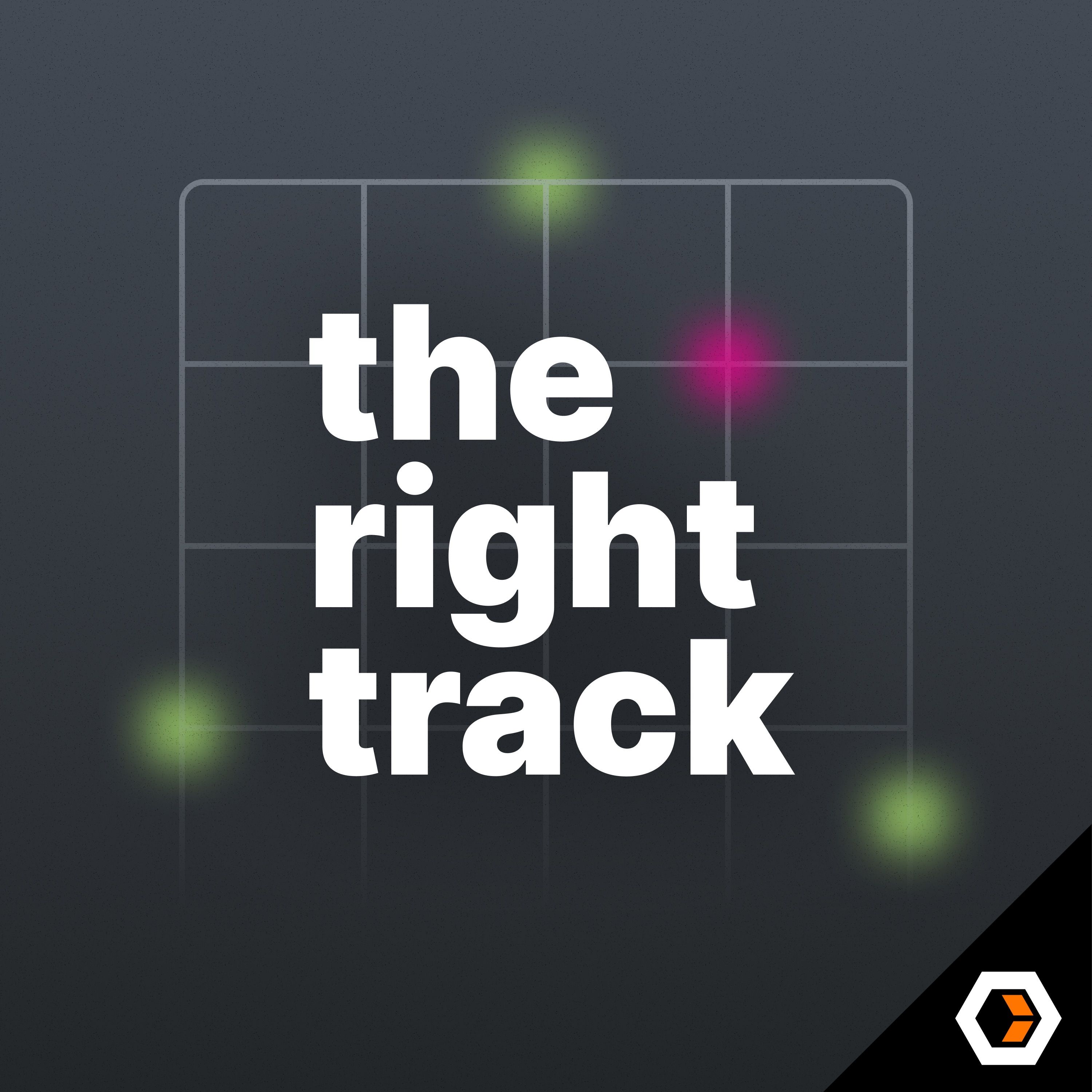
Ep. #12, Building Relationships in Data with Emilie Schario of Amplify Partners
In episode 12 of The Right Track, Stefania Olafsdottir speaks with Emilie Schario of Amplify Partners. Together they discuss impact-driven development, actionable tactics for prioritizing relationships within data teams, the quandary of data job titles, and insights on improving data trust.
Emilie Schario is a currently Data Strategist in Residence at Amplify Partners. She was previously Director of Data and Business Intelligence at Netlify after a stint as GitLab’s very first data analyst. Emilie is an admin in the Locally Optimistic Slack community. She is also a wife, mother, Crossfitter, bookworm, and a graduate of Princeton University.
- Down with "Data Science" (w/ Emilie Schario)
- Share Your Data Insights to Engage Your Colleagues by Paige Berry
- The Types of Work a Data Team Does w/ Emilie Schario
- The Missing Analytics Executive by Benn Stencil
- The First 90 Days by Michael Watkins
- Ep. #6, Domain Expertise with Laurie Voss of Netlify
- Ep. #8, Defining the Data Scientist with Josh Wills of WeaveGrid
- Ep. #9, The Argument for Less Specialization with Erik Bernhardsson of Modal Labs
- dbt
In episode 12 of The Right Track, Stefania Olafsdottir speaks with Emilie Schario of Amplify Partners. Together they discuss impact-driven development, actionable tactics for prioritizing relationships within data teams, the quandary of data job titles, and insights on improving data trust.
transcript
Stefania Olafsdottir: Hello, Emilie, and welcome to The Right Track.
Emilie Schario: Thank you for having me. I'm so happy to be here.
Stefania: I am incredibly excited about this conversation. You're a legend, obviously. Love watching your talks and reading your content, and I've loved so much of the stuff that you've built in data cultures so it's an honor to have you here. Could you kick us off by telling us who you are, what you do and how you got there?
Emilie: Yeah. Super happy to. My name is Emilie Schario. I am currently a data strategist in residence at Amplify Partners, an early stage, VC firm focused on technical founders. I was previously a director of data at Netlify and prior to that I was the first data analyst at GitLab, watched the company grow from 250 to over 1,300 people, spent a year as Interim Chief Of Staff to the CEO. Really got to do the hyper growth thing there which was special. Outside of data, I am a wife and a mom and I live in Columbus, Georgia with my husband, my son and our dog, Beau.
Stefania: Awesome, love that. Will we get to hear Beau in this interview?
Emilie: Probably not, probably not. He's pretty well behaved. He is my office coworker full time.
Stefania: Awesome, awesome. That's a great coworker. What was that transition? I'm curious, going from... I mean, because definitely when you are a good analyst, data scientist, whatever you call it, it's often because you have a really strong business mindset as well. I'm curious, how did that transition into chief of staff happen?
Emilie: Well, I was at GitLab. I had been there for over a year at that point and because of my role in the data team, I had gotten a lot of exposure to the CEO, Sid Sijbrandij, pretty directly. I really enjoyed working with him and he decided he wanted to hire a chief of staff. He had a certain profile of years of experience that he was looking for, and I reached out, had a lot of the GitLab context and said, "I'm very interested in this role."
And he said, "You're great, I think you're great. But you're not the experience level I'm looking for. I'm looking for a more senior candidate." And I said, "Yeah, but I could start now while you hire a more senior candidate." And so he brought me in-
Stefania: Solid.
Emilie: Yeah, he brought me in in this interim role and it took nine months to hire the chief of staff so we really had to figure out what the role was and what the profile was and how we were going to find them. In the end, GitLab hired an incredible chief of staff in Stella Treas, I loved working for and with her. I learned a ton from her, but the really interesting thing about that role is I got to go from thing in the business to thing in the business, each problem, basically firefighting with my data hammer, right?
Where the skillset that I had was this data super power where I could sit in a call and a question would come up and I would have the answer two minutes later in a way that was very different from other folks in the business who had to go ask the data team. The real underlying problem there is that data team was in a service model, everything was a question for the data team as opposed to people being empowered with the data that they needed.
When operating in that kind of environment, when I just had the data or could get it myself, it became this super power that let me jump into any part of the business. I learned a ton in that role and I think it made me a much better data professional because of my time not working in data.
Stefania: Yeah, exactly. And maybe it sparked a lot of the culture that you eventually built that at Netlify, I would assume.
Emilie: I think what I saw was this idea that the service org doesn't scale, and if you're going to have a data team where people are just going to be asking you questions, well, then as you add more heads to the business, your data team needs to grow exponentially, right? Because the number of questions coming in to that team will grow exponentially and nobody wants that.
Nobody wants to give the data team an exponential number of head counts, especially where it's so hard to quantify the impact of the data team and so when I went into Netlify, a huge part of what I did was, "How do we turn this data team into a proactive, strategic asset to the business instead of a reactive service org?" And I think we were pretty effective at that.
Stefania: What a journey. I'm sure you get this a lot as well, people reach out to me all the time, both about when they're head hunting for data scientists or for data roles and they don't know exactly what profile they're looking for. On the one hand they're looking for like, "Do you know someone?" And on the other hand they're looking for, "What is the recipe of a LinkedIn profile or a background that I should be looking for?"
And I think one of the most challenging things about that is it's often just they don't necessarily have a lot of experience if you want them to come in and be the first data person, because often the people that have a lot of data experience, they just are burnt out from data and they don't want to be the first data person again. And so you're maybe looking for some hungry people, basically, and those data generalist roles.
I think it's super interesting that you went and became chief of staff and I wonder what happened first, your skills for that role made you a good data analyst which made you an acceptable candidate to the chief of staff, versus all of the incredible skills you acquired as chief of staff and then made you an incredible data leader?
Emilie: I think I'm a process thinker and when you come to it from that, that's part of why I was good at data, was that so much of data, especially in those earlier stages of an organization is understanding how data flows through the organization. That's a reflection of the processes that are creating that data, right?
So if we think about Salesforce for example, the customer journey that is mapped in Salesforce creates a bunch of data that we see in our data warehouse, and coming from that process piece into understanding the data flows made me a better data analyst. I think then having understood so many of the processes in the business and having this wide view of the company through these processes and data flows, made me an effective interim chief of staff.
Stefania: Yeah, this is super interesting. There are so many things to unpack just in that intro, so we'll touch on a lot of them later in the conversation, especially around building the data culture and the proactive data team versus the service org, I guess. But to kick us off into that feeling that we all share, some horror stories and beautiful stories at data, can you tell us an inspiring data story and a frustrating data story?
Emilie: Yeah, which one do you want to start with?
Stefania: I will leave that in your hands, but it could be fun to start with the frustrating one and leave on a high note.
Emilie: Yeah. Actually I could not name a job and have the same story at all of them, which is a combo of something really important broke and nobody knew until it was too late because so much today around data orgs still feels like it's in this pole model, right? "Go refresh the dashboard or go run the query," instead of information being pushed to people.
And I could tell you really a gajillion, an unquantifiable number of stories that are some combo of, "We needed this number, we thought we had it, we thought the dashboard was working. It wasn't, until someone refreshed it and we realized that the number's didn't make sense."
I think part of the arc of my career has been thinking about how we address those sorts of things and how we catch them sooner. I think the other side of that would actually be the inspiring story, which is how technology has enabled us to do more of that.
So I think about times at Netlify where we were proactively catching those things more and more often, and in the last couple months that I was there it felt like the, "Hey, this thing is broken," sort of comments were few and far in between. Those were now the exception, instead of the rule where earlier on in my career it felt like 80% of the inbound messages to the data team were, "This thing is broken."
Stefania: Oh my God. The horror story. You're actually touching on something here that we didn't cover a lot in your backstory because you mentioned a few of the key milestones in how you go there. But you already had a substantial data experience, even before GitLab.
Emilie: Yes. In some ways, my claim to fame is that I discovered DBT before a lot of other people. So I joined DBT Slack in December, 2016. We were using DBT at my then job at Small Direct Club, and it was interesting because I was person number like 50 in DBT's Slack and now there's 25,000.
My career has really grown with the Slack community, and so my technical skills, my maturity, my thoughts as a leader, my mental model for how we build and grow data teams, the impact that the data team should have on the business. All of that has grown as the community has grown, and so I've been so grateful. It feels almost like I've been on a parallel journey with DBT and the DBT community that has been really special for me.
Stefania: That's incredible. That's actually a really beautiful story, like a growing up together story. When you first got into data, how did that happen and why did that happen?
Emilie: Well, I started off by getting into tech. So I am an army wife, my husband is in the military here in the US and military families in the US move on average once every 2.9 years. But just by the nature of his career, we have moved a lot more than that. We were college sweethearts, we were pretty serious by the end of college, and I looked at him and I looked at the career ambitions I had and I said, "If I'm going to make these two things work together, I want to work remotely. What are the industries where that's going to be possible?"
I looked to startups and technology as the sector, so I joined a post grad fellowship called Venture For America that takes recent college graduates and puts them in startups to work in non traditionally startup cities. So not in San Francisco and New York and Boston, but in Baltimore and Cincinnati and New Orleans. So I joined an early ed fin tech company called Alloview that helps school districts manage and allocate their budgets.
When I was interviewing with them I was mid writing my senior thesis and my senior thesis was a very technical analysis of how certain behaviors were good or bad indicators of voting patterns for women. I was doing this analysis in our... I wasn't very good, I didn't know what a function was and so I had all this code that was copied and pasted where the only thing that changed was the variable.
But they saw this very typical R output, a bunch of statistical analyses, and they knew they were getting this fresh out of college grad and so they took a chance on me and I really appreciated it. I was working remotely pretty much from the beginning with them. I was in that role for a while and learned a ton there, including learning how to work which is a thing you have to learn in your first job.
Stefania: Totally. Navigating relationships and-
Emilie: Yeah. It was hard, but great. I joined as person number nine, watched the company grow to 30, which felt so big at the time. Then from there joined that Small Direct Club role where they were implementing... That was my first taste into data in the analytics sense. The work I was doing at Alloview was very much around data implementation and integration, so helping our customers use our software and configuration. Much more like ETL systems integration sort of role. When I moved to Small Direct Club, one of the first analyses I had to do was customer acquisition costs for digital ads.
Stefania: Oh, awesome.
Emilie: Right. Facebook, Instagram, tools like that. It was my first foray into analytics and they were... This is now 2016, they were building what today we would call the modern data stack. They had Red Shift, nDBT, nDBT Cloud which at the time was called Center and Looker and Stitch. They were also using Stitch. That was the first foray into the modern data stack for me, and then it's really just evolved from there.
Stefania: Incredible. Yeah, I just wanted to touch on this because it's always such an interesting path that people go into data science, and for software engineering, for example, you typically study software engineering and then you become a software engineer. That is just so far from being typically what people do for the people that become great data leaders or analysts. That's certainly not the case, necessarily. I mean, we've definitely seen a growth in people studying data science, but most data leaders that I know, they didn't study that.
Emilie: No.
Some of the best data folks that I've worked with don't have college degrees. Others have PHDs, right? So I don't even think we need to think about education as like a formal path into data. I'm all for school, I loved my four years that I spent at college but I don't think that that's a prerequisite to a career in data and we shouldn't discount people who don't have it because so much of what we learn in school doesn't translate into real world application for data.
Or, we studied something completely different that's not related to the work in data anyway. And so I don't even think that education should be a prerequisite for most data roles, maybe for high level, advanced, applied ML, statistics where we're talking about certain advanced technical subjects. But the vast majority of data roles, in my opinion, do not need any sort of education requirement.
Stefania: Awesome. Okay. We're in the midst of your frustrating data story and migrating into your inspiring data story. You've talked about the silent failures, basically. Do you remember a moment, do you remember like, "Oh my god, someone's just pinging me here and I don't know what's happening"? Can you share that? Literal stories?
Emilie: Yeah. So I was in the Target on Skybow Road in Fayetteville, North Carolina, it is three o'clock on a Sunday and my cellphone rings. "Hey, we're prepping some numbers for the board meeting on Tuesday and the dashboard doesn't work. I need you to look at it." And I have a cart full of groceries, I'm like, "It's going to be two hours before I get home." "We can't wait that long." But it is what it is. And so they did, they had to.
But no, that sticks out as like, "There's got to be a better way." I don't think any of the dashboarding tools are particularly great about this, if you want to know if a dashboard is broken you basically have to run it on a schedule all of the time and that has its own consequences. Especially around compute resources.
I think there are some toolings starting to get better, like integrating with your DBT project and reading the code of your BI tool and things like column level lineage that make it easier for you to map the effects of changes up stream, to changes in your BI tool, more of it being treated like code.
Interesting tools now that are reading and extending the lineage stack of your DBT project. I think there's a lot being done, but I haven't seen a real solution yet, but I'm glad to see that a lot of really smart people are working on it.
Stefania: Yeah. Everyone is solving their own problems, that's what's happening. Okay. This was a great, great story, and maybe just a side note into that, I think one of my learnings also and I'm curious to hear what your journey through that learning was. I talked about this a little bit also with Josh Wills, he shared his perspective on this at Slack.
The learning for appreciating data needs of executives, obviously it matters a lot but there are also a lot of other things going on and you, as a data scientist, you get excited about a lot of things. But ultimately also, the board or the marketing team has to report on just things that you consider maybe vanity metrics, or they have to tell a story around the data that fits the strategy of the company around fundraising or something like that.
I remember always this feeling like, "Why am I always getting pinged about board meeting data the night before the board meeting? Why can't we plan ahead a little bit for this?"And yeah, I just remember that feeling and not understanding why it had to be like that, but always responding to it and we were always on it and we always worked through the data and created a deck narrative and all those things.
Now I do, and obviously with time you start understanding the different needs and purposes of the data from those perspectives and particularly now running a company myself, I totally understand that preparing a board meeting, you wait with it until you absolutely have to do it because you want to wait for the last data to pop in, you want to finish all the stuff you want to do.
Do you remember a journey through that perspective of how you collaborate with high up stakeholders, high up executives on these things and your journey through and how your patience towards that changed? Was it always there?
Emilie: It was always there, because in a lot of ways as an IC, I loved the opportunity to work with executives. Here I was, a lowly data analyst, what today we would call an analytics engineer, and getting a chance to sit in meetings with these folks with C level titles. I loved that and I always enjoyed doing that. When I transitioned into a leadership role, I wanted to give my team members that opportunity to be in the room, and so I think the frustration is really that people, one, don't anticipate that the board meeting is coming so we know we're going to have some last minute requests.
But, two, recognizing that when it comes to working with executives, the data team will always be a service organization, period. And coming from that perspective of when we talk about proactive data teams, when we talk about how we enable analytics for the rest of the company, we need to recognize that working with executives is an Asterisk on the problem. The CMO is not going to want to slice and dice something in Look or Transform or whatever tool you're using, right?
What they want to do is just ask someone and get an answer, because that's what they do in every other part of the business so why would data be any different? And so coming to it from, "This is a group of people that we are going to treat differently,"because the other part of thinking about it that way, every team member's time is worth a certain amount of money to the business and the CEO's time is more valuable than that data analyst.
It is more worthwhile for the data analyst to sit with the CEO and answer their questions than it is for the CEO to try to answer their own questions in an Excel spreadsheet. So when you come to it from that model, it's like, "Oh yeah, of course we're just going to have the data team do the analysis for them and help as the questions evolve." The question is where do you change?
I think it's everywhere else in the business you need to think about enablement and helping people understand what metrics mean and where they can find information on their own. I don't really believe in self serve analytics, but figuring out what some version of self serve looks like at your organization. I think the best model that I have ever seen is one where the business defines a number of metrics and self serve is around allowing people to discover those metrics, and then slice and dice those metrics appropriately.
That was the model we had at Netlify. We used Transform as a metrics repository, people could slice and dice as they wanted and expand from there. But everyone knew that those were the numbers that we cared about as a business. It's also a great way to standardize on the definition of things. I have heard horror stories from friends who work at other companies where the marketing team counts revenue as top of funnel revenue, so all the people and all the purchases.
But the finance team subtracts refunds from revenue, so marketing and finance have different definitions of revenue and that sort of story is just scary for a lack of a better term. It's a horror story, truly, when it comes to data. And so being able to standardize the whole business on, "This is the only definition of revenue that applies to this company," just unlocks a lot.
Then everyone in the organization knows this is where the definition of revenue and the number for revenue lives, and that's very different from the way most traditional BI tools have handled it, which is that every dashboard might have a revenue tile on it. And, when something changes or you want to apply it to a filter, it doesn't apply it everywhere so now you might have incongruous definitions of revenue across the business.
Stefania: Yeah, exactly. This is a great story. I want to add to this with a standard definition of a metric. I also remember there's a classic issue of ad blockers and things like that, with the definition of a user and then when you look and try to consolidate the count of daily active users using other datasets, for example, locks. Server locks. I remember at moment at Quizup where we discovered that according to the server logs we had, I think up 10, 15% more daily active users.
Obviously the COO and the CEO, they were like, "We have to fix that." Eventually though what we did is we agreed that we're going to use the other definition of daily active users, because that's also what all of our other data will be able to sliced and diced on. So we're aware that we might be under representing our daily active users in total, but that is going to be our source of truth, that is how we count and represent our daily active users.
Because, if we start mixing in that other definition, then we'll start to see reports where the Tuesday daily active users were 20,000 here but 15,000 there, why is that? So I think this is a really great point.
Emilie: Exactly, and I think that is a business decision, not a data decision, right?
Stefania: I agree.
Emilie: So as data people, we discover the problem, we understand the root cause, we make a recommendation but at the end of the day that is a business decision that our business partner or executive stakeholder needs to make. And so, it really is a partnership through and through, not just understanding the business problem that you're trying to solve.
In your case, what is daily active users, but also how are we going to use this data and how do we need to be able to slice and dice it? And it is just as important that we understand what are the technical limitations of the data we're working with, if we switch to server logs we would not be able to slice and dice it in these ways.
Stefania: Exactly. Ah, these conversations. Awesome. And so moving over to inspiring data stories.
Emilie: Probably one of the highlights of my time at Netlify was this Insights feed that we built that you talked a little bit about with Laurie Voss. I think a lot about data teams that are stuck in the service trap model, this IT model, question comes in, answer goes out. This doesn't work for the reasons we talked about before, your headcount would need to grow exponentially and you'll never get to all of the things, and people will always think of your team as just answering other people's questions.
I knew and I know that data teams can be more impactful than that. So probably been there for a couple of months when what we started doing was carving out what we called Insight Time. Take an analysis you're working on, anything, it could be an analysis you're working on to answer someone's question, and just turn it 10 degrees to the right or to the left. Just look at something a little bit outside of the scope of the analysis and see what you can find.
Sometimes you're going to find nothing, but sometimes you're going to find something interesting. And so it started off with just take half a day each week, that can seem intimidating to people so make it two hours, time bound it. The goal is just like, "I'm going to exploring in the data for a little bit for two hours to see what I can find." So it started small, with looking at users sliced and diced differently for the same analysis, or instead of looking at this page, "Let's look at this other page on the marketing site and performance there."
We started off with this little block, and I was more than happy to run interference for my team to by them this space to be able to do this. It started off with half a day and it took some time for people to get used to it. How do you right size an analysis to be able to be done in that window? How do you ask good questions?
Eventually what we saw was people would do the analysis, we had a template in GitHub that we used that would allow people to follow the journey if you were interested in it. But then at the end of all that we would push the information to the company. Remember earlier I talked about how so much around data still feels like this pull model? One of the things we did was start pushing this information out.
We did this in two ways, the first was we had a Slack channel called Internal Stats and Graphs where we would post a summary of the analysis. "Here's the thing I found, here's why it's interesting and here's a chart that illustrates the story," as well as a link to our Insight Feed post which was essentially an internal blog where you could read more detail, more takeaways, what was the methodology here.
What we found was very quickly the number of inbound asks to the data team started going down because people were now getting information pushed to them and that was shaping how they thought about the company. It was a really powerful thing to me because it was very hard to convince people to carve out this time at first, and as we did it 12, 16 weeks in people realized it was the most impactful work they were doing every week.
And so, they were carving out more and more time to do insights. I remember specifically this one meeting with the VP of Product where he said, "Oh, the first thing I do every day is log into the Insights Feed.
Stefania: Oh, snap. That's incredible.
Emilie: Yeah, it was such a powerful way of changing this push information, "We are going to tell you this really wonderful thing we found." I think most people on that team would tell you that it really changed how they thought about data organizations, so much so that Paige Bury, a staff data analyst at Netlify, she wrote a blog post for Locally Optimistic about how she shared her insights.
I remember after it was published, Tristan Handy linked to it from the Analytics Engineering Roundup, and the comment he made was something to the fact of like, "This seems so simple but yet it is so powerful when you do it, and so many people don't do it."
Stefania: Yeah. It's a tough challenge to push away all of the things that are being put on your desk or all of the different things that you have to do, that you feel guilty about not doing and to invest time in something like that.
Emilie: But I think what we found over time was that it became easier to prioritize because we saw the impact we were having with it.
Stefania: Exactly. So it's about building a flywheel basically. Like convincing some early birds to start participating in this, doing them internal case studies basically, building a flywheel for why people understand the value of doing this, and then more and more people get sold on the concept. This is the case for so many of the things in building data cultures, of course just in building cultures in general.
But yeah, I'm so glad to hear your version of this story because I really appreciate this perspective and I think what's important here is the data team at Netlify was lucky that they had a data leader that had this insight and that had the drive to put this in place.
Do you think an individual contributor, anyone listening, an individual contributor that maybe is reporting somewhere to the CFO or is reporting to even just a product leader or reporting to someone that is not an experienced data person, what recommendations would you have for that person to start building that flywheel internally if they don't have the buy in?
Emilie: It is certainly hard and I don't envy the challenge, and I'll start there. But I think the easiest way to start is to just take the analysis you're doing and turn it on its side a little bit. And so if you think about the way a lot of analyses work, let's use the marketing attribution example that I talked about earlier, which channel is most effective on our customer acquisition at selling our widget?
That's the first question. Then what's the follow on question? It's which ad on that channel is most effective? If we break it down by ad across all channels, is that question... is it still the same? So anticipating the questions you're going to get could be one way of going through, not just delivering on the question around which channel is most effective for customer acquisition costs, but what are all the ways they're going to want to slice and dice this?
Then not just looking at customer acquisition cost, but the way to shift the analysis ever so slightly is which of those customers go on to have the highest lifetime value? Or have the highest initial spend amount on that first transaction?
Just finding something a little bit smaller or a little bit outside of the bounds of the analysis, that is a good way to start getting in the habit of people seeing you as not just the number fetcher, but the data expert in the room. That's the perspective that you want in order for people to see you as strategic, not just, like I said, a number fetcher.
So I would start there. Then the other piece is a lot of times I've heard questions from people in distributed data teams where maybe they're across many departments or every team has a data analyst but there's no unifying data org. In which case, my recommendation is to start with a Slack channel. You don't need to have a weekly meeting to sync, but a Slack channel where you're pushing information to each other.
I think it can fall into two buckets, the first would be something like the Data Reads channel that we had at Netlify which was just industry articles and interesting perspectives and technical tutorials and things that people were keeping a pulse on and found really interesting. But the other side of it was the internal stats and graphs channel that we had at Netlify which was about pushing out things we found in the data, and you could do that.
You could always start small, you don't have to start by sharing it with the whole company, right? You could start by sharing it with the other data analysts, even if they're not in your organization or the other analytics engineers, even if they're not in your organization. Slack can be a great way to share asynchronously and not make people feel like they have to hop on a call .
Stefania: I think these are spot on action items to take in, so anyone listening, if you're struggling with getting and finding that time to make something even more cool than you're making currently today and build more leverage for yourself personally, listen to Emilie on this one I would say.
Emilie: And I'll add too, there are all these stats on the internet about how much time employees waste on email and other work communication tools. Quit them for an hour, be a little bit more productive next Thursday and just get it done. Block off two hours on your calendar and just take some analysis you're working on, spin it a little and see what happens.
Maybe you'll find nothing, in which case it was an experiment, you tried it, it didn't work. But try it again the following week and try it again the following week and try it again the following week, and I think you'll find that over time it will start to pay off in dividends.
Stefania: Yeah. One great aspect on this to keep in mind also, there was a great tweet from TJ Murphy that talked about the difference between software engineering and data science and how known the output will be based on the effort that you put in. So he's talking about that software engineering has high variance in the amount of effort it takes to get out a relatively fixed output, but while data science has a high variance both for the input or effort, and the output. So just be prepared for that, some of these moments that you take, you won't get much out of them but stay with it, I guess.
Emilie: And null results are interesting.
Stefania: Good point.
Emilie: I think there is this worry that if it's not a flashy takeaway, it's not a takeaway. But people who land on the green version of the homepage or don't convert more often than the blue version, that is also an interesting takeaway and it's not flashy. It would be much more interesting to say, "The green page is twice as likely to get someone to convert," or whatever it might be. But null results are also important because they help shape the way we think of the business.
Stefania: Yeah, that's a really great insight. Okay. Awesome, thank you so much, Emilie, both for the inspiring data stories and the frustrating data stories and the intermediate tangents that we went on which we'll now use as input into our next step in the conversation. I'd love to get some thoughts on how you see the industry has changed, and sometimes it's fun to think about that in a two year scope, sometimes it's fun to think about it in a longer scope. So over to you, what do you think, how has the industry changed?
Emilie: A lot of people still think of data teams as number fetchers for others, and it's not until you work at a place where the data team is strategic that people change their mind. I think it is nearly impossible to change a large organization's mindset around the data team as something other than IT, if not from the top. So all these organizations where people are struggling in service models or in IT, ask for data, get sent a file for you to do analysis in Excel, world, I don't know how you change those. But we're seeing more and more strategic data organizations pop up and I look forward to that trend continuing.
Stefania: That is a really great insight actually. I love that you highlight that. Most of the time when I ask this question, I get a lot of about tools and infrastructure and pipelines, and plumbing and how that's all changed. But this is a really great perspective on that. I think one thing that speaks to this is when I started Avo, a lot of people were skeptical of us targeting data as a buyer because they didn't have budget, typically, and they were in the service org and they were a cost center of the organization. But that has certainly changed so, so much just over the course of Avo's lifespan, which is since early 2018.
Emilie: If you look at the modern data stack and the tools that companies use to get started, just to get rocking and rolling with data right, you've got an EL provider, you've got a data warehouse, you've got some sort of BI, you've probably got some scheduler. And so it takes four or five tools just to go from zero to a dashboard, and what I think is there's companies that are willing to invest because they hear and they see the promise of data.
But then for companies to continue to invest, what they're looking for is data teams to move the needle, and the way for data teams to have the budget and be able to buy the tooling that they need and get the things that they need to be effective. It is certainly one where they need to be moving the numbers in a myriad of ways, whether it's working closely with growth or helping distribute insights across the business.
Whatever the case might be, I think those are the things that allow data teams to have the budget and the headcount that they need to be successful and impactful to the organization.
Stefania: This is such an interesting conversation. I think it could just be a good segue into talking a little bit about, or probably spending the majority of the rest of the time talking about data cultures and how to build them. I want to kick it off by just talking about data trust. I think that often sparks so many interesting conversations. It's an endlessly common phrase, "I don't trust this data." What's your perspective on why that is and how to solve that?
Emilie: The data seems so separate from the consumer of it in a lot of ways, but I think that is the problem. It's interesting that you mentioned earlier that usually people talk about the tech and the tooling and how all those things have changed, but I think those are not that interesting of problems. I mean, it's great, I appreciate the modern data stack. My career is thriving because of it. But the much harder problems to solve are not, "How do I move data from Salesforce into Snowflake?"
The much harder problem is how do I get buy in across the organization about how we're going to think about metrics? What it comes down to is really building a culture of trust and communication throughout the organization, and what I've seen be most effective is thinking of stakeholders as business partners.
At Netlify the way we implemented this was a phrase that I actually stole from Erik Bernhardsson, a recent podcast guest of yours, "Where data teams should be centrally reporting but locally prioritized."
What that means is you report to a manager who understands your job, you have a boss who can help you on career laddering and can help you understand what the right milestones are, it can help you navigate a tricky project, it can talk to you about the soft and the technical side of data. But then you also are working very closely with this stakeholder in the part of the business who has the pain point that you're looking to help them solve.
So you're not supporting them right, this isn't like you just answering the question for them. It is about a business partnership where you are also a part of that finance or product or growth team. You're in their meetings, you understand what they're working on and so you eventually get to a place where you get to anticipate the problems and the questions that they're seeing. That partnership is what sparks the impact that these groups can have together.
The data person who just produces a one off analysis and throws it over the fence and sends it to the head of finance, and the head of the finance is like, "I don't trust this data, these numbers don't make sense." Well, the data person, if the numbers don't pass the sniff test, the data person would've caught that if they had the context from finance right.
And so starting with relationships first, numbers second, allows us to insure that we're building on something much more important than data. It allows us to understand not just, "What should the SQL query look like? Because I'm going to write it for you because you just don't know how to write SQL." It's instead a, "What is the problem you're trying to solve and how can I help use my skills to help you solve your problem?"
Stefania: Ah, I love that. I couldn't agree more with the relationship building. It's one of the first things I always recommend to people as they're starting out in a new data role, and it's one of the things I mentioned when you were talking about just learning how to work. My first professional work role related to what I studied, excluding things that you did when you're a kid or something, it wasn't data.
I think a lot of that, a lot of the skills, I learned how to program in that role even though I would probably never qualify as a software engineer anywhere. But that's where I learned that, and I learned how to work with datasets, but I think my biggest learning was really learning the hard way how really important it is to really build relationships with the stakeholders.
Awesome. Incredible. Thank you so much for that insight. I love that we're talking more about culture than tools. I agree with you that those are the more interesting parts of this. The hard problem is not piping data, it's how to build buy in and desire and curiosity around data across the organization.
Emilie: Yeah, and like I said earlier, your data is a reflection of your process and you get a culture and people buy in to the process, and then the good data quality is a result of that. But that starts with the relationships, and so if we always try to fix the data afterwards, we're going to like a dog chasing our tail.
Stefania: Yes, wow. I couldn't agree with this more, and I think that's one of the most impactful things you can do also. I think product analytics data is one of the most complex sets of data that you can work with because it is ever changing, it's raw analytics, advanced structures that are ever changing. It changes every time you release a product, you have a new goal that you have to measure the success of so you have a new type of metric.
You're always looking at the high level KPIs of course, but you then have granularity in those metrics that are some sort of indicators and inputs into your high level metrics. So you're always creating new metrics for your product releases, and you're always creating new data structures for your product releases.
I think the most impactful thing that I've seen happen at organizations for data quality is when the product engineers actually start caring about the analytics data and measuring and understanding the impact of the products that they release. That's when you start seeing good quality data get created for every single product release, and they care about it.
Emilie: I'd be curious, do you see that start mostly with growth engineers?
Stefania: I would say not necessarily, but I think they are often in the role that works the closest and the tightest relationship with a data analyst and a product manager. They're on a team that specifically has really strong growth metrics, and so I think Erik Bernhardsson put this really nicely in my interview with him where he was saying and highlighting that when you have to sync across teams, that takes weeks.
When you have to sync within teams across individual, it takes days. When you have to sync within a single individual, that's a dream state. And Josh Wills also highlighted that a little bit on my interview with him where he was saying the biggest data quality that he saw and the biggest leverage for data was always when the consumer and the producer of the data were the same person.
Which was the case for things like the messaging team at Slack or things like that. But for growth teams, I think the case where that could support your theory about growth engineers might be the first engineers to really get this thing, I think it's because they work in that sort of second tier that Erik was referring to.
They're in a single team where they have a really shared strategy with the product manager and probably there's an analyst there and so they're super close to the data, and so I think that might be the reason why they could be a good candidate. Is that what you see?
Emilie: Yeah. I think too that a lot of times, it feels like that's the first time you're trying to tie individual interactions into chained events, into sequential interactions. What I mean by that is the whole product org will have DAUs and MAUs, the whole product org will care about how many people have accounts and how many new signups. But being able to say, "This user who we gave a special treatment to then went on to convert and pay us more money than this user who was in the control," that's where getting your product analytics right is so much more important and so much more impactful.
It feels like the orgs need a why before they invest in product analytics, and I have seen too many growth functions spin their wheels trying to get off the ground, where they're like, "We're going to run an experiment," and then they realize they don't have any of the instrumentation in place they need to be able to run that experiment. So it's a chicken and egg problem where then you decide, "We're going to build this airplane while we're flying it," and just continually have that.
In my experience that seems driven by growth engineers, but every company has got their own story. I really like the way you framed it though, around where the producers and the consumers of the data are the same because I think that is what maps onto that growth engineer story is that they are looking to see and measure the impact of whatever they're shipping, the feel as accountable to it as the product manager. That's why they have this moment of epiphany where they realize the value of product analytics, it's because now they can think about their work and measure the impact of that work in a new way.
Stefania: Yeah. I couldn't agree more. Because another thing, it's like, okay, as a data person you are asked to answer the question, "What was the impact of this product change?" And early in the maturity stage of an organization, then when you get that question you might not have had any input or knowledge even that that thing was actually going to be released.
So you get that question when things are out the door already and you get a ping from the CEO or something and they're like, "How did that go?" And you're like, "How did what go? What?" And so I think that's a super interesting thing, and the next step in that is just try to inject yourself a little bit earlier in the process and then you start to think about experimentation and how can I quantitatively say that this actually is better than whatever it was before.
What I really enjoyed doing at Quizup and we did this in the same capacity as you built with the Insight Time where we built the flywheel around this. We started off with an engineer that was dead curious already, a little bit at least, he was sometimes sending me charts and asking me questions and things like that.
Then pulling that person into thinking about preparing a product release and asking that person, that engineer, the question, "What do you think is going to be the success definition? What is the goal here? How would we measure the success of this? If we looked at a chart and it would go down or up by this much, would you say that this was a success or a failure? Would you be able and feel comfortable to say that?"
Then sometimes they would say. "No, because it could've been because of a seasonality or it could've been because of a marketing campaign or it could've been because of various different things."That's when they would actually come up with like, "Why don't we actually release this as an A-B test." Getting that initiative and thinking from the engineer I think was one of the most impactful things we did at Quizup, to actually start that experimentation culture as a part of the DNA.
Because otherwise it's always someone begging the developer to actually build two versions of their codebase and maintain two versions of a feature for a while when they don't really necessarily have desire or a reason to do that, so I think that was really impactful. Can you talk a little bit about your perspective on this?
Emilie: Yeah. What you said to me was, "We went and we found our business partner." Whether you start with finding an engineer or a product manager, or whomever it might be, a marketer who's running experiments on the marketing site. What it comes down to is finding someone who's as invested in the results as the data person is. Oftentimes they're more invested, they just don't have the ability to write the queries themselves.
Finding that person who can be your partner, and I use that word very specifically because too often, even where data people maybe aren't thought of as just number fetchers, they still just deliver numbers right. "Here is the results of that analysis you asked." And what we need to be doing is delivering recommendations, and partners are open to recommendations whereas stakeholders more often are just looking for you to deliver analyses or numbers.
Stefania: Yeah, I like that perspective. I'm going to quote you on that. Finding that partner and finding that early candidate for starting to build your flywheels for whatever culture you're trying to build I think is super important.
Emilie: I remember telling someone once, a conversation similar to this, "Pick one part of the business, serve them really well, make sure they're a partner, blah, blah, blah." And he replied by telling me a horror story where he had done exactly that, they were starting to gain some traction, the VP of product was on his side, and then the VP of product switched jobs and he felt like he had to go back to square zero.
Sometimes it happens. It was rough. But it's still, I think, the most effective way to go about it, certainly not foolproof and that's why I mention the story. But it is much better than trying to do a bunch of things mediocrely, it's to do one thing or few things very well.
Stefania: Ah, the philosophy of data cultures. Okay, awesome. I mean, currently you are a data strategist so I'll leave it up to you for whether the org structure there is interesting. But ultimately, I think probably your experience with Netlify and GitLab and all of the data centric roles within product organizations that you have been in, I would love to hear your thoughts on org structures, data-wise, what you've seen, how data works with product and engineering. We've already touched a lot on this, but integrated with your product teams or separate, and things like that.
Emilie: All of the above. Is that a good answer?
Stefania: I think that's a classic answer.
Emilie: Yeah, every business is different. I have some rules about how I like to think about it, but that doesn't mean that there's not an exception and so I start with that caveat. Right now, my role at Amplify, I get to work with multiple organizations, see how they're thinking about data and I see different companies structuring their data orgs in different ways. But what I keep coming back to, and the model that seems most effective and most empowering is still that centrally reporting, locally prioritized where you report into the data org.
Now, does the data org report into finance or product or ops or something else? It depends a lot on your business. How naturally data driven is your executive team? Or where are your biggest stakeholders? And who has the most questions? Where is the funding? What kind of a business are you? Right? A SaaS company?
Which is where I've spent the bulk of my career, it has a very different org structure than a direct to consumer eCommerce company, and so understanding the nuances of your business are important when you think about where the data organization should live across the company.
But I also suggest that people understand a little bit around very standard accounting practices and what it means to be in R&D versus a part of the sales org versus GNA, and what the implications of those are and how industry benchmarks might affect the funding and headcount that your team does or doesn't get.
If I had to make a recommendation for a SaaS data team, my recommendation is that they report into the engineering organization, all of the data team members report into the data organization and then folks have workstreams or business partners across the part of the business where they're going to be spending 60 to 80% of their time.
Stefania: Yeah. And then build the relationships for the other functions of the organization from there, basically.
Emilie: Mm-hmm (affirmative).
Stefania: I think this is a journey, like you said, all of the above. I think this is a journey that most organizations go through, they switch between these data structures, the team structures throughout the company lifecycle. It depends on the size of the organization and then you scale to the next level, and then you scale to the next level.
Emilie: Absolutely. There's also something about maturity there, where when I joined Netlify we were starting a data team from essentially scratch. And so the first few hires were all analytics engineers, we had one data engineer, a couple of analytics engineers. And then as our data stack became more mature it was possible to do more analysis without making changes to our core DBT models.
Then we were willing to branch into data analysts, and so the folks who ended up being locally prioritized to product were data analysts, whereas the person who was locally prioritized to finance was an analytics engineer. Those are super different roles, but they were a reflection of different needs of the business at the point in time when they were hired, and the different needs of those functions.
We could not have brought in and successfully created opportunity for those two data analysts if we didn't already have an analytics engineer who had spent so much time building out our core product models.
Stefania: Yeah. I think this is the perfect segue into what I knew I wanted to talk to you about on this episode, which we just have to touch on. I think we have some shared visions on titles and roles. You gave a fantastic talk at DBT's Coalesce-
Emilie: Thank you.
Stefania: ... last December. Thank you for shouting that out. The title of the talk was Down With Data Science, and where you were arguing for whether that title is helpful at all, basically. I have strong opinions there as well, but I want to just maybe give you the word quickly to... Can you shed some light on that thought, Down With Data Science?
Emilie: So there's two parts to this. The first is what kind of work are most data teams doing? And when we think about science, the definition of science, it really comes to experimentation and proving out hypotheses. Sometimes that's what data teams do, but it's not the only thing that data teams do, and so a job title that reflects a teeny slice of your job probably isn't the best job title. So that's number one.
Number two is we need to make sure that our titles are allowing for people to have careers in data, and not just careers in data like 20 years of having the word data in your job title, but careers in strategic data teams that are going to allow them to be impactful. Right now what we're seeing is, especially for people who came to the modern data stack early, they're five or six years in and they're like, "Okay. Now what do I do next?" Because they've tapped out.
There's no real roles out there for Principal Analytics Engineer or a Principal Data Analyst or a Staff Data Analyst, so there's no career track. So we are taking our best people, these people who are incredibly impactful to our business and they're moving into finance and operations and all of these other things, and we cannot have successful 20 year careers if we don't create opportunities for people to have successful 20 year careers.
So starting with strong job titles can allow for strong career opportunities and creating that vision for data longer term. Part of where this came from was I was at GitLab in that point in a startup's life where people don't love to talk about it, but you have to bring in the adults who have done it before. You've stretched your people who you took a chance on long enough and it's about time.
So we brought in this senior director of data who had a ton of experience and the data team very quickly, what he did was what a lot of people do, is he ran that playbook that he had run in his previous role, building a data team that was very much around this IT service model of, "We're going to build our data platform and you can have your data analysts in lots of other parts of the org. We're not going to be responsible for centralizing things."
I look at that and I'm like, "That is not the career I want. I don't want to reach that VP level role and think to myself that the data team now has to be in an IT service model because that's the only thing that I've ever seen." So how do we take the strategic data orgs which were growing in these early stages, that we're seeing impact the business and how do we scale that? What do we do to take our best data people and help them scale their careers to help us build these strategic data orgs at the next level of a company?
Stefania: Okay. So there's a lot to unpack here and these are great questions. That's the starting question. Obviously ideally we can get some answers here, but I want to just highlight what you're saying here with the career trajectory of a data role. It brings us full circle to two areas that we've been covering on the episode so far.
The first reference really is too early in the episode when we were talking about how do you find people for data roles, and typically you have to hunt for junior people that are hungry and it's really difficult to estimate whether they are the right fit because they have little to no experience already. So you have to make a bet on other aspects because when you've done a data role and when you've built a data culture from scratch or early or been a part of that journey once or twice, you want something else next.
This is such a good highlight, those folks are moving into other areas, a lot of people maybe into maybe product or growth I would say also. Then I think the other full circle that's happening here is the reference you made to that there are a lot of people building incredible data tools right now, and I think this is probably input into that.
Their option was do the same thing again at another company or do something with really high leverage and build a product to solve a problem at scale for many, many, many organizations, which is exactly what we did at Avo. I just wanted to highlight that.
Emilie: Yeah. I raise my hand here because when we talked about my own career story, I told you about the year I spent in an operations role, right? Data was my hammer, but not what I did day in and day out, and I think that is the thing that really helped me connect the dots in my own head, was that I was gaining traction in my career and I saw how side stepping allowed me to be impactful to the business.
So when I moved back into data, I built a team where it wasn't a side step to impact the business, it was your job to impact the business. Now at Amplify, I'm doing this with multiple teams and we're seeing similar effects where being proactive, pushing out insights, making sure that you're seen as a partnership, having workstreams that people are focused on, centrally reporting but locally prioritized, is the kinds of things that are moving the needle on how people are building data teams.
But then the question remains, so what next? Not just for me, but for all of these other data leaders. How do we allow people to continue to grow? I look at a bunch of my fellow data peers and I see them move into product, like you're talking about, especially at data tools companies. So many people moving into product management at data tools companies, and on the one hand I'm really excited because, yes, please take all of the frustrations that we have had as data professionals and go fix the tooling, for goodness sake.
But the other side of that is also a sense of sadness, that the reality in place is that there is no places for people to really grow. You can go run the playbook again and maybe that's interesting one or two times, but then what? Some people will never want to grow, some people don't want to be VP of data and that's totally cool.
So we need to create the same sort of opportunities that we've had for software engineers to go this principal-technical track for data folks at all levels. There's that great post by Ben Stencil where he talks about the Chief Analytics Officer as your IC data analyst who's working for the executives. I said earlier that when you talk about data with executives, you need to just accept that it's a service model, right?
Does that mean that every data team should have a chief analytics officer who's just focused on surfacing the executives? Maybe. Maybe that is the career ladder that we lay out for everyone. I don't know that your director of data is going to get the clout in your organization to give away a C level title like that, but it is certainly something we have to think about when we think about not just five, six year careers in the modern data stack, but 10, 15 and 20.
Stefania: This is so interesting. A lot to unpack there, but I wanted to at least touch on this one thing that you mentioned. Okay, you had the opportunity to be impactful as chief of staff and now when you joined Netlify you were not going to do that tangentially, you were actually going to build a team that was impactful.
When you joined Netlify, what was the role positioned like? How big was the organization when you joined? Were you headhunted directly by Chris and the founders? Or how did that happen? How much of this did you position upfront versus build it as you went? Because it sounds like you already had a strong opinion that you wanted to do this this way, even before you joined the role.
Emilie: So I was recruited to Netlify by the former VP of engineering, Dalia Havens, who's an incredible leader, who I had the honor and pleasure of working with at GitLab as well. In a lot of ways, I went to Netlify to work for and with her. I was reporting into her and my mandate was to lead data.
I was starting from scratch-ish, and by that, Netlify had hired a PHD who was very good at very complex statistics but did not know anything about the modern data stack. And so all of their pipelines, getting data out systems were custom built and they would break a lot, and so there wasn't a lot of net new work because they were maintaining lots of things and there were things written in Scala and R, and nobody peer reviewed anything.
So I had opinions on how we were going to do things, but I also did what I would recommend to any leader, which is you do nothing for the first 90 days. Maybe that's like 60 in startup land or 35. But you have to come into a job and just take in what's going on, and there is no amount of context you get during the interview process that tells you what the right answers are.
You just have to start and then start drawing conclusions. There's the great book called The First 90 Days about starting your new job, but when people come in and they say, "This is what we're going to do, we're going to implement this data stack and we're going to change these things," that's actually a huge flag to me because it means that they're not listening to the context and they're not forming the partnerships they need to draw the conclusions.
But at the same time, you can have an understanding of your mandate, you can have a vision for the team, but knowing that you're going to have to figure out what the context you're operating in and how to apply the things you understand about the role to that specific context I think are important.
Stefania: Yeah. That's a really great observation, do nothing for the first 90 days. But it sounds like you had really strong buy in and here it comes again, the power of relationship building. You had previously built a relationship with this person and so there was trust there, mutual trust for you to do your thing and-
Emilie: The thing that I'll add there too is I think of interviewing like dating, so it's just as important that Netlify find the right data leader for them as it was that I find the right role for me. And so I asked for more interviews in the process, because I wanted to talk to more people, I wanted to get a better understanding, I wanted to understand more about the culture. So I think it's really important to come into it from that perspective as well, that you are not the only person interviewing here, you should also make the company interview for you.
Stefania: Yeah, I totally agree with that. We also have that as a part of the Avo hiring process, so there is definitely room for reverse interviewing. I think that's really important, and also ask someone that is hiring, you do learn a lot also from reverse interviews because you learn a lot from questions that people ask.
Emilie: Absolutely.
Stefania: I want to just throw this in here, you reference data professionals a lot, and I shared this with you before today's session. We, at Avo, we're currently recruiting for a role that would in many companies be called developer relations, but in our case it's not relationship building with product engineers or software engineers or developers in that sense, and developers, okay, yeah, we could understand or say that data people... they're developing something, maybe they're developers.
For example, DBT has a role referring to developer relations while most of their relations are built with data professionals, analytics engineers, data engineers, data analysts, et cetera. So this was something, and I threw this literally out to Twitter, what should we call this? Should we call it community relations building? Because there is no single reference to a role that is as encompassing as developer. Do you have a hot take there? What should we replace data science with?
Emilie: I will say I don't envy your position, this is a hard problem and I think part of it is you're going to post a job and hopefully find your candidate. But what I would recommend is being open to helping the candidate tell you what the right title for the job is, and what I mean by that is maybe they want to be analytics engineer relations or product business partner relations or whatever it might be. It is a catch-22 where the title attracts the candidate, and I think you make up for it by having a really strong responsibilities and requirements section and outlining what the role is and doing a lot of great job sourcing.
I think the best way to find a strong candidate is to go find them instead of waiting for them to come to you.
But then also being open to asking them what's a better way to articulate the value prop of this role through your job title? But that all being said, it is a hard problem and I don't think there's a clear answer, but my guess is in the next short term future, we're going to see some industry standardization on what a good title for devrail and data looks like.
Stefania: That's interesting. Yeah, so specifically around devrail and data. Yeah, I think that's really interesting. I am excited about that. The take away basically of the title talk, Down With Data Science, or one of them, you talked about two really important reasons why titles matter, early here in this session, the title actually represents what you do so that's helpful for you to understand what actually is your role.
Then the other one is titles should help you develop your career. I think what you also highlighted in the session is data science is too broad and we need to be more specific about the titles instead of using a generic data science title.
Emilie: Yeah, it's to unspecific and unmeaningful. I would interview candidates with the data scientist job title, and the first question is like, "So what did you do? Were you writing algorithms or were you writing SQL queries?" And never did I ever get pushback on that question because all of them would tell you, "Yeah, I know my title is ambiguous."
So the blog post that I point to is that blog post from Lyft where they're like, "We're rebranding data analyst to data scientist because branding." It is such a sad thing to me that we would choose that branding and the ambiguity that comes with it in favor of the stronger careers and the stronger communication that comes from a clear, more direct title.
Stefania: Yeah. This relates a little bit to a conversation that I had with Maud Church about the caste system also of data titles, it's really interesting. But we are approaching an end here. I definitely want to end by asking you for recommendations for what is the first thing teams should do to get their analytics right and what is one thing you wish more people knew about data and product?
But before we do, I would also love as like an input into the locally prioritized, centrally reporting, can we talk a little bit about... Because we talked a lot about product analytics earlier and experimentation, and you had shared some thoughts on what should that process look like, who should be involved in the planning process for feature releases?
Now we're getting in the nitty gritty, so we're not talking about high level, we're talking about nitty gritty, planning it, implementing it, QA-ing it, prioritizing future work based on data and all that stuff.
Emilie: I think what it really comes down to is starting with that partnership relationship. People never love to talk about ratios, but we should think about it in terms of ratios. If the right ratio for your organization is half a data analyst per product manager, depending on the domain and the demands you put on them, then that's it.
But you need to make sure that that product manager and data person, whether it's a data analyst, an analytics engineer or something else, is someone who is working very closely at every step along the way to make sure you are capturing telemetry as part of early release and that there's some standardization across the organization.
And so that has to be a two way street because data cannot just ask for those things, it's almost like then there'll be a nag to the product org and product can't just ask and then say the numbers don't look right. They need to feel ownership over how those numbers are getting produced. I think it's not just data and product, but it's also engineering.
We can't just build specs for engineers and expect them to go implement it. We also need to make sure, like we were talking about earlier, they understand the impact of that and why those are important and how we can get them in on this partnership as well. So it really comes down to feeling that relationship, and iterating until the process feels right for the both of you.
Stefania: Yeah, I love that. Thanks. Let's start wrapping up. What do you think is the first thing teams should do to get their analytics right?
Emilie: If you're starting from scratch, I always think of a talk I gave at the Modern Data Stack Conference in 2021 and I talk about the five types of work that your data team does, and operational analytics is always the one I think can unlock the most because that's how you take data that only the data team has and you spread it throughout your organization in the tooling that people are most comfortable with.
And so putting product data in Salesforce where the account managers and the customer success people can play with it and feel empowered with it in a new way, or giving marketers new pieces of product data that they can trigger analytics on, or trigger messaging and campaigns and things like that.
Giving support information about the last email that the person opened and just this cross pollination of information that today the data team has but the rest of the organization doesn't have, that is a real way for people to feel the impact of data in their day to day work.
Stefania: Awesome. I think that's a really good recommendation, also because it starts immediately building those relationships and buy in and getting you sold as an ally to those other teams. What is one thing you wish more people knew about data and product or just data in general?
Emilie: It's hard but so fun. Maybe everyone knows that, but I love it. I joked for a long time that data was both my job and my hobby, and then I had a kid and I was like, "Haha, no more time for hobbies." But I think I look at communities like Locally Optimistic, which is a community of data professionals where I am one of the admins, that is a labor of love if there ever was one.
All of the admins are people who just really like data and they don't log off at 5:00 PM and turn their brains off. They are thinking about how do we build better careers and how do we make our tooling better and what's the next steps and looking forward. So if I only had one thing to say it's that data is hard, but so fun.
Stefania: I love that takeaway. Let's have that be the last words of this episode. Emilie, thank you so much for joining us on The Right Track.
Emilie: Thank you for having me.
Subscribe to Heavybit Updates
Subscribe for regular updates about our developer-first content and events, job openings, and advisory opportunities.
Content from the Library
The Right Track Ep. #11, Defining North Star Metrics with Che Sharma of Eppo
In episode 11 of The Right Track, Stef Olafsdottir speaks with Che Sharma, CEO and Founder of Eppo. This conversation includes...
The Right Track Ep. #10, Getting to Know Your Users with Boris Jabes of Census
In episode 10 of The Right Track, Stef speaks with Boris Jabes, CEO and Co-Founder of Census. They discuss the impact of SaaS on...
The Right Track Ep. #8, Defining the Data Scientist with Josh Wills of WeaveGrid
In episode 8 of The Right Track, Stef speaks with Josh Wills of WeaveGrid. They address common misconceptions about data and...