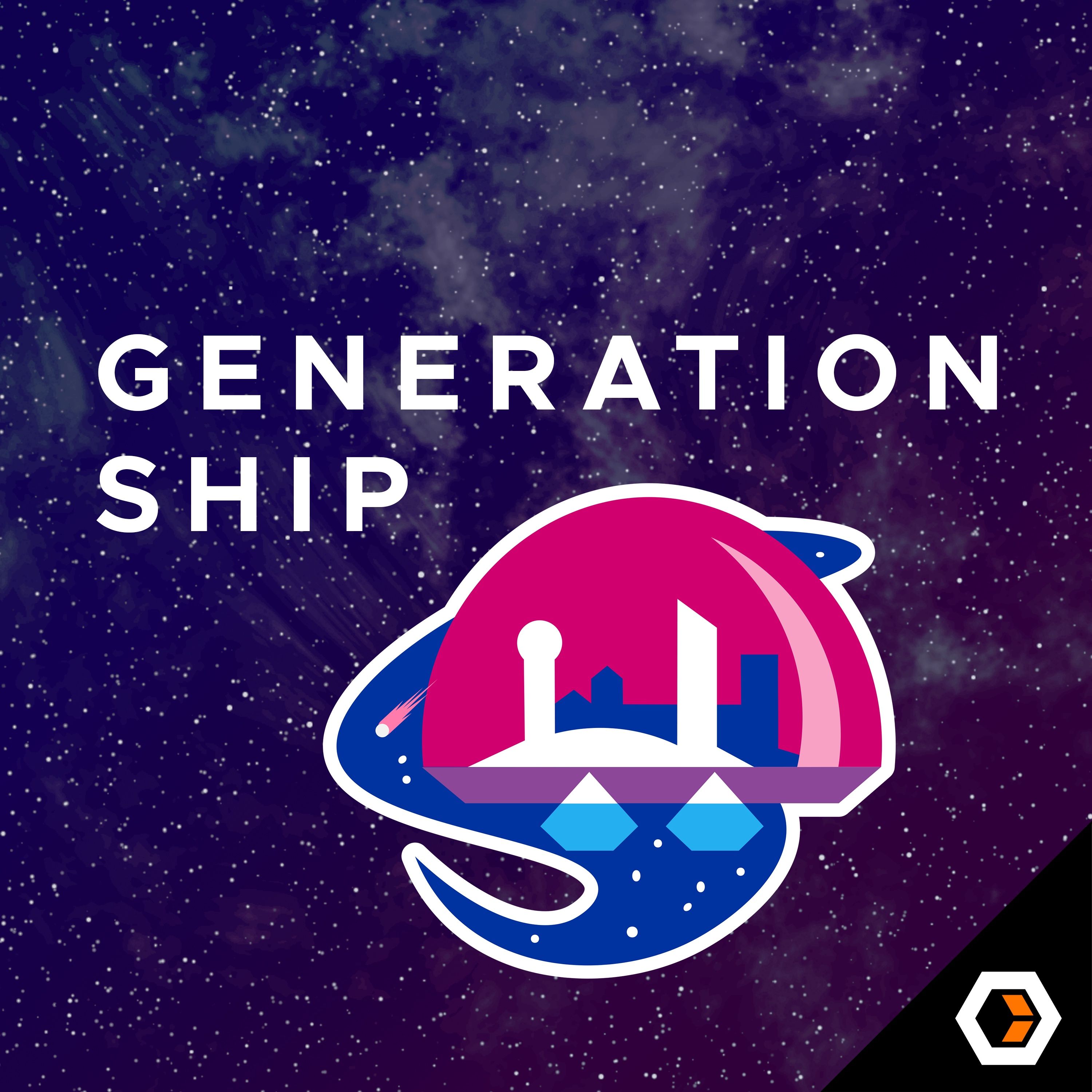
Ep. #9, How We Will Work Together with Michelle Yi
In episode 9 of Generationship, Rachel Chalmers speaks with technology expert Michelle Yi. This conversation examines how generative AI will change the future of work. Together they explore topics like the philosophy of work and productivity, the advent of multi-agent systems, and what to consider when investing in AI.
Michelle Yi specializes in machine learning and cloud computing and has 15 years of experience in technology consulting. She serves on multiple advisory boards and is affiliated with Basis Research Institute, a nonprofit startup AI lab that specializes in generalized reasoning. Michelle's passionate about diversity, STEM education, and careers for minority communities and is an avid volunteer with Girls Who Code.
- AI Act
- Collective intelligence for deep learning: A survey of recent developments
- Yann Lecun: Meta AI, Open Source, Limits of LLMs, AGI & the Future of AI
- Covariant
- How Infrastructure Works by Deb Chachra
- Timnit Gebru
- US health department opens probe into UnitedHealth hack
- Distributed AI Research Institute
- Nightshade: Data Poisoning to Fight Generative AI with Ben Zhao
In episode 9 of Generationship, Rachel Chalmers speaks with technology expert Michelle Yi. This conversation examines how generative AI will change the future of work. Together they explore topics like the philosophy of work and productivity, the advent of multi-agent systems, and what to consider when investing in AI.
transcript
Rachel Chalmers: Today I am thrilled to have my good friend, Michelle Yi on the show. Michelle specializes in machine learning and cloud computing and has 15 years of experience in technology consulting. She serves on multiple advisory boards and is affiliated with Basis Research Institute, a nonprofit startup AI lab that specializes in generalized reasoning.
Michelle's passionate about diversity, STEM education and careers for our minority communities and is an avid volunteer with Girls Who Code. She enjoys building teams dedicated to leveraging cutting edge technologies and techniques, AI, machine learning, cloud platforms to tackle some of the most difficult business and societal challenges.
Some fun facts about Michelle, she speaks six languages. She went to university at the age of 13. She has played for the New York Philharmonic. She was a finalist for the Women in Technology, Women of the Year Award in the science category, and she enjoys fast cars and motorcycles.
I always think, did you ever see that series of ads for Dos Equis beer, which had the most interesting man in the world? I think you may be the most interesting person of any gender in the world, Michelle. So thank you for coming on the show.
Michelle Yi: Thanks so much for having me Rachel. I'm excited to be here.
Rachel: Obviously we're here to talk about generative AI, that's why we call the show Generationship. How do you think generative AI or any other kind of machine learning is going to change the way say software is developed and deployed?
Michelle: Man, so much has happened in the last year alone. It's been such a crazy journey and I'm excited about it. You know, after a long period where it seemed like AI wasn't getting that much traction or it was like really struggling to make it into production and now it's so exciting to see all these developments. I'm in particular excited at not just like the large language models, but also the rise in multi-agent systems in particular.
Rachel: Yes.
Michelle: And I think that's just going to drive so much, not just automation, but like it's really going to change the way we do deployments, but also like coding in general, especially in combination with all these other technologies that we're developing. So in the future, I don't know, I think our jobs are going to get harder, like.
Rachel: Oh no, that's the opposite of what everyone's saying.
Michelle: Because well not, and maybe it's not necessarily like in the quantity of hours that we're like, that might become less, but I feel like we're going to need actually even more critical thinking, right?
And even as you're interacting with like LLMs and things like that, you do need to think critically. Like, okay, am I using this as a crutch? Could it be hallucinating? Do I need to double check that? And I mean like, sure, a lot of tasks that don't require that much energy, maybe they will get automated. Like I'm not going to copy paste stuff from Excel to, I don't know, some document. But in general, I feel like the problems that we will have to spend our energy and focus on will be the harder problems like architecture or like these maybe niche or like outlier use cases and things like that.
Because at the end of the day, like the current state of LLMs and AI and agents, they kind of like solve for a median or like an average, right? And so anything outside of that distribution is where we have to put our energy. And I feel like that's just like the hard problem. So I don't know if it's solving anything for us, but that's what I feel like we're going to, how it's going to change like maybe fewer tedious tasks, like we'll get that time back, but then we have to really put our focus on like these bigger challenges in technology.
Rachel: That feels like a really powerful way of thinking about it. If you think of work output on a normal curve, because AI is tuned to the wide part of the bell curve, anything that's falling below that, AI can push it up till it's at median standard. But anything that we're doing that's on the high end of the bell curve, that's on the long tail, that's like of higher achievement. AI is going to drag you down. It's going to give you the median result.
So to say back what you just said, we're going to refocus on the sort of the harder part of our job, but also the part of our job that's more creative, more meaningful, more nuanced, more human. We can automate the stuff that, you know, give it to a computer and that'll bring it up to the mid standard of what humans can do. That means that humans have to specialize in the high end of the bell curve.
Michelle: Yeah, absolutely. You're spot on and like, you know, it's like back in the day we'd have to manually spin up VMs and kind of like hand manage or like allocate memory or like do all these other things and even that's progressed. And so now I just see this as the next evolution and it's not the case that our jobs got any easier. I think we just had harder and harder tasks.
Rachel: And that opens a whole bunch of questions about what is ease in work and what is toil and what is productivity, you know, because I'm back working in venture really, you probably only make one decision a year that meaningfully affects the outcome of a fund, but you spend the rest of your time making sure that you have enough information and enough muscle memory that when you make that decision you've got a better than 50% chance of getting it right. I think more jobs are going to be like that.
Michelle: Yeah.
Rachel: We'll just be rehearsing to make one good decision here.
Michelle: No, exactly. And I actually, that is a concern I have is like how are we going to measure productivity? Like we're already productivity obsessed as a culture. Especially in the bay. But like I just think back in COVID, like one company that I was collaborating with during that time, you know, they were measuring developer productivity as just like total GitHub check-ins, right?
Rachel: Lines of code.
Michelle: Yeah. Which you can have tons of lines of code but you know, it doesn't give justice to the person or you know, whoever is solving a, I don't know, maybe it's only a 10, you know, lines of code problem, but like it was a really difficult thing to narrow down.
Rachel: Yeah, it's like when you're in college and you've got a six page essay that you have to hand in, so you put everything in 14 point and have a massive margin around it.
Michelle: I never did that, definitely never.
Rachel: Of course not, of course not. But is that part of our job harder or is it hard also just to like manually go through one at a time and fire up instances and connect the dots to make sure they're all secure and all of the ports that should be closed are closed? I mean that's hard in a different way. It's repetitive and it's easy to make mistakes because you're not being intellectually challenged.
Michelle: That's true. But are those the problems that we really want to be spending our time on anyway? And I guess it's a valid question, right? Like maybe there are certain aspects of that that we do, but in general, like I think we do want to have kind of like this maybe higher level of abstraction or like a role at a higher level of abstraction than maybe being too much in sort of like the detailed hands on management of things.
So maybe in the future our productivity looks more like a different level of abstraction where we're managing things at a bigger scale but with agents helping us or things like that.
Rachel: Yeah, I think that's what it starts to look like where you have, as you were saying, the multi-agent world and you've got you know, little pieces of code program to do little pieces of your job and then you have to sit back and decide judiciously whether or not they've done a good job. But that's sort of getting us to the equivalent of the work that we're doing today.
And I'm wondering what does the work we're doing tomorrow look like? Does it still mean like we're maximizing profits to manufacture a small group of white billionaires or does it mean we're working back from let's try and make the planet sustainable for everybody. Let's try to get everybody fed, let's try to get everybody housed. Crazy stuff like that.
Michelle: I hope those are the hard problems that we're solving for in the future and not like making niche companies or like solving for small groups or specific groups of people. But what you point out is also like if you frame it one way, this question of sustainability or even like the demographic piece of it, those could also be seen in a way as out of distribution problems because I mean, now it's gaining traction that people care a little bit more, maybe I don't know about sustainability and things like that.
I hope so, although sometimes I'm in doubt or like, you know, gender parity and all these things. But those are also kind of in theory like out of distribution and that the majority, like the main chunk of maybe society or like people contributing in the technology space aren't necessarily focused on, they are focused on maximizing profit for X reason. Sometimes it can be for good but yeah.
Rachel: Are you worried at all about the risks of widespread use of commercial LLMs? Are we baking algorithmic bias into our systems?
Michelle: In general, I'm a technology optimist in general.
I do think there's a lot of lift that LLMs and other AI technologies can give us, but I'm also absolutely worried about the bias. And again, these are like the hard underlying problems that I don't know that people really want to fix, right?
So in terms of like bias and I think, I don't know if you saw that EU regulation Act finally got, AI Act finally got passed officially. And I think they have some really valid kind of restrictions or stipulations there like around critical infrastructure and the use of AI and anything deemed critical or high risk that includes like energy or like healthcare and criminal justice.
And then they also have like some transparency regulations around like, hey, if you develop an LLM, like you need to provide metadata of the trained model, right? And so like we don't have that visibility today. And so there's definitely biases hidden in there.
Like one thing, one crazy thing that stood out to me was like there's a really common computer vision data set called LAION and over the span of like three years and maybe five papers, it was identified that there was like bad images, cp, which you know, we won't really say this but, so there was like cp and there's some really bad images of vulnerable populations in this dataset that's public and widely used to trained image models and like three years, five papers, people pointed this out and nothing was done.
And so like even if you use Stable Diffusion models today, like actually those are trained on this dataset too. But instead of like solving for the training data and like the biases that we ingested from, you know, the goodness of the hearts of people on the internet, like even our techniques for solving or like mitigating this bias is sort of like a layer on top of that. So like alignment and red teaming and all of these techniques, which I still believe are good and we need them and are useful.
Rachel: But they're reactive rather than proactive.
Michelle: Exactly, there are things that are on top and there are things that like, oh red teaming for example, like I need to expose manually, right? The biases that the LLM produces, right?
And so like I think one of the words that maybe it was OpenAI or Microsoft recently banned was like abortion. Because anytime you ask it to generate something about abortion it's like horrendous results. It was really bad. And so they actually just outright banned the word or like saying like, hey this kind of violates our terms of service or like use and so like again, we're just, we're solving for these reactively and whereas the underlying problem is already baked into the model.
Rachel: So a couple of really interesting things there I think, I think the reactive approach to how we're managing these flawed data sets still reflects the profit motive. Like how can we build a business around correcting for the flaws in the underlying data sets when the real challenge to build new data sets that more accurately reflect what we'd like to imagine are our values would be very expensive. It would be a cost center rather than a profit center. And so there's little incentive to build that.
And then going back to the fact that Europe has once again led the way in legislation that's, you know, tuned into the risks of technology, this long history of Europe and America being in this interesting dance where America is more freewheeling and produces a lot of innovation and some of that innovation is potentially damaging and Europe is able to take a step back and think about the consequences and introduce legislation like the GDPR, which is able to manage some of the harms, which makes it sound like I think Europe is the good guy. I actually think that Europe's in a position to do that because it's very wealthy and has very fortified borders and has a relatively homogeneous population.
And so it's reducing harms for a low power distance society, which is only possible because of the high power distance between Europe and the rest of the world. A long-winded way of saying, don't you think it's interesting the ways in which our approaches to the AI reflect long, long histories of how we impose and define both power and harm in those respective geographies?
Michelle: That's a really interesting way to frame it. And you're absolutely right. The incentives just aren't there. Like at least I can't speak to much in Europe, but like there's not really the incentive like imagine if OpenAI or you know, any of these companies I'm only, I was just picking OpenAI, they happen to be top of mind 'cause I was reading about them, but if any of these companies, right, like had to retrain from scratch to account for these, I mean that also would cost a significant amount of money and effort.
Rachel: Money and time and water and fossil fuels, energy investment of resources into these things is enormous.
Michelle: Yeah, like what's the incentive for doing that? Like providing more equitable results like, right? Whereas like oh we can provide equitable results by kind of glossing over like, I shouldn't say glossing over because these research areas do take a lot of effort but instead of solving the actual underlying problem, like we can sort of mimic or like get around some of these bigger issues. I don't know if you remember back in the day too, like when facial recognition just came out and like the gorilla incident where.
Rachel: Yes, yes, hard to forget that one.
Michelle: With Google by the way, so I can pick on somebody else that's not Microsoft or OpenAI. And so yeah, they identified like one software developer and his partner were identified as gorillas and then the solution was to remove the label at the time. I don't know if they actually created like a better solution for the underlying problem.
Rachel: I'm going to take a wild guess.
Michelle: I have no idea. But it's sort of similar here too, right? And so yeah, I am really worried that we just reinforce these stereotypes and we don't have a good solve for fixing the underlying incentive structure.
Rachel: Yeah, I mean I think it's innately human to love ease and to love tools and to love novelty and things that we can play with and things that we can use to do what would otherwise be onerous jobs. I don't think it's as innate in us to think about the broad implications for people that we don't know.
And I think one of the challenges as we go forward is to try and educate ourselves into this larger awareness. And again, this is why I love talking about Generationship, this larger awareness that we share a finite space ship filled with finite resources with a community of other people. And that's hard to do. It's not how we're wired to think and it takes an effort.
Michelle: Yeah, and I guess the other question too and probably more in the VC space than on the startup side is sort of like how can we invest in the technologies that can actually impact like sort of mitigating these risks? And so like that's one reason actually I'm interested in sort of like the work that for example like Basis Research does around generalized reasoning and their approach involves also like causal approaches and so it's more explainable and like there's other methods basically and research areas that could be used in conjunction with LLMs and like these other foundation models. And so I have hope that there's other areas of opportunity to mitigate this, but we still have to incentivize and fund like that type of work and how do you make that profitable?
Rachel: I think that's part of risk mitigation, but starting with the technical, tell us about some of the approaches that Basis is working on that you think are really promising.
Michelle: So what stands out to their approach to me anyways, at least from my time so far is like this causal inference piece. Like actually being able to tie the, like not just a correlation but a causal relationship from one thing leading to another based off of like models of the world or models of what you know, I think that's really compelling to me because so much of what we have today in LLMs and stuff is quite spurious, like, you know.
Rachel: They're just guessing. They think they're doing their best, they're just guessing, they're saying what they think we want to hear.
Michelle: It's like there's, yeah, there's like bias by underrepresentation but there's also bias as it just from spurious correlations. Like dogs are always outside, that's not true. There's just more images of dogs outside.
Rachel: I got one right here.
Michelle: Yeah, see and still a dog by the way. So there's like, you know, and so like actually being able to explain and then reason over these things is really compelling. And then I guess the second thing I would point out is they also have a probabilistic approach or component to it, which you know, gives you more of a grounding than just, oh like for example if a model hallucinates, it's like, yes this is a, I don't know, a dog where it's actually a cat, but instead you would actually get a probability of like, hey I think it's 70% a dog and 30% a cat. And then you could understand why.
Rachel: And when you're using a model to, you know, generate a first guess and then you are going over and editing it, those weighted probabilities again take work out of you editing the whatever that the model has produced for you. So it does seem to get us a little bit further.
Michelle: Yeah, exactly.
Rachel: You can punter this question if you want 'cause you're not an investor yet, but when we think about that larger sense of incentivizing, motivating people to build technologies that are useful but less harmful, how do you think about that? What are some of the areas you might want to invest in hypothetically?
Michelle: So I do think this area is super interesting. There's a couple of startups that also do things in collective intelligence. So like Sakana and Basis both have this in their approaches. And I think that's also really interesting because it kind of is like group decision making and inspired by nature also. Like, so the original paper by David Ha, well one of his papers is like inspired by different animal behaviors and like how they conclude which direction to go and things like this.
And it's kind of interesting to take real life, basically these, I mean these animals kind of make, they're smarter I think, I don't know if you watched the Yann Lecun Lex Friedman interviews, three hours, just watch it on speed or just read the transcript. But like one of the points Yann Lecun makes is that like the average house cat is smarter than the AI model. So like the modern AI models including foundation models
Rachel: I think that's true of two of my cats, the third one.
Michelle: You're not sure?
Rachel: She's more of a potato.
Michelle: That's probably still smarter than modern AI models, I'm not going to lie because she's an efficient potato I'm assuming.
Rachel: She is, she's very good at it.
Michelle: But there's all kinds of decision making processes that your potato cat also makes that we don't understand, right. And it's much more complicated than the decision making process of like the foundation models that we have today.
So if I were investing in technologies that are kind of like looking at that next level, I would definitely go beyond LLMs. Like I think there's still plenty of opportunity, especially the application of LLMs to industry. But if you're looking more at like the core tech side of the house, I think there's really cool but also more explainable types of models that inherently have more guardrails than maybe some of the ones that are out today.
Rachel: We are definitely going to keep talking about that. What do you think will be some strategies for coexisting with AI in the workplace of the future? How will we continue to like justify our existence and pay our rent?
Michelle: Well our work is going to get harder, so.
Rachel: Yes, so we should get paid more for it. That sounds good.
Michelle: I completely agree. We should definitely all get paid more for our work because our work will only get harder and I think.
Rachel: And we're managing all of these agents, we should get like a managerial bonus.
Michelle: That's an interesting idea. And if the agent like starts to, I guess like lack in productivity or become inaccurate, is that our fault?
Rachel: What about if the agents start to unionize and demand fairer wages?
Michelle: Okay so that is actually something when I was thinking about this question, like I thought about because like, well first of all what is the workplace of the future? And like I saw, you know, there's a spinoff of, I think there are ex open AI employees but like basically there's a spinoff called Covariant which specializes in robotics. A researcher I like to follow, I've followed for a long time at Stanford, Chelsea Finn, she is co-founding a robotics startup called Pi, like the policy Pi.
So I think like that's going to be a super interesting space and like how do we coexist with like AI in the physical world? Like that's a whole different thing. And then like even in the, I think in previous conversations and episodes like you had Mike Sawka on and okay now we're coexisting with them in the terminal.
And so like I think all of this to say one strategy I think we really need to also think about is, I mean not just automation or job security but also security in general like, like how are we going to think about for example, there's a paper that just came out where an agent based off of an LLM could independently hack websites and basically identify exploits and then use those vulnerabilities to extract information from how to like secure information from the websites. And so like I think that's an area we're really not well prepared for at all.
Rachel: I'm reading the amazing Deb Chachra's book, "How Infrastructure Works" and she's talking about large scale threats to infrastructure. It says, you know, human actors can like fire a high powered rifle into a transformer or they can hack into a water utility's website, but they're limited in what they can do because you know, even if you have quite a large terrorist movement, it's finite. But when those threats are multiplied by the ability of agents to carry out complex attacks, it becomes scary on the level of like a very large solar magnetic storm event. You could conceivably knock out the electrical grid for a continent and that does get frightening.
Michelle: Yeah and I think UHC actually, UnitedHealthcare just was compromised pretty severely from a security standpoint
Rachel: Oh well they don't have anything important about us, do they?
Michelle: Definitely not. I hope, I think I have UHC as my provider but, but you know, like, and I'm just imagining like being able to identify these exploits at scale as, and especially as we have like, well the first software engineer Devin came out and can independently code and so yeah, I guess like my main concern or like desire to have better strategic thinking for coexisting with AI in all these different environments is really like security and also like the human element.
Like how do we account for like our wellbeing? Because I also like for example, like there's some computer vision applications in like quick service restaurants where like it measures like you know, worker productivity and things like that. And it doesn't account for, okay, so let's say that somebody is making your burger if you eat burgers. I don't but like if for people who enjoy burgers or french fries, like someone is making them at like five seconds slower than normal average rate.
You know, what if they had a illness or some kind of like thing in their personal life that there's these things that like we can't really account for the human element that I think we need to have a better human-centered strategy in addition to security.
Rachel: I think it's pretty safe to say anyone who wants poor people to work harder has never worked a minimum wage customer service job, I have.
Michelle: I've done my time at McDonald's.
Rachel: Some people haven't and it shows. When gen AI started to become a really hot topic, I was kind of distracted and I hadn't been following the field for a couple of years and it really hit me on my curmudgeon side. It was right around when Web3 was starting to come unstuck and I dismissed it as another crypto phase until my partner said, no, you really need to pay attention to this.
How long do you think this will last? You know, we're clearly at the hype peak right now. God, I hope so. I hope it's not going to get louder than this. But what does the trough look like and what's going to be left when the wave recedes?
Michelle: Oh, well so I was actually recently reading like a venture report on this like, so people were saying that in January I think 2 billion in capital was deployed in AI startups and that's when people were saying that, oh the hype is dying down. Oh we only did 2 billion last year. We did like 50 billion capital deployed in 2023. And then this article said something like, oh, well in the first two and a half weeks of February, like 3 billion was deployed. So now we're, yeah, we're back.
Rachel: In January, VCs are usually skiing.
Michelle: Yeah, I was like, come on, what is this article. Like no, we're still in the hype and I still think like there's a lot left to go. Sorry Rachel, I still think there's, I still think there's a lot to go.
Rachel: I'm so tired Michelle.
Michelle: Also, especially in like narrow intelligence, I think there's still like so many applications that we haven't seen like in industry, like there's sort of these general foundation models and things like that but.
Rachel: Robotic process automation and all of those fields, just ripe for it.
Michelle: Yeah and like even just like in specific industries like finance or healthcare and infrastructure, even if we're going to go to more core tech, like I just think there's still so many startups that have yet to come. So I think we're still in the hype, but I also think that piggybacking off of the sort of gen AI hype is there's a ton of research in like different ancillary areas that are super interesting.
So like now we see the rise of video, we've talked about robotics and then like multimodal models, we still haven't even really truly hit the full potential of multimodal and yeah, there's just like so many other things that I think are just going to all explode as more and more capital goes into the space.
Rachel: What do you think will disappoint people? What do you think? I mean crypto disappointed people 'cause there's really no value creation there. I do think this is a little different. I do think there's real work that gets done by these models, but where do you think people are going to start to feel disillusioned?
Michelle: I know I was also pretty bummed about the like the Web3 and like crypto movement, but I guess to some extent people are disillusioned, but in general I think people are optimistic with like kind of like the gen AI movements, again because I can't say that it's really hit production that much, right? Like, so people are still interested, but I guess like the real challenge is going to be, especially this year, going into this year, probably more companies are going to be trying to actually productionalize, you know, gen AI models or like these different use cases.
As more and more hallucinations or like different challenges happen, I think the use cases are going to become narrower and narrower until the actual like underlying technology improves, which is sort of like a weird race. Like will the technology improve fast enough for production adoption and like, you know, to broaden the number of use cases that you can actually use it and not get sued. I think that's going to be the real question.
Rachel: And the number of AI startups I see looking at legal automation, you know, we are going to sue each other through a network of agents as well.
Michelle: Okay, but that being said, I did see a great presentation at Data Day Texas recently where this person built an agent that was like, it's around automating health insurance claims with an LLM and that is part of like gaming the system, right? I didn't actually know that you could save that much money filing a claim manually, but apparently that is like a really important thing to do.
I just trusted the system like a really naive person but, and so I'm like okay, maybe in the future like you will have these agents fighting your parking tickets for you and fighting the claims process for you. And those are in my mind very positive things because nobody wants to do that.
Rachel: I've got library books I need to read.
Michelle: Yeah, exactly. I'd rather do that than read the insurance forms.
Rachel: Speaking of books, what are some of your favorite sources for learning about AI?
Michelle: Anything published by, there's certain labs that I like to follow. I know you and I are both big fans of DAIR. So like you read a lot of the publications.
Rachel: That's the Distributed AI Research Lab, I believe is the acronym.
Michelle: Yes, founded by Timnit Gebru and there's also certain kind of like academic labs in general that I really like. So the University of Chicago has a great one by Ben Zhao, he's the creator of Nightshade, him and his lab and for example, they work with like artists and underrepresented communities to like basically help defend their copyrights or like their work. And so my go-tos are typically like these sorts of more social or neutral kind of nonprofits research think tanks.
And then of course there's sort of like the archive best papers and those listed at conferences, those are sort of my go-tos.
Rachel: Good to know, if everything goes the way you think it should for the next five years, what delightful utopia will we be living in Michelle world's 2030, almost.
Michelle: Everything goes the way I want it to. I think we will have solved all the bias issues in, yeah, probably longer than five years. But you said ideal, so I'm just going to throw this out there. In my ideal world, more than 2% of venture capital goes to female founded startups and maybe that's like 50% in five years.
I also want to see, now this is something that could reasonably happen within five years, you know, more AI startups and investment going into areas like education and social investments. So like there's a few startups I'm excited about in that space. But yeah, I think like there's a big potential for like increasing or democratizing access to education and quality education. Not just like garbage scraped from in, you know, like Reddit and stuff like this. So like real value.
Rachel: And that one's super personal for you. Do you want to talk about how you got access to education?
Michelle: Yeah, so when I was what, nine or 10 I basically, or actually younger, but then I was applying to college around 10.
Rachel: As one does.
Michelle: Yes. Around the age of 10 or 11 I started to research how to get into universities and get a scholarship and the internet was my savior so I would go to the public library, this is in South Korea and we had fantastic internet connections, it's free. For that time period, it was like a T1 landline speed connection, which is super fast for this time. And yeah, I just self-educated. YouTube wasn't really that popular. There was no Khan Academy, but I accessed books and I also had access to like PHP forums, which I don't know if anyone remembers those.
Rachel: Absolutely.
Michelle: But like, so I would just be like the nerd going on the math forms and asking people like kind of these dumb questions about calculus and all this stuff and yeah, I self-educated and I imagine like AI could do that for somebody like me in the future.
Rachel: Yeah, that's the dream. A library that you can talk to.
Michelle: Oh that would be so good. And you know, those books are really high quality. Probably no hallucinations if you use that kind of quality data, so yeah.
Rachel: Big finish. Michelle, if you had a colony shipped to the stars, what would you name it?
Michelle: First of all, I'm never coming back. I think it's a one-way ticket.
Rachel: There may not be horses in the stars. That's the only thing holding me back. Horses, dogs, cats, are we taking them with us?
Michelle: Yes, the answer is yes, I am going with Gestalt.
Rachel: Oh, I love that.
Michelle: Yeah, it is because it's always about the whole and not the individual parts and I like to think that our ideal future society looks that way at the bigger picture and doesn't judge us for these tiny components because we can get so narrow-minded sometimes.
Rachel: We're not soloists, we're a choir.
Michelle: That's right.
Rachel: Michelle, it's been a delight. As always, thank you so much for coming on the show.
Michelle: Thanks so much for having me. It was so great talking with you Rachel.
Content from the Library
Generationship Ep. #10, Ethical Benchmarks for AI with Dr. Marie Oldfield
In episode 10 of Generationship, Rachel Chalmers and Dr. Marie Oldfield explore ethical benchmarks in AI. This conversation sheds...
Building Apps with LLM Observability
In this talk, Honeycomb Principal Product Manager Phillip Carter discusses what LLM observability means, how Honeycomb approached...
Generationship Ep. #6, AI’s Cognitive Leap with Mark Wallace of Global Worldwide
In episode 6 of Generationship, Rachel Chalmers speaks with Mark Wallace of Global Worldwide. The conversation kicks off with a...