- Library
- Podcasts
- O11ycast
- Ep. #81, Observability 3.0-vNext-final-DRAFT with Hazel Weakly and Matt Klein
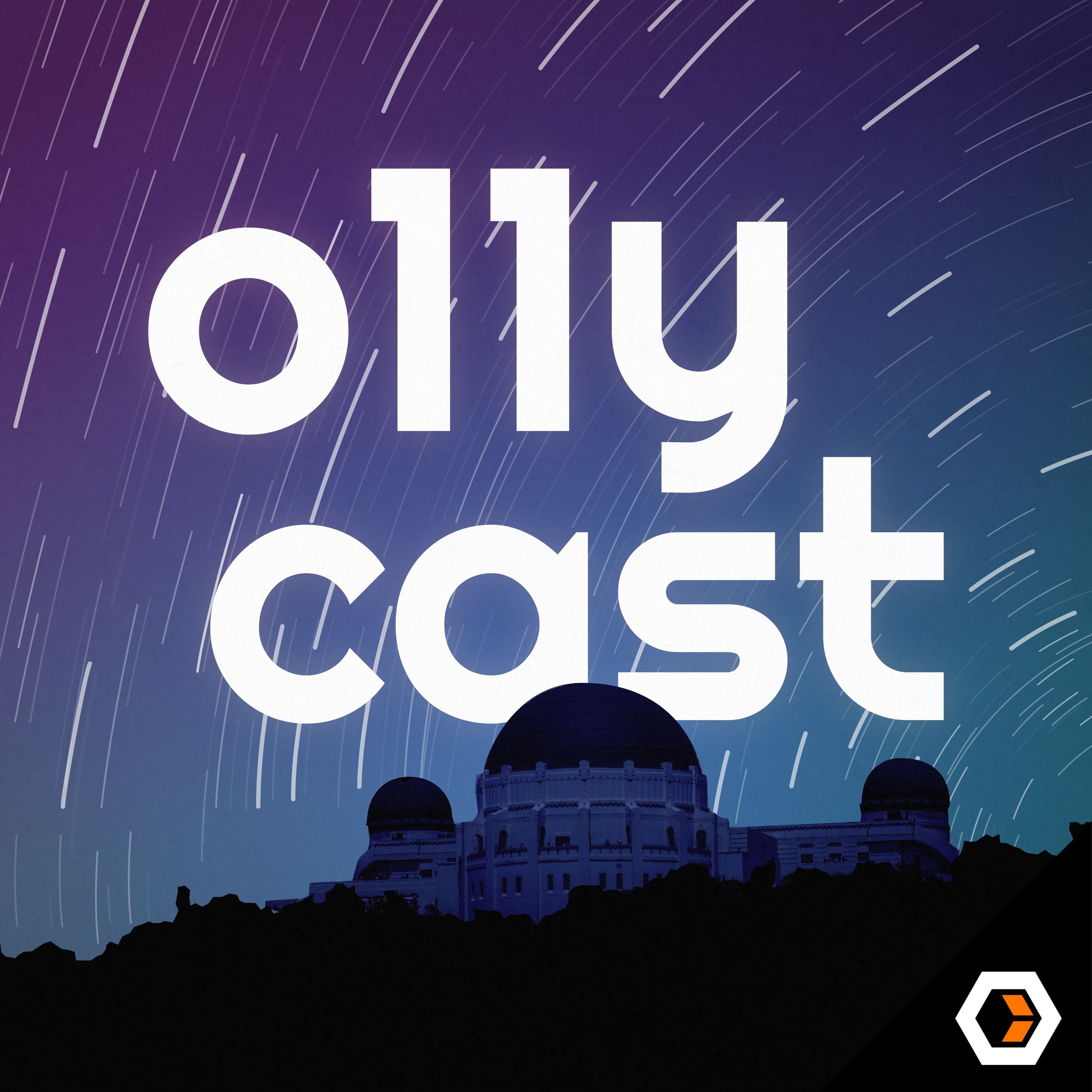
Ep. #81, Observability 3.0-vNext-final-DRAFT with Hazel Weakly and Matt Klein
In episode 81 of o11ycast, Charity Majors and Martin Thwaites dive into a lively discussion with Hazel Weakly and Matt Klein on the evolving landscape of observability. The guests explore the concept of observability versioning, the challenges of cost and ROI, and the future of observability tools, including the potential convergence with AI and business intelligence.
- Hazel Weakly is a systems thinker who tackles complex socio-technical problems. She writes and speaks about how to improve collaboration and understanding within complex systems, aiming to help the industry scale effectively.
- Matt Klein is a seasoned industry veteran with a background in low-level systems and application internet space. He created the open-source project Envoy and is currently the CTO of bitdrift, a company building a mobile observability solution.
transcript
Hazel Weakly: I think one of the things that's interesting here is as an outside observer, one of the things that I noticed is you have a trend of an incumbent company that becomes known for having this mindset shift or a paradigm shift in how you approach a problem.
And it's so easy to take a mindset shift or a paradigm shift and then reuse the word or reuse the label, keep your old thing the same, and then because you have so much interest in the market.
Whenever they do, you can avoid talking about the mindset shift, avoid talking about what's actually happening underneath the scenes.
And because it's, I mean it's a line item at the end of the day, for most executives, it's not letting them anyway.
They're going, we have this company, they say we provide value to the business. This other company, they provide value to the business but only hold the box sideways.
And that's about as understandable as the conversation can really get. And so if someone just copies the word over, they don't need to change the font, they don't need to evolve it, they need to actually do something.
Charity Majors: I mean, it's so much cheaper to talk about what we're doing than to build it. Talk to me about Datadog's infinite cardinality sometime. I dare you.
Matt Klein: Look, you know, I think from my perspective, as I was saying before, I will admit being recorded right now that my observability 3.0 was a marketing post.
And you know, I don't believe in as much in the versioning as Charity was saying.
And even though I am a vendor now, I am a longtime system operator. So I'm an observability user and I've been one for many, many years.
And where I'm coming from is, you know, trying to think about how I could have better solved my own problems. And then now as a vendor, how can I better solve customer problems?
And you know, where my posts came from, just stepping back from a technology perspective is, as I'm sure we'll get into--
There is a cost crisis in the industry. We all have many opinions on what means. And you know, I think as an operator, I've been frustrated for decades at this point on the inability to get the information that I need to solve actual end user customer problems and do that in a quick way, even setting aside cost. And I believe, again, sending aside versioning, that there are paths forward that we can take in the observability space that will allow us to, you know, solve customer problems faster and hopefully do that at a cost point that's actually lower.
So, you know, if it's helpful to talk about versions and the technological underpinnings of that, I think that's useful. But from a, you know, 1.0, 2.0, 3.0 perspective, again, I'll be the first to admit that that's entirely marketing.
Martin Thwaites: So we've got two amazing guests on with Charity, so I think it's probably important for people to understand the context of where these people come from.
So Hazel, why don't you introduce yourself. Tell us about you.
Hazel: So my name is Hazel Weakly. I have thoughts, lots of thoughts. They never stop thinking, they never stop thinking.
And I do systems thinking. I deal with complex socio technical problems.
I write about it, I talk about it and I try to help the industry and people figure out how to work with each other, how to collaborate and how to actually take really, really complex systems and understand how to work with them and learn together.
And then how do you scale that? How do you think about that? How do you do that better? That's kind of what I do.
Martin: Awesome, and we've also got somebody else on with us, Matt, tell us about you.
Matt: Hey everyone, thanks for having me. My name is Matt Klein. I've been in the industry for quite a while at this point.
Most of my career's been working on low level systems like operating systems, virtualization, those types of things.
About 10 or 15 years ago I went into the application internet space, mostly in the networking area, worked pretty early on in Amazon Web Services and then worked at Twitter and then Lyft.
While I was at Lyft, I created a popular open source project called Envoy.
And then a couple of years ago I started a company called bitdrift, where I'm the CTO. And we're building a mobile observability solution.
Martin: Amazing, and I mean most importantly, everybody here has wrote some kind of post around versioning observability, which is why we're here to talk about stuff.
So we've got 2.0, we've got both Hazel and Charity who mentioned that. And obviously then Matt just went right over the top and went, ah, we don't need two, it's fine.
Hazel: So when I came to 3.0 before I finished writing it and published it, I actually went to Charity and said, "Hey Charity, I'm about to kind of rain on your parade a little bit. Are you going to hate me forever?" And she said, "Absolutely."
Charity: Yes.
Hazel: But the reason I wrote the blog post is it's not really about versioning observability, but I titled it Observability 3.0, because I knew it would get people discussing and talking about it.
And the thing that I really wanted people to talk about was when I was trying to get the conversation shifting over to why we got here in the first place, which was we don't have a good way to talk about what we actually do with the observability thing.
It's one way to say, oh yes, we help engineers debug and troubleshooting systems, but there's no way for us to really articulate and package that up a nice way. We've just never done it.
And so my observability 3.0 post was really, hey look, we have observability 1.0 as this box for this approach that often has these tools and it talks about these types of questions, these types of answers that you have about your system. It tends to have these limitations.
You have observability 2.0 that has a mindset shift and has a paradigm shift and it allows you to ask a lot of different types of questions at a different point in your double shooting and debunking experience in your understanding the system.
But ultimately you limit it to with a certain, you know, shape, it's within engineering, it's all about having your data in a sense, in the same kind of format so you can query it in a certain type of way. Tends to be implemented in this way.
And observability 3.0 was really the box I put around what happens when understanding the system becomes sociotechnical, when it becomes, it's not just engineers, it's not just system engineers, it's not just product people, it's not just the people in the CTO work. What happens on the system?
Holistically is the entire company. What if you stop breaking down the having these silos, what if you stop dealing with things and putting it in a tiny little box?
And if you can do that, then you can start to ask very, very sophisticated questions that historically have been not your problem.
So many engineers might ask the question, hey, is what I'm building see driving revenue? That's not your problem, is it Mr. Engineer, Ms. Engineer, whenever.
It's not your problem, worry about your code.
Charity: Nobody does that.
Hazel: So many people, I know, I know. I'm surprised too. Most companies that I've worked at, I've asked those questions and people say, that's not your problem, stick in your lane.
Charity: Well that's a shitty place and they should quit and go find a new job.
I think on the long arc of history though, I think we're all kind of aligned that it's moving towards a data lake style model.
And I think that like the sort of journey that you know, with the proliferation of pillars and signals and everything getting stored in its own location, the problem is that as your bill goes up, the experience actually goes down.
Like the bio that you get out of them actually gets worse because what connects them? You do.
You, sitting in the middle of all these fucking pillars going, well that shape over there looks like that shape over there so they're probably the same thing or request ID over here, you know, oh shit we sampled it out of the tracing tool.
You know, we don't... And it's just, it's absurd. First of all, the cost multiplier, Gartner put out this research and they showed their customers on average use 10 to 20 tools.
So at minimum every request that enters their system is getting stored 10 to 20 times. That is a hell of a cost multiplier.
Your observability bill is going up 10 to 20 x as fast as your business is growing at a baseline, which is just, it's untenable.
Matt: And what you're describing is, at least for me, why we started bitdrift. Because again, like as an operator, I was exposed to the cost explosion.
I was exposed to not only the cost and the data multiplication, but that data is often written, it's rarely read. A vast amount of observability data is never read. Not by a human, not by a machine.
It's written and it effectively goes to dev null and even if storage is free, let's just, you know, take that as a hypothetical point.
And I think a lot of times vendors and users are, you know, quick to talk about how storage is getting cheaper and cheaper, but that does not account for network transfer and compute and all of the ancillary costs that are actually quite expensive and especially from the mobile world where the costs are even more, right?
Most large organizations that have mobile applications, they understand that the amount of data that you send and receive from the phone, it has implications on the end user experience.
And for many large mobile applications, this sounds crazy, the applications send more in analytics data than they do in actual application data.
It is crazy and I have been in so many incidents in my career where the data from the analytics system was dwarfing the application data and the application stops working.
So I'm just saying that cost is larger than just storage. It's all this data that's never read, it's having application impacts. It's the fact that once the data is there you have to sift through all of it to find what you actually want. It's a complicated issue.
Hazel: One of the things that this really reminds me of is, and you're seeing this in a couple different areas, all at once.
But one, you have the system and you have all these sort of levers of things and you don't feel the pain or you don't feel the feedback of something, it's really easy to build all these layers of innovation or evolution that don't take certain factors into account and then because you don't optimize and it's worthless.
Or you don't, you know, minimize something because you don't need to. You can build a system that becomes grotesque over time.
AI is actually a fantastic example of that. One of the huge hypes around AI recently is DeepSeek.
And it turns out the main innovation of DeepSeek was they just didn't have five mountains worth of GPUs lying around.
So they said, oh what if we just tried for more than 20 fucking seconds to minimize data transfer between GPUs. And then they got like two orders of performance just by picking up off the ground.
And we have these microservice architectures, you have these unlimited internet, you have this, you know, 100, 400, 500, whatever fucking gigabit networks.
You have these network NIC cards in the cloud. People go, oh I can transfer terabytes, terabits per second, petabytes per second between things.
And then oh this is for you, this is for you, that's for you, this is instantaneous. 10 years later, 15 years later, mobile finally gets involved in the picture.
People go, what if our websites didn't break? And then oh it turns out a system that has never once said, why don't we just care even the tiniest bit about the logistics of storing or handling or thinking about this telemetry?
And we just never cared about it for 10 years. So obviously it's great that companies like Embrace or you know, bitdrift can come in and say "look at all this fucking free candy on the ground that nobody's ever picked up."
And it's called cost optimization, network bandwidth, you know, optimization, this whole thing at the client side, some processing of it here, what if you just didn't transfer the whole internet every 10 seconds.
And what else is there for stuff, when finally we start asking the more people get involved, the more factors we have to deal with, the more in the system that we actually understand rather than externalize and right away and say we just don't need to care about that part.
The more we do that, the more holistically the system's going to be reasoned about. And so I'm looking forward to seeing what comes out of that.
So I'm actually very, very glad that you're doing the thing because someone needs to.
Martin: I think there's a lot to be said that we've applying the same techniques across so many different systems, but not taken into account how unique those systems are.
The idea that we apply backend techniques into mobile, into the front end, that they're subtly different. Like you know the amount of bandwidth.
Everybody assumes everybody's on a 5G network on their phone. Can transfer at five gigabits a second over WiFi.
Like not everybody's in that situation. Some people are on a 2G connection on a mobile. And these are all things that we need to consider when we do it, aren't they?
Hazel: I think just want to know is fascinating dualities of everything is different but everything is the same. And in either case the limiting factor seems to be we just don't transfer knowledge effectively and take what we already know somewhere and then put that elsewhere.
Charity: So what's the solution?
Hazel: I think that the solution is going to be multifaceted. There's no nice little box for it, it's systems thinking.
But like how did you as an organization get better at learning across all the different person, the organization, how does the industry get better at actually integrating that learning?
So like as an example, one of the thing the things that always bugged me about observability, is all these companies spend buckets on observability. They built our systems, they're imperfect. At no point does that learning about the system translate into anything anybody else can just download and share and get and acquire.
Like surely, if 60% of the development out there is front end, if 80% of not front end development is React, someone want to figure out how to share some type of knowledge about how to build a front end that's reliable.
And I'm not seeing any of that shared, any of that built or abstracted or any tools for that really being developed in the same way.
Matt: I think to me what's most important, so first of all, I completely agree with everything that was just said.
And the way that I approach it is I like to start with the end user business results. Like I said this many times.
But at the end of the day, you know for those of us that work at, you know, companies and we all work in infrastructure and all of those things, we are there in service of the business goals.
Like we're not there to build beautiful infrastructure. We're there to you know, sell whatever widget it is that the company is actually selling. So the business goals are the most important things.
And what I have often found crazy with the way that we approach many of these infrastructure systems is I think we tend to look at them as end results in and of themselves, right?
Like versus looking at the end result of what we're actually doing. And a key example of that is we as an industry, we love to talk about success rates.
We love to talk about, you know, SLOs and measuring success rates and looking at our 99.99 whatever, seven nines, blah blah blah on our server.
And the number of times in my career that, you know, some JSON has put apps into some perma crash situation, right?
And like it's returning 200 from the server and no one knows, the SLO is perfect, everything's great, all the users are frustrated, they're quitting, they're like going to some other company.
It's happened so many times. And that's just an example of, we're solving all these technical problems but are we solving the right problems?
Charity: As someone once wrote and put on a t-shirt, "Nines don't matter if users aren't happy,"
Martin: Who was that?
Hazel: My version of that one is you probably only need one nine, it just has to be in the right place.
Matt: I agree. Absolutely.
So my problem with observability again just as a industry and I'm a vendor now to be clear. Is I don't know yet that as an industry, and this comes back to what Hazel was saying, is that--
I don't know that we're helping people solve the right problems and get the right information at the right price. Because at the end of the day it's some intersection, It's a math formula, right? About like information versus price. There's some cost benefit trade off. And as an industry, I don't think we're there yet. I don't think we have the right cost benefits right off.
Charity: Yeah, I 100% agree.
Matt, I have enjoyed your blog post so much over the past couple years 'cause you have come out with a flamethrower on multiple occasions.
When we were, for those at home, when we were doing our little pre-check, you said something kind of spicy in our little group here, which was that something about, we were talking about the cost crisis in observability and I think your line was, "I don't think people care."
Matt: Yeah that is a spicy take.
And I think what I've realized over the last couple of years, it's not that people don't care, it's that typically in organizations, the users of observability systems are different from the buyers of observability systems.
And that is a really key point in the sense that users of a system, you know, you give them a Datadog interface, something that's been developed over many years and has lots of features and whatever else and they're very happy.
They don't care what it costs. It's the CFOs and the buyers and the people that as you said are looking at their bills go up 40% year over year, they care.
What I've come to realize though is that in our effort, you know to bring better ROI in these tools, what's actually important is the product has to be better and it has to cost less, right?
Like it has to be both. And I think what that means is that we have to get people better value for the data. And sorry, I'll just add one thing real quick.
And this is going to be another spicy take. Is that we had a lot of years in the mid 2010s, and these are mostly the observability, I'm using air quotes, you know, since we don't have video for the observability 1.0 tracing systems.
Observability 1.0, tracing systems I'm just going to say are useless. I mean they are useless.
Charity: Yeah.
Matt: I mean they're actually worse than useless.
Like they have negative value because you spend so much money and time like emitting all this data and then find me a fucking trace that actually tells me anything about the problem that I'm facing, impossible, right?
And like I was one of these people that deployed one of these systems and I regret it every day, right?
And to me that style of tracing is a great example of we as an industry put out these tools, we got people to buy them and they had negative ROI, right? It's like we're not helping people.
Charity: Also worse than useless because rolling out 1.0 tracing was such a massive organizational lift.
Matt: Absolutely.
Charity: It took so much coordination and then the payoff was like not there.
So people lost credibility that they desperately needed. And part of the reason it had...
Like there are so many places that put in all this work to rule out Jaeger or something in the 1.0 error and two, three, four years later they have all the traces.
But when somebody has a tracing problem they go to the... There's like one or two priests. There are the people who understand.
They go to the priesthood to ask for help with their tracing shape problem instead of going to the tracing tool. 'Cause it's so siloed off, it's not connected to their data.
You know, it's yeah could not agree more.
Matt: Well I was say but that's assuming that the priest even has the data because it was probably sampled in a way that makes it impossible to find the data that you even need.
Charity: Yeah, and a lot of folks just threw up their hands were like, well I guess tracing sucks. When in fact tracing in modern complex systems is necessary.
But it's like a whole new generation that's having to figure out how to roll it out in a way that is like unified, no dead ends in your data and all this stuff.
Martin: I spent years as an advocate for new sampling because you should never do it.
And then I realized when we started working with a rather large retail organization that was saying, oh yeah we're doing head sampling at one in 200,000. I'm like, what?
It's like yeah we've still got 14 terabytes of data an hour coming through the system. I'm like okay that's probably a good reason to sample, because they were getting the representative samples.
I hate the current modern day sampling stuff.
Matt: Right, but for that unnamed organization who's doing the whatever one in 2,000 or whatever sampling and they still have the terabits of data, you go and talk to those developers about how often they can find a trace that is for their specific problem.
The answer is often "no." Right?
And that's where again, like I have my own biases about, you know, why bitdrift is doing what we're doing with the ring buffer and local storage.
And I know Charity will say that that's not as applicable to server. And honestly I agree.
Hazel: Actually I think it is applicable to servers because if you think of, and I'm going to go way off into the weeds for a brief moment as I am wont to do.
If you think of observability and the distributed system and the cardinality of a distributed system, the cardinality of the distributed system can be looked at using measure theory in order to make a metric space.
And so if you take the metric space and you break everything down to equivalence classes of a trace, rather than into a particular point of a trace and what you're doing is you're actually using your tracing, you're using your sampling as a way to verify that you understand the behavior of the system better.
Do you know what a normal trace under certain conditions looks like versus what an abnormal one looks like?
Being able to validate that and scale by way of implementing your tracing and then being able to double check that. It's really, really valuable.
And so the idea of having this sort of, we dump everything here and then we can sample here and maybe continue to sample and incrementally refine it. And then double check things.
Charity: Well, I'm not saying that there's absolutely no value.
But I am saying that two of the hardest, most interesting questions in large multi-tenant systems with a lot of concurrency problems is always going to be what's happening right now and what just changed?
So you can't wait for, oh okay I'm a developer, I'm now ready to look at my... Like it's just a different model.
And I think to Matt's point from earlier, we need a lot of models to solve this problem.
Matt: I think too that like, whereas if you look at mobile, again I'm biased.
I think trying to do proper observability without the ring buffer local storage and control plane is basically impossible. Like I actually think it's impossible.
On server, where I think we're going to land, is more of a hybrid approach in the sense that there's always going to be logs that gets sent all the time, right? It's like there's always going to be signals that get sent all the time.
Where I think that the local storage and the control plane will come into place on server is, you know, maybe foreshadowing our future product areas, is I do think that there are types of tracing, especially processed local, that can be driven entirely by this control plane system.
So for example, let's say that I want to look for per user request data on a particular server, right?
Like the ability to say that I want to monitor a particular user or a particular set of conditions and actually get an entire local trace within a process given those confines.
I think what it will allow you to do is to, for example have your logging, you know, be it info level or general span level but get like super detailed data, you know, for a particular use case.
So I think on server to your point Hazel, I think we will see some of these hybrid systems but again I'm biased but I do think that you know, this level of control in real time will be applicable in different places.
Hazel: I think you can do it in real time as well. But you're also going to see a lot of security integration as well 'cause this is so much data.
But the security tooling at some point is going to have to merge the observability tooling in some sense and same fire hose. They can both share the fire hose a little bit, because if you're looking at like highly compliant companies and a lot of regulation.
They need to look at the packet level, they need to look at the network flow, they need to correlate that to every process on the machine. The need to correlate that data.
There are regulatory requirements to require that level of per packet inspection and per packet actually analysis of things.
Charity: I would actually think, here's my spicy take, I think the security use case might be one of the only ones that doesn't belong in the data lake, because the way that people need to interact with it is so different.
Like typically I think you can just drop it in fucking Glacier and like you don't need a lot of sophisticated analysis things. Whereas with most other stuff, the analysis is the most important stuff.
Martin: We've probably covered observability four, five, six and sevens so far.
And I had a question for each of you really, which was what does observability 10.0 look like? You know, 10.0 is going to be probably the next three years.
Where do we sort of see ourselves moving in terms of tooling and mindsets and users' mindsets and organization's mindsets?
So I'm going to go with Hazel first. What do you think is a 10.0? Where are we going to be?
Hazel: So I think we're going to see a couple different things all at once sort of wrapping around things.
One, is you're going to see people sort of demanding that the ROI of your observability system or things of that nature come from more than just engineering. If just engineering is getting value from the product, why does it cost 10% of your entire or 20% of your entire budget?
That might be a really hard question. People might start really, really wanting that. I don't know if it's going to be necessary.
I think people going to start asking the question of okay big expensive thing, is the ROI just in engineering or is it more and we want it to be in more.
The other thing that I think is going to happen, is we're going to start seeing a lot of the concepts of business intelligence and business analytics, these types of systems, the learnings that have happened over there for decades are going to start shifting over and we're going to start cross sharing maybe some data, cost sharing some analysis, maybe some tools, maybe the blend and the line between this is business intelligence and this is observability, it's going to start becoming and looking a lot blurrier.
So lastly with that, systems thinking in general requires quick dirty tools.
And so you're going to start to see as we understand how to do systems thinking better, people are going to demand from their observability tools less of, I want this polished, ornate, shiny button that does a thing, and more I want a bunch of quick dirty units like things that I can smash together to get information out of my system and actually reason with it.
I want to get my hands dirty in this and play in the mud. And they're going to start wanting this out of the systems that have messaged and positioned themselves as polished.
You're going to have to start figuring out what it means to be a polished system with messy tools.
Martin: So I think that it's interesting there. So what we're saying is everything's going to merge into BI, and data and security, one big tool.
Everything's going to merge together, but also everybody's going to go for their own little dirty tools.
So we're essentially going to go and evolve but also devolve at the same time. And we're going to disband between the two, which I think is kind of what we already do in every single industry.
We amalgamate around one thing and then everybody goes that one thing doesn't work for me 'cause you've tried to be one tool for everything and then everybody goes, oh I just want this quick and dirty one.
Which I think is perfect. So what about you Matt? What's your alternative future?
Matt: I actually agree with much of that. I believe that as was said, that the perceived ROI--
The problem here is that as we all know, measuring developer productivity, it's like impossible.
I mean people love to talk about it, people love to bloviate about it and attempt to measure it and you know there's some bullshit about Google measuring developer productivity and whatever else.
It's like it's very difficult to actually measure these things. So a lot of it is subjective.
But at least from a perception standpoint, there's a perception in the industry right now that the money going in to observability is not yielding the sufficient ROI out.
And I believe the solution to that, that we as an industry are going to have to iterate on, and this is where I differ with Hazel, is that I do think that though the tool is going to be similar or the same maybe, I think there are different use cases.
I agree with Charity, that like the security seven year storage use case shtick in Glacier, is different from the, I want to debug right now use case.
And I think that observability has to become a lot more dynamic. Meaning when I'm debugging a duplicate care right now, right?
Like I want to know right now what's going on. Maybe I want a little bit of look back, but I want more control without doing deploys.
If I'm doing security, I know mostly what I need for compliance. I want us to wear it for seven years.
And then there's some middle ground of things that I know that I want to alert on and maybe I'm going to, you know, get those on a more constant cadence.
And I guess my point is today I think the tools restrict us. We tend to have to emit logs and we have to use those for security for seven year storage and then we convolve the debugging cases and everything else in there.
And I think to get the ROI correct, we have to look at these cases and basically allow the system to adapt and have a very real time dynamic component, a long-term durable storage component.
And make those knobs super easy to turn so that people feel that the data they're getting out they're using, they're not using 1%, they're using 95% and if they're not using that data, they just turn it off.
So that's my observability 10.0, it just has to be more dynamic.
Martin: Okay, Charity, what do you want from 10.0?
Charity: Ah, Matt, I think stole so many of my little bullet points there. About the dynamic and the durable storage and that. Yeah, 100%.
So I guess in order to have something that is not just agreeing, I'll throw in a perspective of we've got to get better at, as Hazel said, you know, aligning, you know, what engineers are doing...
The data, I think yes, I think observability engineering teams are increasingly going to be data engineering teams.
I think telemetry pipelines are emerging as a way for us to do a lot better data governance.
But there are two different ways that observability makes a huge contribution to the bottom line.
And I think that when it comes to the sort of external half, every big company has done a lot of work here.
You know, Google will talk about how for every a 100 milliseconds you add to search, you drop 20% of blah, blah, blah.
Or Amazon will be like shopping carts, .you know, user interfaces. It's pretty easy for us to quantify an ROI on that data.
But when it comes to the internal half of observability, I think of it like the dark matter of software engineering, it's the stuff you can't see because things are just slow, but you can't see why they're slow.
All you can see is all the things that you keep having to do, you know, and so much of this sense making comes down to having observability that allows you to make sense of your systems in pretty much real time.
And it's like, I use this metaphor a lot, it's like putting on your glasses before you go barreling down the freeway.
If you can't see, if you can't get those feedback loops fast enough, then you feel like you're veering all over, you're constantly like changing the course and when you're sense making is fast and effortless, it feels like you're just driving.
It feels like you're just building. And I think that AI has so much to like, we haven't really talked about AI at all, which is kind of weird, but like.
Martin: I'm really impressed, we've done well.
Charity: I know, right?
Martin: Well done everyone, pat yourself on the back.
Charity: But when it comes to instrumentation, I think a lot of the cost crisis has been built by this sort of like drivers of auto instrumentation that has gathered a ton of shit that people don't actually need or want, but it's how they built their dashboards and their insights so they can't turn it down and it's generating all this crap.
And I think that AI's going to help us there. I think that we're going to move from dashboards to workflows. And that we're really going to pay, like...
Developing with AI requires fast feedback loops to do it right and do it well.
And so my hope is that we're going to get better at identifying the sort of the cost of bad observability or not having observability.
And that teams are going to start to connect the dots between the dark matter that is just constantly like slowing us down and making it feel like we can't make progress.
'Cause it feels like a lot of changes are kind of converging in the same direction and ultimately, you know, I think that's right.
I think developers don't really care about the cost of their tools. I think that what they do care about is that cost. It's not the absolute dollars, but it's the rate of increase.
It's unsustainable and the value that we're getting out of our tools, you should have predictable cost growth and it should go up or down as the amount that you spend goes up or down, right?
The value and the cost should be going up or down in alignment with each other. And that's what I think a lot of the experimentation on our part and a lot of vendors in the market consists of right now.
Matt: I think developers at the end of the day, they just don't want to get yelled at about cost, right?
They want to do their job, they want to have, you know, all the data that they need to actually solve their problems and they don't want someone coming and actually yelling at them.
And as we were saying before, it's completely subjective as to what is the right number. You know, is 20% of your budget the right number? Ten, five, one?
You're not going to get one answer on that.
Charity: But there's a big difference that I want to call out between are you managing your observability bill as a cost center or as an investment?
For cost center, you just minimize it. And if it's an investment, you try to find the point at which you reach diminishing returns.
And you actually spend... You want to spend up to that point. Because the point of an investment is it pays for itself and then some, hopefully it pays for itself five times over, 10 times over.
Matt: Yeah, and this is where, you know, if we want to end on the hottest take, you know, at least for myself, I'll say that at least in the industry today, when most observability vendors are charging by ingested data volume, this is where I think the incentives can be off, right?
And I do think as an industry we have to involve, and again, I am biased, you know, at bitdrift we do not charge on data volume.
Like we're charging more on the workflows, on the state machines on those types of things. Is that correct? I have no idea. We're a tiny company.
But I do think that, you know, the volume based incentives, I think the vendors, we vendors have to be incentivized to again try to get people to use more of the data that they're actually producing. To me that is...
If we're to come up with one metric that matters, it's how much data that you emit do you ever look at? And I feel like as an industry we should try to optimize that.
Martin: So I think we could probably do sort of a series of 20 of these podcasts and still not really have gone deep enough.
But unfortunately we have to really think about our listeners' time because they don't have four hours.
So I think we'll probably have to call it and maybe we'll come back in a few months and we'll do something new. We'll find out whether everybody's approach is.
And maybe we'll come back in four years and we'll find out what 10.0 really look like. So yeah.
Where can we find you Matt? Online? Are you on Blue Sky, Twitter, X, whatever?
Matt: These days, LinkedIn and Blue Sky I guess. I don't know. Like social media is kind of a mess these days.
Martin: And what about you, Hazel? Where can we find you?
Hazel: You can find me pretty much everywhere except the fascism websites. So I'm on Blue Sky, I'm on LinkedIn, I'm on Mastodon and my website is hazelweakly.me. And you can find all the links there.
Martin: Okay, so if you want to know where the spice is, you know where to find it in all those places.
But thank you so much for joining us. It's been an amazing discussion.
Charity: Super fun.
Matt: Thank you so much.
Content from the Library
Generationship Ep. #38, Wayfinder with Heidi Waterhouse
In episode 38 of Generationship, Rachel Chalmers sits down with Heidi Waterhouse, co-author of "Progressive Delivery." They...
O11ycast Ep. #83, Observability Isn't Just SRE on Steroids with Dan Ravenstone
In episode 83 of o11ycast, the Honeycomb team chats with Dan Ravenstone, the o11yneer. Dan unpacks the crucial, often...
O11ycast Ep. #82, Automating Developer Toil with Morgante Pell of Grit
In episode 82 of o11ycast, Ken and Jess chat with Morgante Pell, the visionary behind Grit, an AI-powered agent designed to...