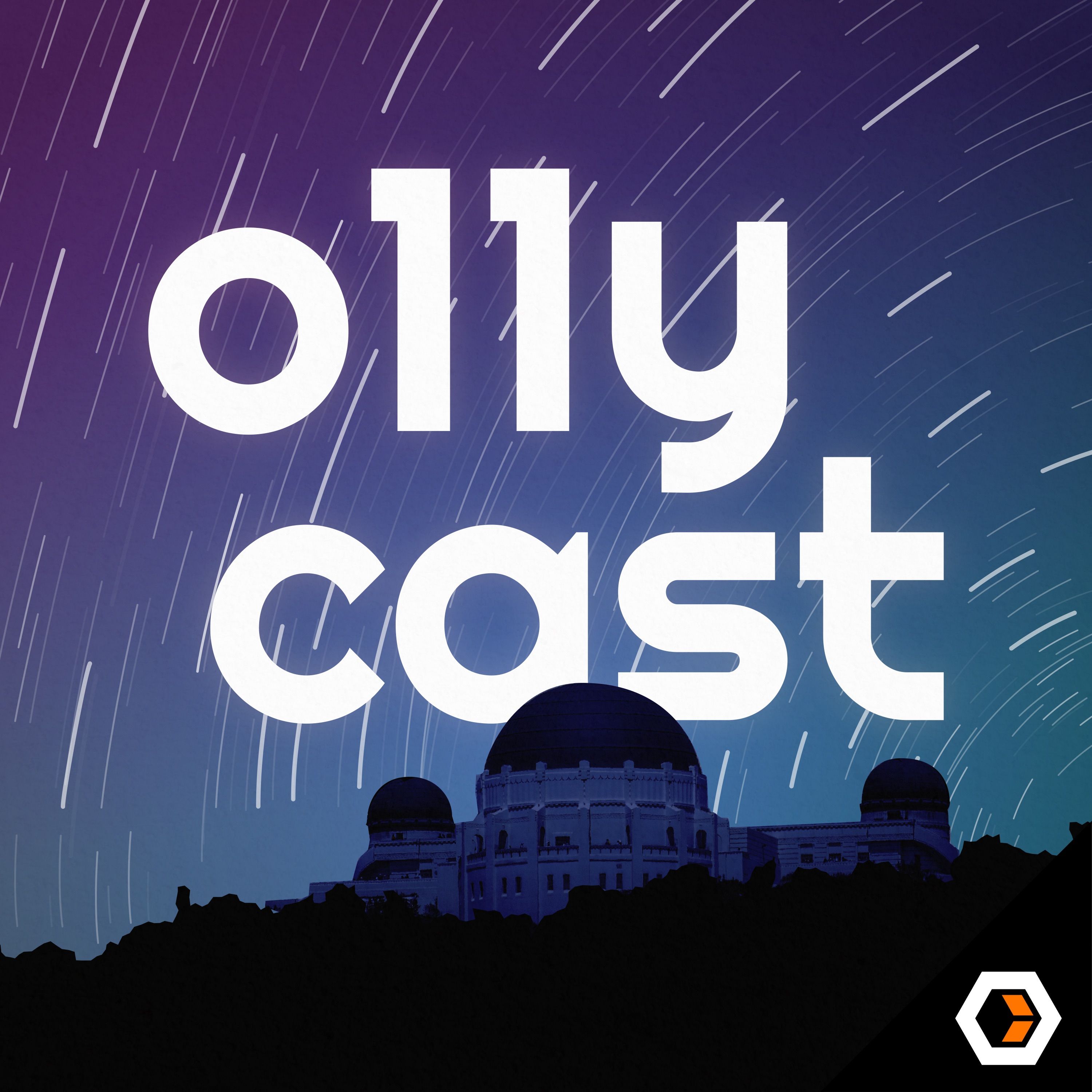
Ep. #69, Collecting Cybercrime Data with Charles Herring of WitFoo
In episode 69 of o11ycast, Jess and Martin speak with Charles Herring of WitFoo. Together they dive deep into the world of cybercrime investigations. Discover the intricate processes of data collection and analysis in the realm of cybersecurity, all while balancing the imperative of cost-effectiveness and citizen privacy.
Charles Herring is CTO, Co-Founder, and President of WitFoo, the world's first diagnostic SIEM. Charles has served as a problem solver for the US Federal government, international relief agencies and businesses ranging from local law firms to the Fortune 500. He is a regular speaker at information security conferences and avid security blogger.
In episode 69 of o11ycast, Jess and Martin speak with Charles Herring of WitFoo. Together they dive deep into the world of cybercrime investigations. Discover the intricate processes of data collection and analysis in the realm of cybersecurity, all while balancing the imperative of cost-effectiveness and citizen privacy.
transcript
Charles Herring: Our role is to look at the world of cyber crime, and we built a company on this basic thesis that the reason cyber crime is such a problem is that we're not able to work with one another. That one organization can't collaborate with another organization in the way that we would, as a say, like a neighborhood watch.
We're not able to call the police. The police aren't able to get information that they need to do their work. And all that really is based on how data is collected, analyzed, and presented to the right person at the right time. So that's the outcome that we want out of our software. But in building that software, we have to understand another set of metrics, which is how is the software working at performing those outcomes? How are we collecting the data? How are the users interacting with the data?
So there's a whole other subset of data on the success rates, whether that's machine to machine success rates, or success rates from machine to people, or sometimes people to people, when they're trying to explain their version of the report to another person that's in a different domain. So really it's about having information, having data, having analytics that one, are accurate, right? That do tell the whole story, or the correct story, the accurate story.
Potentially, I mean, tell the right story when we're talking about putting someone in prison, or a national security outcome. It's very important that we be as right as possible in making those decisions because we're taking away life and liberty in some of those outcomes. So being accurate is extremely important. Having all the facts, having all the data, having that all in the right context is job one, and not making a mistake in that area. But then also the handoff of the data to the processes.
People are going to do things. There's going to be arrests made, and presentations made to grand juries. There's going to be insurance claims. So the way that that story, that data, that analysis goes from being a work product in one system to being a work product in a different process is very important to us. And that mistakes aren't made because of lost in translation type of issues.
So, sort of accuracy and comprehension throughout the entire lifecycle of data is what we aim for, or what I call the predestination of data, that as soon as a signal is born in this world, we need to know everything that's going to happen in its lifetime, how it's going to be used, how it'll be evolved, who's going to observe it, how the person observing its bias history impacts the use of that data and how that's going to impact the course of human histories.
So sort of thinking about the butterfly effect, if you will, on every signal going into every system is what we're working towards.
Jessica Kerr: And you're talking about signals of data about cyber crime?
Charles: That's right. And you know, cyber crime is just a subset of crime, right? And so things that you know are signals of cyber crime, like a malware or virus detected, that's a signal that you have a pretty high understanding or confidence that it's associated with cyber crime. But other things paint the picture, right? That this computer just talked to this computer at some point in time.
And so for us, the data we're collecting is every log from every tool, from every network device, from every service, from every workstation, all the stuff that's in the APIs that you're paying for through SaaS or some other service. The things you're generating internally through private logs, all of that information is digital evidence that has to come in. We have to receive it, we have to comprehend it, we have to put it into the context of a potential crime theory.
Something's happening, like ransomware, extortion via ransomware, or data theft. We have to preserve those things. And even small organizations, it's normally north of a terabyte of data every day that's being generated from all of these signals, all of these systems, and they all speak their own unique language.
Every firewall speaks a different language. Every service speaks their own language. So, it's confounding, like receiving, comprehending that data, and then translating it into different levels of evolution for different audiences to consume to do their processes.
Jessica: This is a lot. You'd better introduce yourself.
Charles: Hello, I'm Charles Herring. I'm Chief Technology Officer and co-founder at WitFoo. At WitFoo, we help the world become secure together by connecting organizations in the public sector and in the private sector, enabling them to share information to reduce cyber crime and reduce the cost associated with cyber crime. I also scuba dive.
Jessica: Excellent. Right, so on the one hand, what you're doing at WitFoo is a use of existing telemetry that most of us don't think about. Because it's not just going to like, internal security teams. This is stuff that you're processing a terabyte of data for a small org, you said, and you're doing this across organizations?
Charles: That's right. There's a number of things you have to solve for. One is the cost of it. So how do you process a terabyte, or petabytes of data, and it not be prohibitively expensive for most organizations?
So we spend a lot of time on how do we maximize the use of the resources that are available? How do we use less expensive resources so that it can be broadly available, so that you're not the cyber-rich versus the cyber-poor, and who's able to have access to this global cyber grid? And so that's one area we work on.
The second is, how do we safely share information? You know, I tell my law enforcement friends that if I get rear-ended in a car accident, it's fine for you to come and take a statement at the car wreck and write that up. But it's not okay for you to go home with me and go through all my stuff, right? There's a level of privacy and expectation.
And so when we're sending information to one another, so if my house is broken into, or I see someone on a Ring cam, or something that's interacting in a potentially nefarious way with my home, I do want to tell my neighbors, but I don't want to tell my neighbors everything that's going on in my home, right? That's not how we work as human beings. We don't share everything. Sharing increases vulnerability and risk.
And so we do want to share it to be good citizens, to be good neighbors. So how we handle the information is second. So the first thing is how do we keep it cheap? The second thing is, how do we keep it safe in the scope of privacy? How do we share enough information so that we're able to help one another, but not so much information that we're causing harm in the act of sharing?
And so those two make up a big part of what we're doing and allowing similar types of collaboration as we have in the real world. So, law enforcement agencies can publish bulletins that are similar to like, an AMBER alert. So you'd be on the lookout for these things, these files, you know, connections to these services, these types of people.
Jessica: This link in an email?
Charles: This link in an email, be on the lookout for this. And they publish that bulletin. And then our system, our customers, our users, see the Chicago Field Office of the FBI's requesting information that you have in this collection of evidence, will you send it to them? And so the FBI doesn't know that you have the information, WitFoo doesn't know you have the information. But you know.
In the same way that if you saw the car driving by in an AMBER alert, you would be obligated to call the police. You may or may not do that, but it gives you the ability to collaborate and submit this affidavit.
Also, we do things like anonymous tips. We are seeing these things, but we're not going to tell you who we are in submitting the tip, because we're worried about the same things we'd be worried about if we were calling the police about a robbery or murder or those types of physical crimes.
We don't want to be hurt by the criminals. We're worried about the risks that us giving a sworn affidavit may pose to ourselves, to our own organization. So, those levels of how and when do we share, we just tried to model on how do we handle physical crime, when do we share, how do we share?
And then on the digital side, because there's so much more complexity, you know, this podcast or recording in my home is generating several hundred metric signals per second, just us having this conversation, and that's being processed. And hopefully it's never going to be connected to a court case. But you never know what thing is happening.
And you know, unlike physical crime, digital evidence, if you don't prepare to collect it before it exists, you don't get to collect it later. You know, this table will have my fingerprint on it tomorrow. And so if they came and dusted, they would find my fingerprint. But this conversation, if you're not recording it, if I'm not recording it right now, I can't come back tomorrow and receive the metrics that concern this conversation, in the same way that you can't put a camera out today to record something that happened yesterday.
And so having all of that thought out, right, what data needs to be received? How do we analyze all of this seemingly benign data evidence in the same way that a law enforcement officer might look at where someone was. If they were across town when the murder happened, they couldn't have done the murder, right? So them being across town, their geographic location isn't a signal of crime, but it does help draw the picture of what occurred.
So it's really important for us to have all of the evidence. And the third part is being able to prove, legally prove that the evidence is true, right? So we have to deal with good defense attorneys. If this hash doesn't fit, you must acquit, kind of a Johnny Cochran approach. So you have those things to deal with, being able to show your work, and also explain it to a jury of landscapers and plumbers and non-technical folks that don't know what a firewall is from a, you know-
Martin Thwaites: A wall of fire.
Charles: Right. Just watch out for the wall of fire. So those use cases are taught, but I think those three main principles, you know, optimizing for cost so that it's affordable broadly, keeping the sharing as safe as possible, and giving the control of the sharing to the person that owns the risk. And then checking the work, proving, validating that what we've received is true and it's verifiable.
Those are all sort of key components. And you know, there's a lot of use cases we have, but as you can imagine in the development side, there's a ton of metrics we have to understand to do that because we're writing software and our software is sometimes deployed in private cloud, sometimes deployed on a ship at sea, sometimes deployed on the submarine under the sea.
And so all of these diverse environments where they're collecting information and the periods of sharing information and collaborating are going to be unpredictable. And so it's not as straightforward as building a SaaS offering to where we can go and just look at the logs and say, okay, users are up. Sometimes we don't know what the users were on.
We won't know all of the users' data today until a few weeks from today. Some of it we're going to have in near real time, but the full of it we won't have until a metrics window opens up for some of those use cases. But they do drive things like, how expensive is the hardware? Can we write this function or this method in a way that could reduce the RAM needed on the machine, right?
If it's a heavy piece, can we reduce the CPUs needed? Can we reduce the speed of the disc? Can we reduce the number of times we're hitting the disc? So that first tenet of just measuring every single method in how fast it's running, and also figuring out when is it crashing? That's sort of table stakes. And we spent almost five and a half years just researching that.
And so we deployed about 30 different deployments in different organizations. And we had this data, these signals, about processing speed and function coming up to us. And we could run experiments on, you know, do AB testing in some of the environments, we could do AB testing against the data.
But the whole point of that was how do we reduce the cost of the architecture when we're talking about storing potentially years worth of this data? So, petabytes and petabytes of data.
Jessica: Wait, wait. When you're talking about storing petabytes of data, is that the user's telemetry that's like, who got an email and which computers talk to each other that you're storing?
Charles: Yes, that's right. Because that's evidence. And so sometimes we may not know that there was a crime when the crime's happening.
That's another bad problem with cyber crime. You know, if I get mugged, pretty close to the time of mugging, I'm aware I got mugged. But if I steal your source code, your source code's still there, right? If you steal my wallet, my wallet's not still here.
And so a couple years ago we had the SolarWinds breach, and there was just this massive global breach from that software through a supply chain attack. And we didn't know about it until almost nine months until after it had started. So that meant to see if you were hit by it, you needed to check your logs going back nine months in the past.
Jessica: Oh, wow. So are you responsible for storing all that?
Charles: So we write the software that stores it. We have service providers that offer SaaS offerings, and typically they offer a year's worth of storage in their architecture for their customers. We have some customers that build their own architecture in public cloud or private cloud, and they store it.
So, I'm a software company, which I believe is radically different than a SaaS company or a services company. And so, but we do have SaaS partners that use our software, and so we have to provide them tools on monitoring and maintaining those clusters, sometimes hundreds of nodes, or several hundred nodes to maintain that data.
Jessica: So your software, and sometimes hardware, is in other people's networks?
Charles: That's right. Yeah. So I only write the software. And so our software is deployed, so you can go to the marketplaces, you know, AWS or Google or whatever, and you can launch the instance or multiple instances of our software in your own tenancy.
So that's one way that things are deployed. We have service providers, one in Chicago is called Impact, or Impelix, their Impact platform where they host our software and you're paying them for the software, you're paying them for the hardware underneath it, and you're also paying them potentially to monitor for security incidents.
And then we do have hardware partners where you can purchase the software, you know, installed on the hardware. So my focus is the software and then meeting the needs of those solution partners, whether it's hardware, Amazon for public cloud, Google, Oracle, all of those, Azure Microsoft. But we just write the software that has to work in all of those scenarios, and they have to work together in all of those scenarios.
Martin: And they have to work with unknown data sets. You know, we promote this idea of wide context data and providing more and more. I mean, I've worked in places where there was tons and tons of log files that were useless most of the time.
And the schema of these things is going to be incredibly diverse across all of those things. And you've got to deal with that complexity of not failing because somebody put a semicolon in when they shouldn't have put a semicolon in the log file and that built a new log file because of it.
Charles: Yeah, that's absolutely right. The comprehension of logs is maddening. I have a therapist and we spend about half the time- Half the time talking about, you know, business loads, another half the time talking about, why in the world would someone write a parsers this way? So he's become really smart on how messages, message formats are written.
But you know, talking about that, it's actually an interesting thing that we handle is, so let's say we have a customer that's in, let's say they installed it on a Raspberry Pi, and we can do that too. So you're on a Raspberry Pi. I have one running here at my house, and I get a new tool or a new application, and I'm sending my logs to that WitFoo box.
What's going to happen is the signal is going to come in and we have this large database of tens of thousands, I don't even know what the count is now, of different fingerprints for messages. So we take the message and we tokenize it. So if it's a timestamp, we'll replace the actual timestamp with a variable called timestamp, right?
And so we do that for, there's an integer, and you have this pattern that's sort of built when you take all the variable data out and you're left with this fingerprint. And if we have an exact match for the fingerprint, we know everything about the message. So I call it the etymology of the message. Who wrote it? Who's the developer? So I have a hit list, in case you're wondering.
Some people ask, just want to dig down, like why, what happened? What happened to you? What trauma happened in your life where you decided you needed to pad each octet of the IP address with four zeros? Why? But who wrote it? What the product is?
What's the versions of the product that use this message? Who owns that? What vendor owns that product? What is it trying to tell us? Right? How does this map to things like, is it telling us this reconnaissance.
There's great cyber frameworks like the MITRE ATT&CK continuum that tells us sort of the different things that happen over the course of different types of attacks. We say, this message happens when someone is trying to crack our password, right?
And so the same way that, as a detective, having to understand what someone's saying, we also have to understand why they're saying it, because the fact that it generated the signal now, but it didn't generate the signal earlier, we have to understand what conditions led to that. And there's all kinds of nuance. Like, sometimes firewalls will stop logging some things if it gets too busy.
And so you have to be aware that sometimes those conditions occur and there's going to be this gap. So essentially we get a signal. If we know what it is, that's fine, we're able to do all of our processing. We put that into a normalized JSON schema, and then it moves off the processing pipeline.
If we don't know what it is, we serialize that fingerprint and say, we have a fingerprint we don't understand. And then the customer will request, can we have a sample of those messages? So right now we just have like, count on this fingerprint seven, we have a thousand of those happening every minute, and they're happening at three customers now.
And so we'll request a sample of the raw messages, we'll see the raw messages, and then we'll put that through some basic machine learning. We scrape the whole internet on these log formats, and I'll say 99% chance this is written at Cisco, it's probably a fixed or ASA firewall written by this guy.
And so it'll give us sort of the summary of what it thinks it is, and then we'll call Cisco and say, man, what were you guys, what's going on here? You know, why this today? And the same thing with API connections , and all these things change.
So the Slack channel we have internally on these things is comical. It could be a TV show on just the rage that we have over these changes. But we write the new etymology, the new frame, semantic frame, and then we push that out. Then everybody that didn't understand what that message is now understands what that message is.
But you know, even in that format, we have these just pure metrics counts, you know, unknown fingerprint seven, we have five customers, here are the five customers, here are the counts that we're not currently able to process. And so that's just how we know there's a problem. And then we need to get down to the artifact, the raw data to understand, how do we fix it, right? What's going on here?
So that whole pipeline's a big part of what we do at WitFoo, it's just constantly documenting the changes. You know, the Wit of WitFoo is just what do we all know, right? Our common collective keenness of mind. And that's always changing. So our, what we call WitFoo Library, is collecting all of these different things we know, right? These different parsers, these different attack frameworks, these different threat intelligence databases, all of these different things.
So we're collecting all this data as the world's changing. And then Foo is just making it move at the speed of computers. So the point on messages being incomprehensible is infuriating to me, particularly because they could just be English or Farsi, right?
You could just write, "At 11:16 this morning a computer with the IP," like you could, there's nothing keeping you from just writing that in English, but instead the developer decided to save space or something, we're going to write and invent a whole new language. I'm not even going to write it in Klingon or Dothraki.
I'm just going to make a whole new language for this message format and tell nobody, right? And so you essentially have a situation to where the machine receiving the message, the log thing receiving the message, doesn't understand what was just said. It was gibberish. The person that's reading it on the machine doesn't understand what the thing says. So linguistically, why did we do this?
Martin: You do realize that if there's any WitFoo customers that are listening to this podcast, they're going to take this now as a personal challenge to make bells go off in your office by obscure formats. You're going to be getting Klingon, Dothraki, you're going to be getting Ferengi, you're going to be getting all these log files written in weird languages.
Charles: We do have it. I mean, they won't stump us. It's nothing new. Bring it, you know? And so I do love our WitFoo customers, our WitFoo kin as I call them. But they're a special kind of weird. Just to hang out with me as much as they have, you have to be weird at this point.
Now, we do have customers that, you know, are sort of out, right? They're purchasing through national resellers that are purchasing service SKUs from our service providers who are then purchasing our SKUs from distribution. So we're so far away from most of our customers that they don't know who I am.
They don't even know they're running WitFoo. It's just like the Intel chip inside of your computer. You didn't buy an Intel chip you bought, you know-
Jessica: Oh, okay. So your software is often a component of a comprehensive security solution, and it's the component that correlates possibly security related events with the same or similar things happening at many other organizations.
Charles: That's right, and sharing them. And it's also different. It's not just a security event. Sometimes there's other stories we need to tell, such as, how much money am I spending on security? Where can I save money? You know, I'm spending a million dollars a year on this firewall. Is it saving me more than a million? If it's not, maybe-
Jessica: Oh, like, is the firewall doing any good kind of thing?
Charles: Right. And same thing on the people, the people that were hired, do they have the tools? Were they able to do the jobs that we need them to do to perform response? So some use cases are, we're called the QuickBooks of cybersecurity.
So translating all of your machine data into return on investments on the different security tools and practices you've put in place. Also looking at where are you not compliant. So we have all of the data from everything. So we know this machine exists because the firewall told me it existed, but I have no telemetry about malware protection or identity or email protection. So this machine is vulnerable.
And either we have a regulatory reason such as PCI for processing cards, or HIPAA for healthcare, or CMMC for dealing with the federal government or just vendors. You promised a vendor you'd have some level of hygiene and you don't.
So we're giving that inventory to, that audience is typically the auditors, but we also have audiences with the chief financial officer that just wants to say, give me the readout. I'm giving you $5 million a year. What is it saving the business? You know, what would it happen if I took that from 5 million to 4 million? What would be the impact to the business?
And so just translating that--D ata tells beautiful stories. It's just the data has to be prepared to tell those stories, right? Most people aren't going to collect a terabyte or a petabyte of data in a day or a week to generate a spreadsheet on financial savings.
You know, if that's all that you were doing, that'd be expensive. But we're also doing things working with insurers. Like the thing you plug into your car, how safe are we? How good of a driver are we in cybersecurity? So while we're trying to establish what should be the premium, what should be the policy, and how much should things cost, how good should the package be?
We're providing that to them where you can just say, ship my reports to my insurance carrier. And same thing, if you have to pay a claim, the adjusters want to know, give me the data I need so that we can go try to find the people that did this so we can recover some of the losses and work with law enforcement.
But yeah, all of that to say that the connective tissue, both in information and just quantumly, is about the exchange of information. When we have efficient exchange of information between parties, the systems become predictable, that homeostasis occurs, right? Things become stabilized and safe.
When information's being lost and we don't understand what we're saying in any relationship, then things deteriorate. So that's sort of where we sit, is how can we take in all this data that's wildly confusing, but when it's translated into different stories, can bring people together because the things that's separating them is misunderstanding?
Jessica: Yes. So, your software processes terabytes and terabytes of these logs and then it communicates some of that back home.
Charles: That's right. So we have different, same thing in the way that we think about building anything else, we have small things you build into larger things, you know, atoms in a molecule, molecules into compounds, and so forth. So we start with the system ingest the signals, and then we take that signal, we preserve it in what we call an artifact.
And that artifact also has a schema. Everything we know about it in this JSON object, right? We rarely are shipping just artifacts all over the place, because that's the highest volume thing. When we're talking about terabytes or petabytes of data, that's the thing that's highest at the volume level.
We then take that data and it builds a graph of every relationship that those artifacts are describing. This computer just talked to this computer and when this user was logged in. So now you have three different objects or graph nodes and relationships between each one of those that we know exist.
And so as more artifacts come in, you start building this beautiful graph of millions and millions of different types of objects, of computers and files and URLs and emails and-
Jessica: And this is still within the customer?
Charles: It's still within the customer. So you have the graph, it's just like, this is our world, right? This is what's happening in our digital world. And so now we can do some object oriented analysis of that graph, right?
Look for patterns in the graph that look like the beginning of data theft, or look for extortion, right? Ransomware or trespassing via phishing, and create units of work out of those, right? So someone is trying to steal our data over here, they're based out of Eastern Europe. Someone's trying to commit ransomware, looks like they might be based out of Pennsylvania, right?
And so you start building these units of work and as the artifacts come in, they start supporting that crime theory, supporting the model, you follow the evidence, or they prove it's not true, right?
That actually wasn't data sets at all. That was a new backup service, right? And so you have these incidents which are just subsections or snapshots of the graph. And those incidents have injected into them as children, the artifacts that built them, right? Here's how we're showing our work.
Jessica: That's that provenance that lets it be evidence.
Charles: That lets it be evidence. Sort of think of it as like a case book, right? Here's everything we know about this particular potential crime, and then we're able to generate reports about the incident.
So show me all of the ransomware incidents that were automatically stopped by our firewall, or by CrowdStrike, or by whatever the tool is. And so now you're able to do business metrics because business metrics are fundamentally based on units of work, right?
So as a sales guy in the past, I had opportunities. So how many opportunities do I have? What's the deal? There's different characteristics for determining that. Uber has trips. You know, go to a restaurant, it's based on the size of the table, how many people are at the table, how many people do we need to staff? How much food do we need to bring in? It's all based off of these units.
And so business math comes off of those units. But we can also do cross-organizational campaigns. So we are able to work on one campaign where there was a criminal that was attacking small businesses and stealing between $20,000 and $300,000 worth of property or currency.
But the amount of money wasn't big enough for the FBI or Secret Service to come in and say, we're going to find these guys. It's just not enough money. There's not enough resources in law enforcement to do that. But because we started aggregating them together, so they're reporting these incidents at a service provider, we found out there were 37 different victim organizations and then the losses totaled several million dollars collectively.
So now you have sufficient evidence for the Bureau to come in and do the hard work of tracking these people down and putting handcuffs on them. And so we call those campaigns, right? Which are collections of incidents. And so essentially we have artifacts, we have incidents, we have reports, and we have campaigns, and then we have the graph so we can interrogate the graph.
And then the final thing we have is just intelligent sharing. Here's a bulletin. Be on the lookout for these things, right? These computers, these URLs, these types of emails, these types of files. And so when you receive those, you can search all of your artifacts and they'll create or update incidents, right?
And then you can request, in publishing it, if you find any of this, we would like to know, please call Charles Harring at WitFoo Research. We're trying to build a case. And they can push a button and automatically ship it to me to a different cluster.
So, all that to say, each individual cluster has providence of its data, but they're able to ship their data to another cluster in use cases where it's needed. So another example of that are ships at sea that need to coordinate with a shore operation center.
So they might have someone, the smartest higher level folks are on the shore because there's more room for that. And then the folks at sea are able to send their data to the ship. The ship's able to say, reset the password. The ship sees that command, the software sees that command, and then executes that job.
So it's not just sharing data, it also allows sharing of operations globally when and where that's appropriate.
Jessica: Which is a very, very distributed system. So, the next interesting topic is how your telemetry works. Like how you keep track of whether your software is working and how cheaply and-
Martin: Who watches the watchers?
Charles: That's right. To move fast, we have to have metrics, right? You have to know. And one of the disturbing things, I was working at another startup, about 10 years ago now, and I would come in, I was a systems engineer, and I would look at the logs on the system, and it had been broken sometime for months, right?
But there's no, that data that's sitting on the disc, and the log partition wasn't making it to me, right? Wasn't making it to our support. And so that was really concerning to me that you could have a system that's degraded or disabled, but the notification of that, it's sort of like if I fell down and had a heart attack right now, hopefully you guys would call 911 for me and say, I don't know exactly where he is, but we saw him fall down.
But if I'm alone, who's going to call, right? You know, it's one of those types of situations. So the nice thing that we've had, you know, we started research and development at WitFoo back in 2016, and a lot of great things have happened, right? With containerization, has made some of these use cases easier, right?
To where we can compile a docker image for running on Arm or running on AMD architecture, right? But you have that layer. So we have several layers because first we have the code, right? The code, if we say it's running in a JVM, or it's compiled C-level code, it's running in its own space. So we have metrics inside the code that need to be generated. So every class, every method we write is generating a metric.
Jessica: And do you use the word metric, where I would use the word event? It's a signal, definitely.
Charles: It's a signal in this measuring something, in this case. So it's saying the cycle time, the time to run this method was four nanoseconds, or 50 milliseconds or whatever. So there's generally numbers at that metric level that's telling us things, or how many messages were we able to process in the processing window? How much memory was used?
So it tends to be just a measurement. So that's one thing. We're also catching alerts or events, which would be error caught, right? We expected this, something unexpected just happened. And so we need to know the unexpected thing just happened. And so those are also kept, we transmit all of those metrics and, let me finish that up.
So we have the code itself, and then if it's running in a JVM virtual machine or something else that's external to the code that's handling heap, we need to have metrics on that thing. And then we also need to have metrics on the container, right? It's running inside of a docker container in our situation.
So what are the metrics for CPU utilization and disc utilization and all of that inside of the docker container? And then we have the instance those containers are running on. So we might have one instance that's running all of our containers, or it might be distributed where this is only running data and this one's only running input and this one's running UI, or whatever. But we need to know the mechanics of those instances.
So CPU, errors, all of those things, need to come at the instance level. And then we have the cluster, right? The customer's cluster. What's going on at the cluster level? And so that includes what's the user doing, how are the containers and the images connecting or not connecting with one another. And so at each layer of that Russian doll from hell, you have to have the right information being exhausted from it.
And so our first approach, philosophically, we knew that that was just too complicated for us to write alarm conditions at the very beginning, right? So we didn't say, if this gets too high, send us an alarm, or if this is this, plus this is too much, or the difference or the standard deviation. We didn't know what to write alarms for.
And there were so many conditions when we first started charting it out, I just said, forget all this, we're going to set all of the metrics up, we're going to put them on graphs, we're going to eyeball the graphs, and if something's weird, we write the alert for the weird, right?
And so it really accelerated what did we write things for because you know, when you start combining these systems, they also, the principle of complex systems, when you start bringing more systems together, they kill weakness that exists if you're only in a single system. Or another way to say that is, you can be sort of a horrible person in a small group maybe, but if you start working around a bigger group, the bigger group is not going to let you be a horrible person, right? Something's going to change.
Either you're going to leave the system or the behavior is going to leave you, right?
Jessica: Does that work with computers?
Charles: It does. It actually does. They actually will not boot up. They won't connect. So the things that fail, fail quickly. But the short of it is, the basic principle was send all the metrics, and then use a human that understands the system, that wrote the code to say, why is that cycle time so high? Why is CPU spiking, why is it taking so long for garbage collection?
And then we write checks against that collected data. So all these systems are collecting data, they're sending it up to a to WitFoo Library, WitFoo Library is storing it. And we typically store that for about a month and then just age that out. Most of the stuff ages out after a month. But we write checks for anything in the past that we knew was wrong.
So if we see errors, obviously we write alerts when we catch errors, there shouldn't be errors, or at least caught errors, for sure. And then we watch the metrics. Metrics have what we know to be the normal threshold. So we say, let's put the baseline here. We should expect to see this number on cycles per second, or messages per second, or users completing the flow, right? Whatever the metric is, we have some baseline of normal.
And then what we do is every 30 minutes we interrogate the data to say, in the previous window, and we have several hundred checks at this point, things to look for that the analysts would go look for weirdness. If any of them pop, that opens a ticket for us, captures the data, and then we go and investigate it. And where this became really cool is after we post, after we publish a release, right?
We've done all the testing, we've done our lab testing, we've written all the unit tests, we've written all the system tests, we've done the beta testing, and we push the thing, and then boom, the metrics say not good enough, right? When like, how? What did you miss?
Because this person's using a system that's logging in Unicode with a meg per message or something like what, how, what? And so now you're dealing with that, but I wouldn't have known to write a test for that until I saw it. So it allows us to sort of push the release beyond the release, right?
The release cycle goes beyond it and we're able to catch sort of instantly. So it's sort of funny to us. We never release, obviously no one should, on Friday afternoon, right? No one should do that.
Jessica: Oh, we always do. We totally push on Friday. Yeah, that's a thing at Honeycomb.
Charles: It's a great way to keep your adrenal glands strong. Just pushing on Friday goes nuts. Or maybe self care.
Jessica: I mean we watch it, we don't push it like, right before we go home, we look at what happened.
Charles: We do a tiered roll. So we do have the ability to put different deployments on different versions of code. And so we typically do a tiered release and normally have those in different buckets on the characteristics of the deployments.
But those metrics come in, we're able to, if we need to make a patch release potentially in an hour, you know, of the first release, right? Just push it through, go through all the system tests, unit tests, write the new check inside of the system tests to check for this thing that we didn't know to check for. And then it's caught earlier, right?
So it's about how can we push detection as early, shift as far left as possible, but also how can we catch the net new weird? And you know, for me, because I integrate with so many things, the variability of weird is so high. You know, I already have a high count of personas that use the system, but we have hundreds of things we integrate with and they're always changing. So they do a software code and we often get, you know, this API just broke because someone just released a new piece.
So catching the metrics and then having this idea of the developer checking the metrics as part of the release cycle, right? That I wrote this new feature, or this new method, or we tweaked it or whatever. Being able to go and look at a dashboard and say, okay, we expect the metrics to be in this range.
Jessica: And do they have a metric for their specific method or are they looking at high level?
Charles: Both, right? So we have the cumulative impacts. So, normally you're going to look at the impact.
Jessica: That's a lot of time series.
Charles: It is, but they age out quick. I mean, you have, for us, we probably receive about a hundred thousand different types of metrics per minute, but you know, it's not a billion. But that idea of metrics first has really helped us. And it also helped us reduce a lot of the way we test.
We do write code coverage tests and all that, but you just never know, right? You could write code coverage and have 100% code coverage and still miss 100% of the bugs. It's just the nature of things.
And you can write all these great system tests, but until you sort of have this complete collision of the unique hardware the customer's using, the unique data that's coming in from the customer, the unique processes from that customer, and all the variability of that, you really can't write the test. But it has really helped us, you know, and it also gives us great confidence, you know?
You can release a good, it is good that you can release on a Friday afternoon, which means you guys have great confidence in your ability to adapt, catch, and prevent deficiencies. And that's where observability comes into effect, right? Where if you know all the answers before you do the release, then you're in great shape. And if you know how to catch the unknowns.
Jessica: Right, right, the answers that you didn't know you needed.
Charles: Right. And hopefully it's not a big rewrite, you know, and also having a way to roll back. And that's another great thing about containerization is like, nevermind. Next Friday. Everybody go back to the old code. And being able to do that.
And one of the things, you know, by orchestrating product releases, and our customers can opt out of it, they can do manual release windows. But as a general rule, we'll publish when their release window is, and we manage that for them. And so we try to provide as much SaaS convenience as possible, but still maintaining the ability to deploy wherever it needs to be deployed, whether that is SaaS hardware or private public cloud.
Martin: So, my big question was, how do I get hold of this name and shame list of developers? I'm sorry, I'm still hooked up on that. I need the list.
Charles: It'll be anonymously posted to Pastebin at some point in the near future. Still ranking them. And I also, one thing I do want to do, if the business constraints weren't that bad, I would publish the top 10 worst log formats.
My favorite example is, there was a specific type of firewall that when it blocks a communication, it adds the string 'pattern=1' to the message log to let you know I blocked it. And so it's not action deny, it's not action block, it's just 'pattern=1.' And so you have to know 'pattern=1' means it was blocked.
And so I do want to write the worst ones. And it's also their standards, you know, that are associated with these things that are not adhered to. And sometimes, you know, it used to work for Cisco Systems, so I'll beat up on them a little bit. They wrote the RFC for NetFlow and they regularly disobey their own RFC, you know?
And so I'm like, well, who else do you go to here? And so it is all of this nuance, right? How do you handle it all? But I do wish we would get to a place and, you know, now that we've moved into, you know, NLP being as mature as it is now, you know, computers can understand English, right?
And so we can relax, or we should be able to relax, on how we write messages, right? We can just tell a story, we can write a paragraph. And that could be the message that a human could read, that a humanities major could read and understand what happened. And then I wouldn't have a job, but it would make the world spin better.
Martin: I mean, in IT, we're so good at writing stories, you know, user stories, we're so good at writing them, so we should just write all our logs in stories.
Charles: It is tough. I had a meeting with a dev team earlier this week. We're going through roadmap things and we're trying to name some things. And it's funny that when I'm coding, I can't name a variable. So like that part of my brain shuts down. The creative part that tells stories is compartmentalized.
And so normally when I'm writing big swaths of code, I pick a TV show and I name the modules after characters in that show. So, you know, I have this one big one that's after Battlestar Galactica, it's called the Caprica module.
Jessica: Please tell me you go back and give them meaningful, useful names later.
Charles: No, no, absolutely not. Somebody does. I don't.
Martin: I have people for that.
Charles: I define what they are. I define what a Starbuck is and what an Adama is, and this is the module that does this. But some of the things, you know, it's funny when you are naming it, it's like, I'm going to take this thing that's a blob, and turn it into, you know, a list of strings. And so, you know, 'blob the string of fire' would be the developer's name.
Jessica: That's a fine name.
Charles: But I do get sick of, and otherwise if I don't use something, and people do rename it. I like to say I write all of the prototype ugly code at WitFoo and then everyone else goes and fixes it.
Jessica: That is the CTO's privilege, yes.
Charles: Yeah, I was like, this is what it's supposed to do. Make that not horrible.
Jessica: So I have learned some fascinating things today about what happens with telemetry that I never knew about. And I am glad that WitFoo is out there checking these things and correlating them with other people.
Martin: I've met somebody that, when a data engineer says to me, data cleansing is hard, I can go, can I introduce you to Charles?
NCharles: Yeah, I am thinking about starting a support group for those folks that are having to deal with data cleansing, data sanitization. It is a nightmare. It's a complete nightmare. There's going to be drugs for it soon, I think. I think they're new pharmaceuticals are coming out just for data engineers. I appreciate the time. Thanks for having me very much.
Jessica: Great, thank you so much.
Content from the Library
Jamstack Radio Ep. #147, Secure Local Dev Environments with Chris Stolt and Ben Burkert of Anchor
In episode 147 of Jamstack Radio, Brian speaks with Chris Stolt and Ben Burkert of Anchor about securing local development...
Jamstack Radio Ep. #142, Decoupled Authorization with Alex Olivier and Emre Baran of Cerbos
In episode 142 of Jamstack Radio, Brian speaks with Alex Olivier and Emre Baran of Cerbos. This conversation explores tools for...
Jamstack Radio Ep. #140, Accelerating API Development with James Perkins of Unkey
In episode 140 of Jamstack Radio, Brian speaks with James Perkins of Unkey. This talk examines the difficulties developers face...