture Amp, building their data culture and product intuition with self-service tooling, education, and deep analysis.
In episode 3 of The Right Track, Stef is joined by Nick Threapleton of Culture Amp. They discuss tactics for building data cultures, Nick’s journey from marketing analytics to product analytics, and empowering teams to use data in their work.
transcript
Stefania Olafsdottir: Hi Nick, welcome to The Right Track.
Nick Threapleton: Hey Stef, thanks for having me.
Stef: Super excited to have you.
Could you tell us a little bit about yourself, who you are, what you do, and how you got there?
Nick: Yeah sure.
I'm a senior product analyst at Culture Amp, although sometimes my job title is product manager of analytics, it's a little bit gray at the moment.
My background actually initially was in industrial design.
So I studied, for those that don't know, it's very much like product design, pretty much in the world of architecture, because I really just liked solving problems.
And then I realized that there's just no industry for it at all in Australia, sadly.
And I got really interested in UX and I was doing a bit of UX through like advertising and an advertising agency.
And I kind of landed in the agency world and ended up doing digital marketing 'cause that is most of what you do in the agency world.
And that's where I learned that at the end of the day, digital marketing, like good digital marketing, is really good analytics.
It's one of the most important skills of being a good digital marketer.
So there was a problem for me to solve and I was really interested in solving that.
So I really focused on that and build my skills in analytics in that world, which I think is a pretty common path for people moving into like a product analytics, digital analytics kind of role.
So I was doing that and I realized I really wanted to work in product.
So that's when I made the move to Culture Amp and I've been here for about two and a half years now and have been working my way through more and more around building a data culture and thinking about how we do analytics, not just ourselves, but getting our product managers and our product teams to do analytics themselves to make changes and make a better product.
Stef: I love that story. I actually didn't know that you came from industrial design, you said that, right?
Nick: Yep, that's right, yep.
Stef: Yeah, wow, I was actually close to studying that myself.
Nick: Really, I just build furniture now on the side, that's fun.
Stef: Wow, that's amazing. So, I mean, okay, side story.
I registered almost for this education, but instead I went and traveled in Australia for a half a year.
Nick: Yeah, it's a way better decisions.
Stef: Yeah, like Melbourne has always been one of my favorite cities.
Nick: Really?
Stef: Yeah, definitely.
But yeah, I think you touched on something that's super also interesting, just before we dive into all of her data culture stuff, which is the journey from marketing analytics to product analytics and your theory on, "okay that's a common journey."
I'd like to touch on that maybe a little bit deeper because I think that's right.
Do you have like a thought on why that is?
Nick: Yeah, I think so. I think digital marketing pushes you into kind of the web app space.
So really to do digital marketing well, you have to understand at least the basics of tracking.
I mean you have to understand how data is collected and how did this processed, so that you can use it in the way that you market to people. You have to think about how segmentation is run or the quality of the data to understand who you're talking to and how you're talking to them. How accurate is that audience that you're going to end up talking to and how accurate is your tracking?
So you end up learning, I think a lot of skills around a bit of front-end, a bit of tracking, and just kind of data literacy skills in general.
And I think 'cause that is really the skillset of a product analyst.
It is a combination of what a more traditional business analytics skills like sequel and things like that.
And then having that kind of front-end, slightly more technical literacy around the web or app.
Stef: Yeah, I love that.
I'm wondering also whether it is a little bit about like this journey, I mean it fits a little bit into the AARRR, the metric world, I would forget how many A's there are and how many R's there are.
But it's like you're, we're talking about the acquisition, pardon, getting the right people through the door and then getting them into your product.
And then after that, obviously, what you want them to do is be successful.
So it seems like a natural progression, number one.
Nick: Yep.
Stef: And then the other part, which is interesting is just like this, how the world has shifted in how they think about growth.
It used to be about acquisition, but really now it is way more about like how do you build so good products that have so good value that people want to stay and keep using them for a long time?
Nick: Yeah, it's maybe not all about retention but it's largely about retention.
Stef: Yeah, exactly. I would love to ask you if you can tell us an inspiring data story and a frustrating data story.
Nick: I'm going to start with a frustrating one because I think it's quite serendipitous to this recording.
About a few minutes before we kicked off this recording, we picked up that one of the most critical events that we track in the app was significantly under tracked.
So we realized actually only about 9% of the events that happened were actually tracked.
And it's one of the events that goes towards our North star metric, which is weekly active managers.
That means that our North star metric we've been reporting on for the last year could be 50% of its actual value that it should be reporting on which is pretty dramatic.
Stef: What was the discovery? How did you discover it?
Maybe you don't know that yet, we've just found out.
Nick: Yeah, it's--
No, we actually discovered it because we tried to switch our old active users definition to our new one, which is uses Amplitude.
And we realized the user count was way, way less.
We looked at it and found that the major discrepancy was this one event, which is when someone submits a survey, we're capturing their separate responses, which is a very critical thing to a company that does surveys.
And we think it's just to do with the way it's implemented.
I think it's just, it's firing before they're redirected and sometimes redirect happens before the firings.
It's a bit of a race condition problem.
Stef: Hey, my favorite kind.
Nick: The best kind.
Stef: The best kind.
Nick: I think it's an interesting problem though because it really touches the whole chain of responsibility.
In a decentralized world, we have a whole company along on a metric that relies on all of our teams to contribute.
So I think it's going to raise some insane questions about how we can maintain data quality and data custodianship so that people understand the teams, not just how they use it, but how the rest of the company will use it as well.
So I think that's going to be an interesting day for me.
Stef: Oh my God yeah, I almost want to do like a part two of this, conversation.
Nick: Go for it.
Stef: How did it go?
Nick: Stay tuned for part two?
Stef: Yeah, exactly.
So I think you're touching on something really exciting though, which is learning the hard way about how much of an impact you can have, on the entire sort of business trajectory and decision-making by skipping a single.
Nick: Look it's not ideal but in some ways it is a positive thing.
And the reason it's a positive thing is I think you do need to go through these things to see the real impact.
Like it's a bit ambiguous the value of data or the value of getting things right, until the impact happens and people realize all like, this critical thing we make decisions across the whole company is now not working 'cause we didn't get this right.
So I think it's a really good way to actually demonstrate the value of doing these things correctly.
So I'm not, that's not great, I think there's an upside to it too.
Stef: Yeah, I just, I you're right, it's serendipitous.
I have two follow up questions that are, one of them is probably a good segue into the rest of the conversation.
The other one is sort of more of a comment.
I love that you're talking about data custodianship or how did you frame that?
Nick: Yeah data custodianship.
Stef: Is data custodianship something that you, did you make that up?
Nick: No I stole it from one of our backend engineers, 'cause it's a problem in backend engineering and it's a problem in analytics.
It's the idea of responsibility of data and people understanding where those lines fall, which is really critical, especially in a company that's kind of heavily matrix store decentralized, which I think a lot of product companies are.
And the way we look at custodianship is more of an outcome of everything else, that is like if we get all of our data culture things right, we get people that are interested in using analytics, the data custodianship becomes easier. You know, that people understand the responsibility, to understand the benefits to owning that data.
So it's a hard one to drive on its own I think, it really is something that you measure but you try and change through more indirect ways.
Stef: Yeah, I love, thank you for sharing this.
I love this framing because people have been talking about data governance a lot and I personally come from a data background and it was a framing of a part of my job that I just hated.
I was like no. Number one, I have no interest in governing this, we should all be incentivized for this to work, and number two, I was just very allergic to the word.
So I think this is a word that I could have always related to, so I really like that.
And what you just said, I feel like you're touching on this journey that companies go through, which is from the centralized BI team to the self-serve analytics culture, to realizing that self-serve analytics culture doesn't work if you don't have quality data.
And then you get to this like centralized governance, and then the ultimate mission will be like decentralized data custodianship type of thing, maybe.
It's like a beautiful utopian world, so you can build it.
Nick: So that is the utopia and it's a very hard place to get to I think, but worth it.
Stef: Yeah, yeah, and this conversation today will be about, I guess your journey to get there though and the learnings along the way, which I'm excited to cover, which brings me to my other question, which I think will be a good sequel into like the cultural aspect of the company.
Who will care about this thing today?
Like who will be a person that will be bummed out that this happened?
Nick: Are you talking about the problem with the North star?
Stef: That's right.
Nick: Yeah. Look, our board who we report to, our exec and all the people in private that make decisions about it.
We just focus if there's a big analysis on decomposing that way I'm into some input metrics and understanding where the drop-off is and driving that north star and some of the opportunities to fix it.
We're going to have to look back then, I mean that that all that work as well might be wrong.
So that also means us do we care about that, because it really does affect the insights and the actions we've decided on based on the problem with that data.
It really does go across flips the whole business.
Stef: Yeah. We'll probably touch on this later, but I feel like there's a maturity stage in the company life cycle of where front end developers even, that historically have seen analytics as tasks, they start caring about this also, that's a maturity stage in my opinion.
Nick: I totally agree and I think that's a big part of data custodianship, is that moving that from that understanding of like, analytics is something that I add when I have time as a nice bonus to help out, to being like, I know analytics is really important to me and the company and it there's a benefit to me doing it and people want to do it.
That's a hard place to get to but I think it's a good goal.
Stef: Awesome. Thank you for sharing that frustrating and timely story.
Did you have an inspiring one as an opposite?
Nick: Yeah, I do actually.
So this is a little bit longer maybe a year ago, but I think it's one of my favorite examples of using product insights, and pretty simple ones, honestly, to make an impact.
So we'd been struggling for quite a while as a product analyst team in terms of just questioning, like are we really making impact?
Like, yeah we're answering these questions, people want to know how people use my thing and where we're all centralized, so we're just answering it for them.
And, you know it was very hard to get a sense of like is this important or worthwhile?
What's the point of our jobs? And then this question came through, I think to explain this question, I'll just give a little bit of background and culture.
And for people that don't know, one of the major parts of product is engagement.
So we help companies survey their employees to understand how engaged they are.
And then the really, the big value moment for this companies is getting the report back, because that report gives you a lot information about the drivers engagement and compares it to other similar companies and things like that.
So that's a really critical point. Some companies choose to follow, we have a thing called an action framework, where people can choose to take actions based on those focus areas.
And then the last thing they can do is ask their employees how they went taking that action.
So it's like an action feedback survey kind of thing.
And one of the product managers of that area was like, "Oh no one is using this thing, like why is the use of it so little, like we need some insights on how we can improve this sort of thing."
So we started looking at it and we're like, "Yeah, you are right. There's, you know, it's not significant, the usage."
And they're like, "Yeah, can we dig into it? Like what kind of customers are using it? Can we like find more of those people? Can we expand that way?"
And we started looking at it and I'm like, "Hang on, is this really the right area to be focusing on?"
So what we did is we just built a really simple funnel which we looked at from the very start of launching a survey, through to reporting, sharing the report, coming into action and then doing the follow-up survey.
We realized really clearly that the problem is not at the bottom of the funnel, the problem is way further up.
And there's a way bigger opportunity to affect way more customers and provide way more impact as well as improve this thing, and that was to get people to share reports.
So we realized that most companies weren't sharing reports with most people.
So a majority of our companies were just sharing reports with HR and maybe exec, and they weren't sharing it out to like new managers, and ideally we try and coach companies to show it to everyone, filter down to the relevant teams and things like that.
So that this huge, huge drop off in the funnel from launching a survey to sharing a report.
So, the chance of someone's going to make it through to then, get four steps down this funnel is very unlikely.
And when we realized that that actually sparked a big change in the whole entire group, that deals with engagement, and actually shifted their goals is highly over the next year nearly, to focus on the quality report sharing, the accessibility reports and all that kind of work.
And that's actually made a big impact.
Stef: I love that.
Nick: Which pretty incredible.
And it was such a simple piece of analytics.
It's literally a user journey funnel.
Stef: I agree that this is a really inspiring story.
And this is like a story where, it's wonderful that the data was in place or that you could implement it fairly quickly to set up like this analysis.
But stories like these, they are the stories that trigger people to want more.
They are the internal marketing of the value of data and analytics that sort of spark us to like, ah, okay, I might want to do more of this.
So I think these are like doing huge promotional internal events for learnings like this can be super valuable for building good data cultures in my opinion. Congrats on this.
Nick: Yeah, it was, it felt good.
Stef: I think this might be a good segue into like, I don't trust this data is a very common statement.
We've talked about that before you and I, and I know you have some heartaches under that conversation, but I think it will be fun to share.
And inspiring stories like these, they are trigger for people wanting more about data.
And so this statement, I don't trust this data, it's super common, why is it? And how can you solve it?
Nick: It's a really good point.
That is a challenge that I think everyone that has a self-service culture has dealt with before and probably deals with really often.
And it's a problem that we've in the past addressed as a technical problem, which is not necessarily wrong.
You know, we look at making sure that there's good QA and things like that, but I think it misses a larger part of the problem and that is a larger data cultural problem.
Which is that forget data quality, you need people doing analytics, because if they're doing analytics and they care about checking good quality data.
But to get people doing analytics, you need good data quality.
So it's this kind of, it's similar to like the marketplace problem where you're trying to get both at the same time, and it's a really, really hard one to solve.
Like how do you build this like cycle, where you get data quality and analytics and one doesn't break the other.
You know you might people start using it but then the data quality is really poor and then all of a sudden people stop using it because they don't trust that data.
That is a hard problem to solve, it's not one that you, I think you can solve right away, and I think it's only you do need to solve iteratively.
The one thing I think that we did get right and that we're still focusing on is building this like a minimum level of quality.
So you make it as easy as accessible as possible for people to get data right. It does require a bit of work and a bit of handholding, and it's about like having the right tooling, making it really easy to see what events are coming through, and making people really see literally how the data comes through as well, so not making it too abstract.
I found like really helpful people can just see the events as they come up, when they go through this journey.
It makes a lot of sense to them, they can go into amplitude or wherever they're doing their analysis and then understand that, Oh, okay like I'm doing these things, that's the journey, that's how the data comes up and so I analyze it and that's what I'm going to get for everyone else.
So I think that's where you start to get a bit more trust in the data is getting that minimum level of quality, but you need to also consider that problem as well, that problem with people actually using analytics to get data quality in the first place.
Stef: Yeah, I just love this comparison to the marketplace and finding a product market fit with product analytics with in the company.
I think you've really hit the nail on the head there.
And I think what you're highlighting is like, well there are trust issues.
They are not only technical issues, they are very much around data literacy, which is one of the things that you touched on.
Like for me to actually understand what is the thing that I'm looking at.
And it sounds like, exactly like what does an event even mean when I press a button and when it count stuff?
Having that transparent is one of those things, I relate to that.
Yeah, so there's data literacy, there is data inspiration, and then there's the data quality as well, piece.
So yeah, I think that's a really, I love this, I love this analogy.
Nick: The more I do this, the more I realize when you're trying to do self service, it has a lot of parallels to just regular startup problems.
And you mentioned product market fit and I really do think a data culture is having a really good product market fit.
And when I say market, I mean your internal stakeholders, between the self-service tool and the way that you sell it and build it and the culture.
At the end of the day, I think all of these things, data literacy, data quality, it doesn't matter how good your tooling is, you could really have the world's best analytics self service tooling, but if you haven't sold it in the right way and you don't have the culture around using it, it's just isn't going to work.
People aren't going to care about it, they're not going to understand the value, so they're not going to use it.
And if they don't use it, you're not going to track the data and get the data quality that you wanted in the first place.
Which is frustrating but it's also kind of inspiring, like it's an interesting and fun challenge.
Stef: You've touched on this thing which is an obsession of mine, a personal obsession of mine, which is bringing data consumers and data producers closer together.
And by data consumer, I mean people like product analyst, product managers, data scientists, whoever is playing around with the data, eventually.
And then the developers that actually write the code that get the data points to whatever you need to use the data in, but also the data designers.
And so having those people in group communicate really well together, I relate to that.
And particularly when the data producers become the data consumers, that when developers actually care about analytics and point and click and charts and amplitude or whatever, or Mixpanel, or Looker, or wherever they're looking at their data, I think that does a lot to, I agree.
You did mention also, I guess it's another way of framing it, but I love that you mentioned it, it's, you once told me that you have commonly heard verbatim I don't trust this data, but you've also heard sort of, I don't trust myself to pull this data.
Which I guess is around this data literacy thing.
But I wanted to talk a little bit about what you're doing to fix this, because I know it's been an adventurous and inspiring, and sort of rapidly changing culture at Culture Amp.
Nick: Yeah, culture change of the culture company is interesting.
Stef: Yeah, yeah. And so can you tell us a little bit about this journey?
Like what needs to happen to find that product market fit?
What has already happened and what are you doing more around that?
Nick: So I think there's some tactical things that we're doing and then there's some motivational things that we're doing as well.
So I'll talk to the tactical first, and those are a bit more obvious and literal.
So that's focusing on things like data literacy.
And so we're looking at do we buy in or do we write some kind of course to just scale up all our dated consumers and ideally data producers on data literacy.
So they have that confidence in themselves to know that when they're pulling data you know, they understand correlation and causation. They understand some of the common pitfalls outliers in data that are pretty easy to fall into. And they have that confidence that when they're pulling it, they're probably pretty accurate, they're getting pretty confident with things.
But like I came back to before we could offer these courses and I think if we put it out today, we could buy the best course and the best university in the world and make it super interesting and really fun.
And our PMs will do it and they will really like it, but I don't think that they'll apply it right away, and that's not a fault of their own or anything, it's actually a challenge in our data culture.
It's like, we need to fix this problem of incentives, like we need to build this world where our product teams care about data enough, that there's a pool for this stuff rather than a push.
There's always going to be a little bit of a push, you always going to push some things out, you know, enforce some things.
But I think if you go too far in the push end, it's like, well you got to executive, can you enforce things and you say everything has to be done this way.
And I think I'd like to borrow a term for economics, it's basically rent seeking behavior.
Like it just, no one likes to be forced to do something and I think that's why it's really critical to build a culture where people are the incentives and the interest in pulling data in the first place.
And then the things like focusing data literacy, friction data quality become more important.
Stef: Yeah, it's like this, it's the top down sales versus bottom off.
It's just easier to sell stuff when there's already buy-in and interest in sort of the smaller stuff and you've seen adoption.
I remember you also mentioned or I guess like maybe a up question of this, what does that look like then in practice?
What are, how do you create that sense of time? Have you been doing it so far and what's coming up?
Nick: Yeah, that's honestly the biggest question we have right now, that what we're trying to work through as a team and that's our focus for this year, is how do we really embed the incentives and the data culture into the company?
And there's a few things that are happening, I don't have too much to report on their success so far, but I can talk about how we're doing those things.
And the first one is we are starting a little bit at the top, and by that I mean, we have a north star metric, but most teams have no idea how they can contribute to that because it's so disconnected from what you do day to day.
So we've done some work to decompose that North star into a funnel, and then a series of metrics that drive that funnel.
To give a practical example that it's effective managers. Well, the first step is you need to, you start with contracted users, you need to identify if they're imagined the first step.
And then once you know their manager, you need to get them into the platform for the first time, then you need to get them back in some more regular periods and then need to retain them.
So, all right, there's some steps that we can focus on and the job of each of those steps make more sense.
That some of them are a sales and customer success at the top, and some of them are product and protocol lead down the bottom.
And then each of those metrics can be broken down further as well.
And I think as we do that, we're going to build metrics that are both very relevant to the company strategy and actionable at different levels.
So some are good for kind of department or what we call camp levels, or like product group levels.
And some are, you know as we get further down, more appropriate for teams.
But that's still a lot of metrics, like that is a lot of things for a lot of people keep track of.
And one thing I'm really fond of that our product execution group is doing is this idea of themes.
So as a way to simplify thinking about this, is like grouping everything into four themes and an example of a theme is bringing imagined in the platform.
So a theme has a set of objectives and people attach their kind of, okay as to those themes.
So we can track this theme with a key metric, like manage the platform and manage retention.
And then teams can work out how they contribute to that theme.
So they can pick a metric that links to it, or if they have a hypothesis that they want to test, they can also do that and see if the impacts that outcome measure.
So I think the benefit of all the doing all this work and thinking about these things is that teams have a goal that can be measured that they care about.
Because right now if the person above a team doesn't care about the measures that they're working on, then they're not going to about it, the incentive isn't there.
And so analytics becomes a nice to have, because you're doing it but you're not measured on the outcome.
And I'm not advocating for this like extreme world of everything has to be delivered on a specific measurable outcome.
But I think a step towards that direction, having outcomes that indicate success is really handy for teams, is really important for building a data culture, because everyone cares about the metric.
Then if you care about the metric all the data has to be tracked really well and you have to make sure that the quality is there and you have to make sure that you understand the data well enough to report on it.
So I think that that's probably the key way we're going to work on building that pool, is actually through these themes and these metrics.
Stef: I am so excited. I'm your behalf, I love that.
There's just going to be fun, yeah.
And just kudos on a really exciting execution on this strategy to the product execution team. It sounds like.
Nick: Yep.
Stef: And I agree with you, this is the step towards like, you framed it just so beautifully.
When teams have goals, they actually care about, analytics becomes something they care about and it doesn't stay as one of the tasks they have to complete.
It's just as unfortunately our mindset that is still there in some cases and makes sense as a mindset.
If analytics and the value of the data that you're getting from it is so far removed from you.
Nick: Yeah, it's really, it does come down to organizational structure and incentives actually.
You know, even if the individual is really motivated which you have some PMs love analytics and do an incredible job of pulling their own data.
It's extra work you know, it's not a part of their day-to-day and we want to make sure that it's a part of the skillset and the things that they do, because it is important for building a better product at the end of the day.
Stef: Exactly. So I want to maybe segue this a little bit into sort of your org structure.
I know that you are personally sort of running some initiatives and adding to the team and putting more focus on analytics and product analytics and things like that.
This is something that I think I get this question a lot when people reach out for advice on who should they hire and who should that person report to, and should the analytics data specialists or data scientists or data professionals or what we want we call them, should they be in their own team or integrated into the other teams?
How does data work with product and engineering?
I'm dumping a bunch of questions in one as sort of like, can you talk a little bit about your org structure and how that has evolved, what you've learned along that route and what you aspire till there?
Nick: Yeah. I'll start with our structure when I started about two and a half years ago.
And that was a really traditionally centralized analytics team.
So the team was centralized, there was three of us, one person that you'd probably call analytics engineer today but it was called product analysts back then, you know team lead and myself product analyst.
And our infrastructure was centralized too, so we'd pull the data in, we were the gatekeepers to the data.
If someone asks a question, we'd choose to answer it or not and we give them an answer.
And some people like that because it's like, it's easy.
You can like send a thing off and you get something back sometimes, it's like.
Stef: Hopefully.
Nick: Yeah, hopefully, and you know there's a bunch of back and forth and it might take two weeks before you get an answer to a question, and you probably already shipped the thing anyway because time doesn't stop for analytics.
So, you know, that was like, that was going okay until when we really noticed this is the problem, was we run a test with one of the product managers.
They added a new little banner to one of our main kind of top level pages and it was basically a called to action banner to use a new feature.
And what we did is we said that's about 35 people I think in kind of the larger product group that looked up with product.
We sent a survey to all of them, like what percent of people over the next month that go into the platform, will click this thing at least once?
So you know that when everyone from like engineers, data scientists, product managers, QA agents, everyone in the camp.
And the average guests, like the average like middle guests was about, I think it was 48%.
So, on average people guessed that 48% of people would click this banner, and like to anyone that probably listened to podcasts, watching analytics, so ever looked at, you know kind of web analytics, you know that that is extremely optimistic.
And the actual result was 8%, which I mean that wasn't too bad. It was higher than I was expecting.
And the only person that did get that right, who said 9% was a data scientist who had worked in that area before.
So we realized that there's this gap between what people's intuition around product usage was and what it actually is.
The reason that we think that that was happening is because people are only answering little dots at a time with the questions.
Like you're getting illuminated about this little area and illuminate this area.
So you had some kind of calibration idea of usage in this spot and this spot.
But they didn't, you don't have this good kind of base level knowledge of like what's the kind of average click-through rate?
Or like how likely people generally to do something within some boundary?
So just fueling relatively educated estimates. And that's because people just couldn't answer simple questions for themselves.
At the time, you can't just go in and go, Oh, hey how many people have done this thing?
Or what's the drop off from this step to this step?
And it's too much effort to ask an analytics team to answer that, it's like pretty low value.
You kind of explain why it's valuable to you, it's just interesting.
To gather all these questions over time, do become really valuable cause they shape this intuition.
So we realized like, okay, we need to change our approach, this is not giving people the information they needed to develop this intuition.
And so what we did is we kind of blew the team up. We went very drastic actually and we essentially said, well, we need to focus on self service.
We need to allow people to answer these questions themselves, it's kind of common relatively easy questions in an easy way.
Like if it's an easy question to be used to answer and much better to do it.
So that's what we've decided to focus on, procuring it to like amplitude.
And the other thing we did is we thought, well the company is growing, it's getting more complex, and there's this really big kind of molehills of domain knowledge between each spot.
And so that's a really good argument for decentralizing the team.
Like you should put people into each of these areas and they can kind of become experts in this domain and that really helps as an analyst, having that expertise in the product.
You really provide much better insights if you can understand that thing particularly well.
So that's basically what we did, we blew the team up, that team ended up very decentralized and we just went from one extreme to the other.
And then sometime through last year, we're kind of speaking and we're like, this is a nightmare.
We'd manage to build and roll out amplitude and implement it and how to keep success in that we've got basically everyone in the product group that should be using amplitude, at least using it maybe not to the depth we'd like it, but they're logging in and they're checking some metrics and things, which is a great step in that direction.
But we have all these like centralized type things, like we managed to use your favorite term data governance, which is relatively centralized.
You have a taxonomy, you need to manage that centralized, you have amplitude and some have been modeling and things like that that is centralized.
So we have these analysts that are like really decentralized and we're optimized for kind of domain knowledge, but what ended up happening is the thing most of the time doing the centralized stuff because it was taking much longer to do.
So, we kind of realized there's an argument for both cases, like there's benefits having someone embedded and decentralized into teams and there's benefits having people centralized as well.
And what we kind of came up with this idea, we call it a hub and spoke model, I'm sure there's a better name for it, I'm sure someone's called it, there's an official name for it.
But the idea is, we're going to keep the instruction decentralized. And what we've done is we've initially centralized the team, so now we have a team that looks after product data analysis and are essentially combined at the moment. And what that does is it means that, we can develop a roadmap, a product strategy for analytics.
We can focus on these data culture problems and building the foundation of analytics. And what that also means is as we grow the team, so it we're hiring some more product analysts over the year to do this, is we can start scaling up and deploy those product analysts to actually do product analytics and not do all this other stuff. So they get the benefits of being in that domain area, but they also have the benefit of not having to do all this like distracting, centralized type work.
So they can actually focus on the bigger me problems of analytics, the centralized team can focus in on the foundation and like the self-service culture of analytics.
So, I mean, check back through the year and I'll let you know how it's gone, but I'm pretty hopeful about it, so far it seems to be working well for us.
Stef: Amazing, yeah, I totally agree with this, with the hub and spoke model.
I went through a similar journey myself, scaling the team at QuizUp, back in the day and I so much agree with you on this.
There are benefits of having the centralization, normalizing the way the company do things and just group of people supporting each other and building the good high impact things that do good for the data culture of the company.
And then there are benefits of the integrated analysis which says, or the integrated analyst, I guess, which is, for example, accidental sort of knowledge sharings is one of the things.
And you also get these, like the data scientists who guessed the 9%.
Like if you have no one who is the data expert and also knows the product really well, you might not get that kind of expertise and that insight about your product.
But totally agree with your approach on like trying to get the best of those worlds.
Nick: Yeah, hope it works.
Stef: I love calling it the hope and spoke model, trademark.
Nick: Or hope and spoke model.
Stef: Hope and spoke mode I love it.
Thank you for sharing that and this was actually a really good, I mean the journey from the centralized team and how extremely painfully you highlighted the bottleneck experience.
I'm glad that's going to speak to a lot of people. I hope so.
It speaks to me, it brings back dark memories
Nick: Yeah, it's tough.
Stef: Yeah. And so as this whole thing has been evolving, you have been changing, I mean your role has already changed in the last two years, this industry is just evolving so fast.
When I started doing product analytics, the term wasn't really even coined, and analytics engineer as a role that I just learned this year or something, it's really new.
And so how are you recruiting for these roles?
Nick: That's a great question. Yeah I'll talk to them separately.
So we realize to our product analysts, if you look at there's a DBT have a really good idea of a spectrum of skills, and it goes from data engineering, kind of data science.
I'm not convinced data science belongs to the spectrum but anyway, data engineering, analytics engineering, product analytics, kind of through to BI.
So that's kind of the spectrum of skills and right now our product analysts have this really wide skillset.
So they kind of go from BI, all the way through to analytics engineering.
They do modeling, they do data pool, they send data to systems and things like that.
And what we're really keen to do is focus those a little bit more.
So the product analytics do focus on the data analytics and the analytics engineering focuses on the analytics engineering side of things.
So for the product analysts, that actually means we can focus in on a narrow set of skills, and those skills that we're focused on now, yes we care about these technical skills but actually not as much as we used to.
Like yes, we expect build and SQL and they still need to data centered modeling and potentially do some, but what we're really concerned about and we can focus in on, is just purely the analytics skills, that your data literacy, your ability to communicate data, those kinds of things.
So I think a lot of the backgrounds, especially in Australia, it's not a lot of people with the job title of product analyst.
So we'll probably get, we'll probably get quite a lot of people from either someone myself from like kind of digital agency or the digital world or maybe some consulting as well.
I think that's largely where those people come from and there might be some skilling, but that's fine, it's a weird niche area.
So we expect that. The analytics engineer is the interesting one and we've been going back and forth on this a little bit, 'cause there's actually, we think there's actually two distinct types of analytics engineers that we need.
And one is maybe what most people think of analytics engineer as which is using things like DBT or you're doing a lot of sequel, you're building data models, you're like helping get a data from systems and systems.
It's not quite like data engineering with, it's not as heavy with infrastructure and things like that, but it's using tools to move data around and things like that, and model that make it useful for the business.
But there's also a really important front end skillset that we need, and like our unicorn is someone that has like a full stack experience, 'cause they can do everything but as truly unicorn.
But yeah, there's this whole other front end like analytics engineering skillset that we need, which is people that can help build the systems and processes of tracking data and getting it in the first place.
So that's not doing the instrumentation, but being the point expert for teams who are trying to do it.
You know, not necessarily owning what the events that attract but earning the framework and systems for getting attract.
So if we need QA and things like that, like making sure that that exists, probably managing the taxonomy or helping manage taxonomy and just ensuring data quality throughout the whole process, and helping us get people to trust that data, to call back to earlier.
So we're just working through that, we're basically hiring opportunistically.
So I think we we've put a relatively broad job description out for analytics engineer and said, you know, we don't expect people to meet all these requirements, we essentially take someone that's good at one of those things.
Stef: Yeah, exactly.
Nick: And hopefully two people eventually.
Stef: And for anyone who is listening and looking, I've had the opportunity to interact with Nick a bunch in the last few months, has been very fun and also the development team.
Nick: It has been.
Stef: I'm going to say plus one on joining Culture Amp.
Nick: Well thank you.
Stef: Where can they apply?
Nick: Oh, just through our careers page on Culture Amp, you'll find at the bottom of the site.
Stef: Nice, good little placement there, I like it.
So it sounds like you're looking to optimize those, like the full stack experience of the process as well and I think that is a really actually interesting question about this getting producers, data production, data consumers closer together.
And you mentioned like the QA part and the setting up the taxonomy and all that stuff, Where would you say that process is today, and who cares about it at organization?
Like what is the, who has a passion for that today?
Where does the developer standpoint stand?
Where does a PM standpoint stand today?
And what is the ideal situation in that?
Nick: You know, that's a really hard question to answer and the reason for that is, is that it's not consistent.
So we have two main products at Culture Amp, engagement and performance.
And the company product teams are largely split that way.
We also have a platform camp which focus on the kind of unified experience.
And each have a slightly different culture around it, and we have to actually approach each a bit differently.
So I've been appointed to the performance part for a while and we've been working largely with them, and you know one of their, the kind of senior PM in performance camp is an ex analyst from AWS.
So we have a really good partner stakeholder in a senior position in performance camp to kind of build these things.
She understands all the benefits, she's has the data literacy, she's able to help us make this impact.
In the other camps, we have people with skills just as great and just as strong, but I think the approach is different, because they have different histories.
The hardest thing I think we struggle with and I think most teams are probably at this point still, is that data and analytics is treated as a, most people call it logging for one and two, it's kind of treated as a nice to have.
Like it's thought about just before they shipped something, if there's time to add it or often after it's been shipped and so it will get partial data on a new feature release or something like that.
And that's probably the number one problem I want to tackle, is that I want to bring analytics in, you know, when we kind of start having purpose meetings which I got that from you Stef, thank you. Yeah, I think that's a really good idea.
Much earlier on in the product journey and I think one way we can do that is actually partner with our UX designers, because the time that they're starting to hand things over and settle on a problem, is a really good time to start thinking about measuring it.
You know, they define the user journey, also the UX designers are super interested in this stuff as well.
So I think we have a good ally there in kind of building this.
Stef: Awesome, yeah, it sounds like you're on a journey with the themes and with some of the sort of initiatives and like how you're going to support those product teams in being data driven through the teams.
There's an exciting thing happening and actually I think it's not a given that designers are passionate about this.
I mean, they definitely care about UX, but there is a set of designers--
I'm sorry designers if you take offense to this, I don't mean offense, but I feel like there is a set of designers also out there that sort of is like, you can't force creation or something like that.
And one of my favorite sort of designers that's super data-driven also is Lex Roman, who has been sort of a spokesperson for a lot of product analytics stuff.
She coined, or at least was early in coining the term growth designer, which is this way of viewing design from this perspective of like optimizing the user journey a little bit.
And I think she builds a community around that as well and she has a lot of material on sort of training people in doing that. So yeah.
Nick: That's interesting.
Stef: A lot of placement, another placement. Yeah, she's great.
Nick: I've heard of it but I'll have to look into it, that sounds really interesting.
Stef: Absolutely. Oh you should, we should set up a panel, so talk have beer.
Nick: Yes.
Stef: So, but sort of maybe to start wrapping up, I'd love to maybe hear you talk a little bit about sort of industry changes.
You've been mentioning your own both personal journeys, or professional, individual professional journey, and the company journey you know, a lot of tool changes, a lot of process changes, a lot of mindset shifts.
Like I was talking about earlier, product analytics or just digital product development is a fresh angle.
Product analytics is a fresh angle within that, and digital product is even already a fresh angle within like software development.
It's just also new and it's changing so rapidly.
So even if we look at just the past two years and then we could look at five years as well, but what is changing? What is the trend?
Nick: I think that the nicest thing about this trend I've noticed is that, it's become less of a cowboy field and things are coalescing around kind of accepted patents.
So maybe even a year ago, it was like for any given problem, there was probably three to five leading tools and then probably a hundred others you could probably use that might do some of what you need.
And I think everyone had some different combination of some of those tools, and it meant that nothing worked very well with anything.
Like it was pretty frustrating.
You know, you're trying to build a data stack that work together and you might use for example, DBT, but then are using Presto and Oh, it doesn't work Presto, that's like really frustrating.
I think they're working on that. I think as these things coalesce and become more standardized, and they're just kind of more accepted patterns, I think things will get stronger and easier to work with across the board.
And I think all those decisions around your what tool do we use become easier and easier, because you can just lean on someone else's learnings and lean on someone else's time spent solving problems, which I think is really good.
And I think that is actually making things a little bit easier in that front.
I think the biggest trend I've seen though is actually, it's two steps.
It's, one, a movement kind of, I guess back towards front end tracking and not necessarily front end, but generally front-end tracking and at least event based tracking.
Front end tracking has its drawbacks, it's not as reliable as using data from a backend you know.
Again from the JavaScript and browsers, et cetera, but there's something beautiful in its simplicity.
You can do a thing and you can see an event fire off, it's very, very literal and that really helps with data consumption, it really helps with data tracking.
And when you got all these other complex things, it's really helps to have just a simple, consistent model, but the next step to that is getting the data call your fund head events.
We've picked this up as well and I'm sure a lot it comes as, we didn't enforce the taxonomy for quite a while, and we ended up at this point where we had like you have a 900 unique events.
And we'd have survey launched, launch survey, surveys launch, we'd have like all these different versions of the same event type.
And then we started getting, cause we focusing on localization, then we started getting events in different languages, that it's not particularly easy to detect language and translate it, so we basically ended up just tying them all into untranslated object event.
It became practically unusable, no one knew what any event meant, I didn't know what any event meant.
So someone asked me to pull some data from the, I would, the only way I could do it was actually just reverse engineer it and like go and do the thing, see what events came through and work with us.
There's no documentation or ownership, it was like really, really hard to work with.
And I think one of the best things we did, which we were really resistant, like hesitant to do, because it's not nice, but we started to enforce a taxonomy.
And what that meant is we've tried to as light as possible and we try to do as collaboratively as possible, but thinking about the whole taxonomy of our product and making sure that people are using the same consistent type of event for the same thing.
The example I was given is like Spotify to our PM's, it's like, if you play a song from a playlist, or you play a song from a daily mix, you just want to know that someone played a song, and then you might want to know about where they played it from, but really just want to know where they played a song in the first place.
And traditionally people would probably track like played playlist or played daily mix and you would end up with all the possible ways you could possibly play a song and then you'd have to try and turn them into a one event.
And it's just no one's going to do it, it's not sustainable.
So having that taxonomy and getting that right from the get-go is worth the investment, is worth the time.
I think taxonomy management tools, like are pretty young at the moment, like they're pretty hard to work with, and we're looking at ways to improve that.
We're worried about taxonomy, how we manage it and things like that's pretty difficult at the moment, but it's still worth the effort.
Stef: Amazing, yeah I mean, you know, you don't know, I feel strongly about those as well.
It's one of those things maybe that, I mean you can give those advice, but when, yeah when you have to make the decision to go from implicit to explicit tracking, for example, or a free for all to a fairly standardized taxonomy, it's good to have a really clear reason behind why you're doing it.
And I think the experience that you're running with is a good one I guess, it's a good argument that you can make for getting people on your side on this decision.
Nick: Yep and I would also say though that yeah, the movement from track everything, to track just what we need, has been really helpful.
It sounds counterintuitive, like you kind of always assume you want all the data all the time, but you really don't.
When you want to analyze something and all the data is there, you don't want it.
You really want the things that have a strong signal.
Stef: Exactly.
Nick: I know Ryan at Atlassian talks about this, it's like a minimum viable user journey.
It's like let's not track everything everyone can do, let's look at what are the key things that matter, that we're actually going to care about, that means someone's getting value.
We talk about them as like a business event, it's like it affects the business that someone does this thing, and let's not track any more than that.
It's like a good balance between coverage but simplicity of analysis and understanding.
Stef: I love that, that is a really good, I think take home message also.
I'm about to ask you about some take home messages, maybe this will be one of them.
What is the first thing that teams should do to get on the right track, to start getting their analytics right.
Nick: It depends on where you're at right now, I think and your maturity and the size of your business.
If you're a new company and you're like a fresh startup, I would say get your front end events tracked.
Like just, don't worry too much about the tool, like make sure that tracked, there's a consistent taxonomy and you can get them into a database or something like you earn them.
You can work out, you can decide if you want to mix panel Amplitude or Looker or some cast like whatever down the track.
But having that data from the get-go is so valuable, there's so much you can do with that.
We've lost a bit of a history of our friend at events because the quality there is quite poor.
And it's only really in the last year that that quality is improved.
So it's really hard to, for example, we really hard to compare year on year until we have two years of data now.
So getting that right is really, really worthwhile.
Actually, I think at any size and any stage that's worth doing, even if you're a huge bank and you've got an app and things aren't great.
Right now it is worth the investment of getting that up, that's probably the number one thing you can do to improve your data quality and accessibility of analytics to have your stakeholders are.
And yeah, it's pretty bad taxonomy, it is a bit of extra work, but it is worth the work.
If you can figure out a way to get your stakeholders to help you contribute to that in a reliable way, that's great, but if you have to have a centralized person that's doing it for now, that's also fine.
That's where we're at, that's what we're trying to, we're trying to get to that. Early a bit, but it's hard.
Stef: Yeah, I love that. I think that's a good take home message.
Starting small basically that's what you're saying.
Like there's a set of events that just start somewhere.
Nick: Yeah and the other thing I would call out is that regardless of what you're trying to do in analytics, it is truly like an exercise of patience and iterative learning.
You're always dealing with old change, you're dealing with lots of stakeholders all the time.
You've got data complexity, you've got lots of things that make it kind of hard.
You might have an idea of like this beautiful end state, and it's often not a technical challenge, nearly never is a technical challenge.
Most of the challenges are working with your org, working with the teams, your stakeholders to get there.
And so you always recommend have a goal, break it down into small bits and focus on what you can just get done right now, because firstly things are changing.
You never know what the end goal might be and things will take longer than you expect.
Stef: Yeah. It is work but it is work that pays off on the end and it's.
Nick: Yeah.
Stef: We're going through a cusp, like there is a cusp and sort of, there's a shift happening in the world and people are realizing this.
If you are the first person in your company to realize this, then you go, you go girl, we got it back.
Nick: Yeah, you're in the prime position for when it does take off.
Stef: Yeah, exactly. Who should they call? Where do they want to learn more stuff?
What can you share with a person, a single person who's trying to become a champion for this right now?
Nick: I think the best community I've found for product analytics in the world is probably locally optimistic.
So you can just Google that I think, and they have a Slack group.
And as far as I can tell that's, it's a really good group of like people from pretty much every company I've seen doing data analytics from Google and Twitter through to like lots of smaller and medium-sized startups.
If you want to learn about this world, I feel like that's probably one of the best groups out there.
That's probably where I'd start and probably where I spend most of my time.
The DBT community is also really good, a bit more focused on data modeling and sometimes analytics engineering but worth knowing about as well.
Stef: Awesome, thank you so much for sharing your words of wisdom with everyone in the world who hopefully is listening.
Nick, it was great to have you on the show, I hope we'll get to part two soon, where we'll resolve the emergency call as one.
Thank you so much Nick for joining us.
Nick: Thank you so much for having me Stef.
Subscribe to Heavybit Updates
You don’t have to build on your own. We help you stay ahead with the hottest resources, latest product updates, and top job opportunities from the community. Don’t miss out—subscribe now.
Content from the Library
The Right Track Ep. #12, Building Relationships in Data with Emilie Schario of Amplify Partners
In episode 12 of The Right Track, Stefania Olafsdottir speaks with Emilie Schario of Amplify Partners. Together they discuss...
The Right Track Ep. #11, Defining North Star Metrics with Che Sharma of Eppo
In episode 11 of The Right Track, Stef Olafsdottir speaks with Che Sharma, CEO and Founder of Eppo. This conversation includes...
The Right Track Ep. #10, Getting to Know Your Users with Boris Jabes of Census
In episode 10 of The Right Track, Stef speaks with Boris Jabes, CEO and Co-Founder of Census. They discuss the impact of SaaS on...