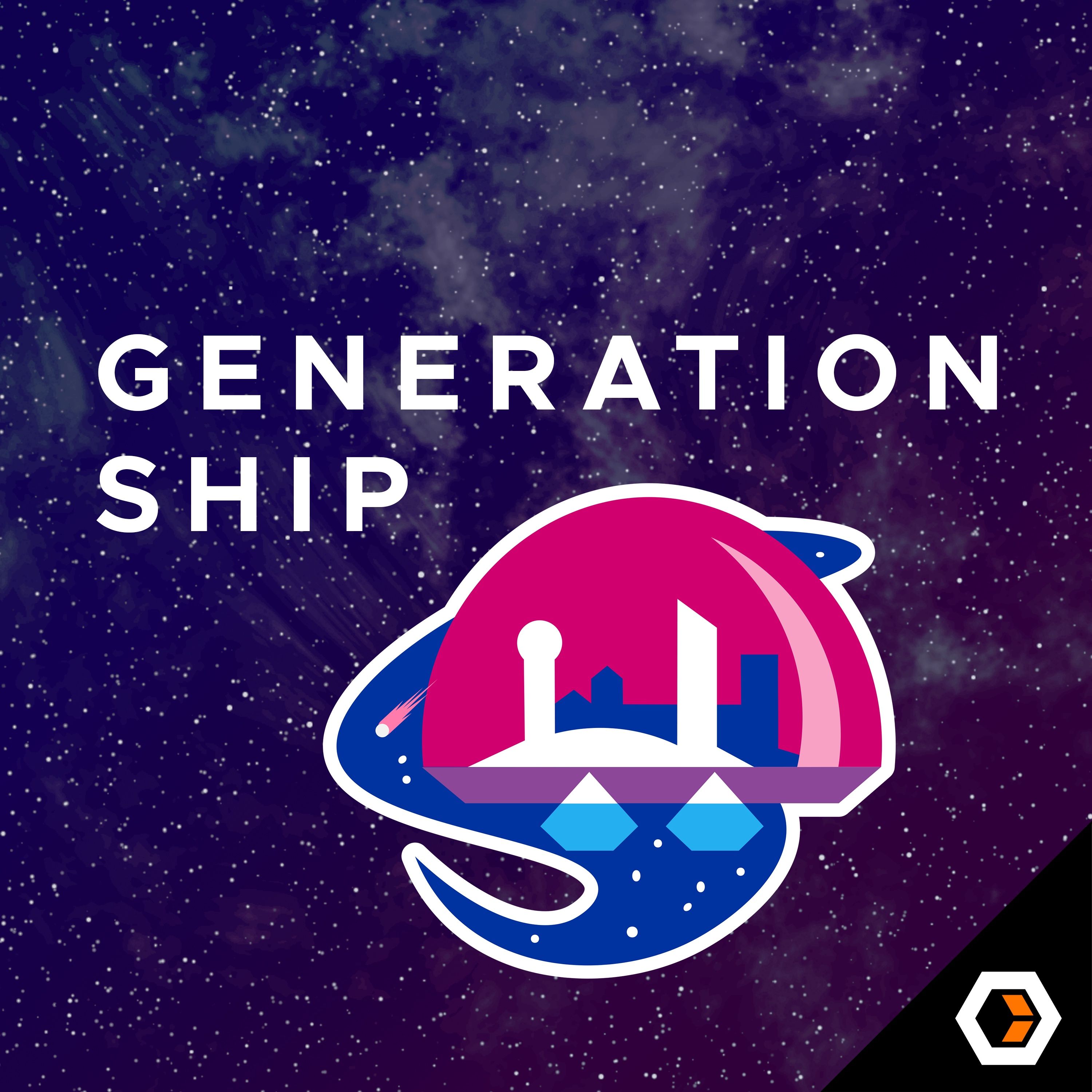
Ep. #1, Building Future Infrastructure Sustainably with Catharine Strauss
Generationship is a podcast exploring the intersection of infrastructure and artificial intelligence through a technical and philosophical lens. In this debut episode, host Rachel Chalmers is joined by special guest Catharine Strauss of Summerstir Solutions. Together they dive deep into the world of infrastructure capacity planning, sustainability in data centers, the future of AI in the industry, the importance of getting outdoors, and much more.
Catharine Strauss is the Founder of Summerstir Solutions, offering coaching services for engineering leadership and digital sustainability. She was previously a project manager at Google and Senior Manager of Infrastructure Capacity Planning at Fastly.
Generationship is a podcast exploring the intersection of infrastructure and artificial intelligence through a technical and philosophical lens. In this debut episode, host Rachel Chalmers is joined by special guest Catharine Strauss of Summerstir Solutions. Together they dive deep into the world of infrastructure capacity planning, sustainability in data centers, the future of AI in the industry, the importance of getting outdoors, and much more.
transcript
Rachel Chalmers: Today it's my pleasure to welcome a dear friend, Catharine Strauss. After an already distinguished career in infrastructure, Catharine spent seven years with Fastly where she rose through the ranks to become Senior Manager for Infrastructure Capacity Planning. Since leaving Fastly she's been recognized with a climate based fellowship, and today she provides coaching services for engineering leadership and digital sustainability through her own company, Summerstir.com. Catharine, it is a joy to have you on the show.
Catharine Strauss: Thanks so much.
Rachel: At Fastly you were responsible for this vast system of networks and servers that support gigantic traffic loads. Can you walk us through your day to day? What's it like? What tasks are involved in keeping Fastly up and running?
Catharine: Yeah. One of the major activities of the day was looking at the traffic over the past day, the past week, and identifying any operational problems we had, and looking over and over at what our peak capacity was, and what the utilization of that capacity was. So every day we're building more and more, we're trying to identify and prioritize what locations we need more resources at, and where we're running into constraints or bottlenecks that might give us trouble down the road.
Rachel: For people who maybe aren't as familiar with Fastly, can you tell us what a content distribution network is and how it works?
Catharine: Yeah. Essentially, if you have a file that you want to distribute out to a large audience at extremely low latency, Fastly will store that file for you and make sure that it gets to the destination as quickly as possible.
Rachel: So if I'm looking for the front page of the New York Times, instead of my request hitting a server in New York it can hit a server in San Francisco?
Catharine: It will hit the server that is closest to you, and so it's much more efficient for companies to use Fastly and compute the content that they want to distribute and then hand it off to Fastly to populate globally, as close to the edge of the network as possible.
Rachel: And how much internet traffic runs on CDNs, Content Distribution Networks, like Fastly? What percentage?
Catharine: I have no idea.
Rachel: It's something high though, isn't it?
Catharine: It is, it is. It's a tremendous amount of network traffic because it's essentially an augment to the already functioning systems, and key to Fastly being able to operate in that way is very good relationships with a wide variety of transit providers and peers in each data center. Having that distributed across many different providers and analyzing the traffic so that in each market they are connecting to the most commonly used networks by the end users who are trying to reach the services.
Rachel: So this is super interesting, you're talking about the literal backbone of the internet, the cables and the carriers and the enormous data centers that are hubs for regional networks. Is that right?
Catharine: Yeah, yeah. It's a very physical kind of job and one of the biggest challenges is you have to get physical items like servers and distribute them across the globe and someone has to physically install them. Then you have to power them, you have to cool them, and you have to connect them to the rest of the internet.
A lot of the way that I think about infrastructure is very similar to how you might think about an energy grid or an energy network. You have to build out the energy network so that you can meet peak demand when everybody starts their washing machine or their drier at the same time. But you are only charging for the every day usage, which may not hit the same peak every day.
So you're building to a higher level than hopefully you're sustaining, so that you've got room for outages or peaks in capacity that you weren't expecting. And so it's a lot of the same challenges that you see in areas like Texas during a heatwave, or other parts of the country as they struggle to keep up with increased energy demand.
Rachel: It's a really helpful analogy, and of course the two infrastructures are actually deeply connected because when there's a heatwave in Texas the energy authority is incented to pay Bitcoin miners, for example, to wind down operations. We often think of compute as abstract and ephemeral and intangible, but it's based on these very physical networks of silicon and metal and sand that are distributed across the world. It's easy for us to forget that because we only look at it through a browser.
Catharine: Yeah. As I've turned my eye more towards the sustainability of data center and cloud computing, I was surprised to find that up to 3% of total carbon emissions are generated by data centers and the machines in them. Water consumption is also a growing concern as you think about areas that need water for cooling the computing resources, but maybe it's in an area where water is at a premium and there's a blockage where municipalities don't want data centers in their areas any more because of these increased energy and water demands.
Rachel: Or we've also seen instances where municipalities, in order to attract data center infrastructure give water rights away at a severe discount which can impact local communities and their access to water as well.
Catharine: Yeah. That's definitely a concern.
Rachel: And as with energy networks, there's seasonality to internet traffic, isn't there? Another not necessarily intuitive thing, demand for bandwidth goes up and down over time.
Catharine: Yeah. That was the best part about being at Fastly and looking at the monthly analysis of the cadences. You'd see the daily peaks, you'd see how they varied on weekends, you'd see large events that were served by the CDN and you really got a sense of all of this human behavior, manifesting visually in a graph that's just going up and down every day.
Rachel: So a peak when people come in and log into their work computers and check Slack, and a peak in January when everybody is watching the Superbowl ads online. Are those some examples?
Catharine: Yeah, yeah. We monitored it very closely because our costs as a business was sometimes with our transit providers could be hundreds of thousands of dollars. You want to reduce your costs as much as possible while still relying on some of the more expensive data transit providers because they provide you the lowest latency, so you're constantly trying to balance those two values of cost and user experience, or really the product quality.
Rachel: It really is just a logistics challenge, like keeping retail stores stocked, making sure you have enough of the product that people are going to want on the shelves when they want it.
Catharine: Yeah, and spoilage is something that I don't think gets talked about often enough. We think about, "Oh, we bought too many sweaters and they were off trend and now they're going to go to a landfill."The version of that that you have in data centers is if you build out for an event and you only hit that peak capacity once and every other day you're at half that utilization, you really aren't making the best use of those resources.
Rachel: So what are some of the problems that you needed to solve? That's a good example, you don't want to build out for Superbowl because there's only one Superbowl a year. What are some of the other issues that you needed to troubleshoot in realtime?
Catharine: Yeah.
We would go through a process at the end of every month, a couple of days before the end of the month where we would look at our utilization on different transit providers and we would say, "Is there a network configuration change that we could make to reduce our bill that's going to come due at the end of the month?" And we would balance off various peers and various transit providers to try and blend the traffic into something that was slightly more cost effective for us.
That is a great opportunity for tools in AI to look at those different priorities of cost and performance and say, "Listen, what adjustments could we make on the macro or a micro level that would get us to a budget constraint or get us achieving a certain service level?"
Rachel: I love listening to you talk about this because you literally were a futures trader for internet data and, as a futures trader, you can already see some of the applications for AI, not only the large language models, but other AI techniques in this world of network planning. Can you talk about some of the places where you said that there may be applications?
Catharine: Yeah. There's obviously a lot in error handling and managing network outages where the systems can preload all of the possible routes that they could take to an open streaming connection. And if there's disruption below a certain quality level, you could automatically shift to one of the less preferred routes and the traffic still goes through. That has to be done very judiciously because if you aren't context aware of these changes happening and every AI system shifts everything onto a particular track then you've just moved your problem somewhere else.
So being context sensitive is probably one of the most powerful things, making sure that there's tons of feedback loops for what a particular change is projected to do to the network traffic and the network experience. Then also making sure that you adjust when new data is coming in from those links.
Rachel: So you don't want all of these AIs firing at once. That's an obvious risk. Can you talk about some of the less obvious risks of entrusting these critical network performance issues to what are, in a lot of cases, black box models?
Catharine: Yeah. I think the most obvious one is then if you don't understand why the decisions are being made by the system, then you've just added something new to your stack that you have to troubleshoot when something unanticipated happens.
I always often wonder are these AI systems like tax code, where it's a lot of rules and regulations that are meant to motivate a certain behavior and you have to weave your way through it to understand what's going on, or is it more like moving to a new country where there are new cultural mores and new rules and regulations, where you need to correctly evaluate what are the values of this system so that you could reasonably anticipate what is going to happen?
Rachel: Those are really insightful ways of describing what are probably two different kinds of AI. Certainly when we think about the large language models, the ChatGPT style of AI, it's more of the latter, isn't it? It's observed customs and rituals, whatever these tokens are they're derived from a big set of source data that isn't designed to incent behavior but reflects it, reflects human behavior, the entire corpus of the internet.
So a large language model is going to behave differently in the context you described from a tax code, it's not going to necessarily do what we want. It's going to do what we typically do. Is that potentially a risk when you're trying to harness these tools in production scenarios?
Catharine: Well, anybody who's sat through a retrospective after a large network outage knows that you really have to dig very deeply into what every individual actor was doing during the outage, so an AI system that is implementing network traffic in this way would need to be able to give you an answer to the question of, "And then what happened? And then what happened at this time?" So getting an output of a system event would actually be a large advantage of AI.
Rachel: Yeah, yeah. I can see how it would drop into things like post mortems pretty seamlessly. I think I still have some anxiety, I think a lot of people still have some anxiety about entrusting it with decisions when the answers aren't even necessarily consistent from one prompt to another.
Catharine: Yeah. The lack of it being predictable means that as a human trying to operate in that system you're not sure what behavior you should take. If you're looking at a self correcting network system and you're trying to decide, do I intervene and do I make a change, or do I just let it work itself out? You really have to put an enormous amount of trust into the system, that it is operating the way you designed it to.
Rachel: And historically, humans don't deal especially well with emergent behavior from system complexity. I read a lot about nuclear disasters because I'm lots of fun at parties, but when you look at Fukushima and Three Mile Island and Chernobyl, something that they have in common is that the operators were doing their best. They were trying to correct the emergent behavior of the system but their intuition about how the system interacted was way off base and lead to disaster.
I worry that the AI systems that we're manipulating are already beyond the point of complexity where a human can understand them. So again, very happy for them to take notes in meetings and write out the results of a post mortem, much less sure that I want them serving my traffic when I have important Superbowl ads that I need to watch.
Catharine: Yeah. When you look at the difference between a junior network engineer and a senior network engineer, a lot of times it comes down to the context that that person can bring to bear on the situation. So they know about that weird, little peer that spikes every Thursday at four PM. They know about the events that are coming through because they were in the meetings where they were being discussed.
Getting that context in place and giving a clear pathway for the AI to follow of, "If this, then check that, and then action." Dissecting that out of a fairly complicated ecosystem is going to be quite difficult.
Rachel: But I think what you're describing is a place where you have a human who's augmented by AI and the human still has the final say, but gets a lot of input and a lot of information and recommendations from the AI. Is that the model that you have in mind?
Catharine: What I have seen implemented is a lot of setting of thresholds on the macro level and then observing how those manifest at higher levels, for the whole system. So setting up what would normally be an alert to somebody on call will just program in what that person on call would typically do in that situation, and set a threshold for when that action takes place. Thinking very thoroughly about first, second, third order causal effects from that one change.
Rachel: Here at Generationship we are very much on the side of letting SREs sleep more, so that sounds like a really good model. What advice might you offer to infrastructure professionals as they start thinking about how to incorporate some of the existing AI tools into their work?
Catharine: I think one of the important things is to make sure that you're being honest about what the goals are of your organization, and balancing those, and asking really good questions. So for the example that I gave before, we were talking about trying to minimize costs, which is good until it starts to impact performance.
A lot of decisions come in those trade offs between competing goals like pushing a new service out that maybe has a higher level of errors, that is something that you have to give the AI context in order to correctly assess.
It's very difficult to track all of those so communicating as much as possible about what the values are and what standards are in place for teams that are working together so that teams know what to expect from each other. In the same way that you would list out the service level agreements that an operations team has to the rest of the organization, you would implement that so that people know what to expect from these automated systems.
Rachel: That feels like a very deep insight to me and ties back to a little of what we were talking about earlier, about the trade offs. An organization exists to fulfill goals, it has certain values, and the humans who work in that organization are optimizing towards certain outcomes based on those constraints.
When we shift gears and start to think about sustainability, we can think about the population of Earth as an organization and we're trying to optimize towards the goal of everyone having enough to eat and enough water to drink, and one of the underrepresented risks of AI is just the sheer amount of infrastructure that's going to be required as these models are enormously GPU intensive.
They're enormously compute intensive. Can you talk about your career shift towards sustainability and how you're thinking about building out the infrastructure of the future so that we have some Earth to live on? I like Earth, it's where I keep my stuff.
Catharine: Yeah. I was kind of alarmed when I saw some of the early figures on training AI models. One of them said that it was something like 626,000 kWh which is about the emissions of five cars worth of carbon. It can be tremendously large, and so it has to come at a trade off. It has to take the sustainability load onto itself, just as a table stakes kind of thing. So, that being said, I think AI has this tremendous ability to help us implement our values around sustainability in a more rigorous way.
So if you code an AI to say that in all cases use the lowest carbon data center resources available, or operate at a time of day when you know that you're going to be on solar power, that's hard coded. That doesn't get the sort of flexibility and the wobbliness that humans can sometimes have with their values.
Much of the work that needs to be done as far as data centers and their energy consumption is going to be done by the acceleration in solar power adoption, and that's true in both the US and China.
We're making really, really fast progress but this is going to alter the ways that we use our energy networks because it's going to be unlimited solar power during the day and then the choice at night of whether or not you're going to fire up the coal plant in order to provide additional energy capacity.
Rachel: Or in China, this new generation of nuclear power plants, which this generation will definitely never meltdown. Totally safe this time around.
Catharine: I hear some skepticism in your voice here and I think, yeah, we're both on the same page with that. So if you're looking at overall energy consumption for data centers, all on this same kind of curve that we've mentioned where you've got this daily utilization, what you want is to have it match energy availability as much as possible. Hopefully, at some point in your calculus you're also taking out the energy that humans tend to use to operate their homes and their air conditioning and all of the other things that we use electricity for.
So that is a large modification from the current daily utilization which, on the network at least, tends to spike in the evenings when people go home and start their streaming services. How can we use AI to take nonessential load off of these data centers and these electricity grids during the peak times and shift it into lower utilization times of day so that there's more capacity?
Like I said, if you have to build to your peak loads and you could reduce those peak loads through load shifting, then you don't have to build out as much infrastructure. It's cheaper for everybody, it's more sustainable for everybody.
Rachel: We can remove some of that extra headroom if we have better algorithms to predict demand and to match demand to supply?
Catharine: Match demand to supply and start asking good questions of our software engineers about is this piece of workload, does it need to be done now? Is it latency dependent? Does it need to be stored forever? And just really start to ask these hard questions, because we've gotten used to an always on, always available, always lightning fast internet experience. Sometimes it's over delivering what we need to survive, so is there a way that we can scale back our expectations to what we actually need?
When I think about this, I often think of a talk that Ben Treynor of Google gave about the early days of SRE, they went through and they realized that they were over delivering on latency. They were delivering search results faster than people could really observe. There was zero latency, so they built into the budget a slower response time to the user.
The user did not notice it was below the physical capacity to observe a delay in the result, and from that time they built in a maintenance budget. They built in time to build and expand the network because they didn't need it to be the fastest thing out there.
Rachel: It was such a revolutionary insight. I remember the first time I was reading about the error budget and it was like, "Yeah, sometimes you optimize so far that the difference between 98% and 99.9% is imperceptible to humans, so what are you spending on all that energy on? Wouldn't that energy be better spent elsewhere?" That's exactly what you're talking about, isn't it?
Catharine: Yes, yes. Well put. So are there opportunities for us to do that?
We have a tremendous amount of information on more efficient, sustainable choices in our computing. There's electricity maps now for data centers, there's a lot of tools out there that will tell you what the energy sources are for a particular data center. At Fastly we would move traffic around, move load around occasionally for upgrades and it really gave me this awareness that for the majority of cases, where your load is computed is not important.
It's the delivery that matters. So are there opportunities to move the load to greener data centers and take a latency hit, but still manage to delight your customers? The Green Software Foundation has a carbon aware SDK that can be implemented by programmers.
There's a lot of different tools out there and the ones that are just databases and not practices seem like a really good candidate to build in rules and guidelines for carbon safety, if you want to call it that, that an AI would use in their decision making framework.
Rachel: Super cool. A couple of wild card questions, forgive me but you'll enjoy these. You have been made God Emperor Of The World, your powers are unlimited. In five years, what does the world look like? How has it changed?
Catharine: Everyone spends a lot more time outside.
Rachel: Oh, I love that.
Catharine: Yeah. I think we really spend so much time in the valley of our minds and in optimizing and in trying to think harder and better and faster, and we forget that one of life's great pleasures is to just exist as a body outside. There are so many delights to be had, and I want people to think more deliberately about the trade offs they are making when they go all in on their digital life at the expense of their physical body.
Rachel: So I agree so hard, and I actually blame Renee Descartes for this. Mind-body dualism enters history with him, and you see this drive towards abstraction, this drive towards the separation of the intellectual world from the physical world, and I think it's had serious downsides. I think we think of ourselves, particularly in Silicon Valley as you say, as brains in a jar, when in fact we're embodied minds.
When we take care of our bodies, when we take care of our physical selves, when we connect to our ecosystem we make much better decisions about, for example, the distribution of resources. Another wildcard question for you, what do you see happening for yourself in the next five years? You've got Summerstir, you're coaching some of these amazing emerging leaders. How would you like that to pan out for you?
Catharine: Yeah. I'd really like to be able to join up with other professionals in computing who are taking this cause of sustainability up. There is so much that people in Silicon Valley, in digital careers, in operations careers who work in and for these data centers can do to slowly start to creep businesses towards a more thorough awareness of the impact of their operations on the globe. People spend a lot of time either despairing about climate change or deciding just to not think about it because they've got their job to do.
What if every day you went into your job and you tried to find one thing that you could do as part of an organization? There are plenty of people who will stop eating meat, or stop taking flights, international flights because they want to work on climate change. But we underestimate the power that we have inside our job functions to slowly influence companies which have a much larger impact on climate change than any single individual will.
Rachel: That's inspiring. That's a liberatory vision. Catharine, if people want to catch up with you and follow you, what are some ways for them to connect with you online?
Catharine: So you can reach me on my website at Summerstir.com. Also I'm active in the Slack group ClimateAction.tech, which has a ton of people who have come to the same realization that I have, that they too can start to work on climate and climate change in their professional role as a technologist.
Rachel: Catharine, it's always a delight to talk to you. Thank you so much for coming on the show.
Catharine: Thank you. This has been a really wonderful conversation, and I look forward to hearing your other guests and what they have to say.
Rachel: You know where to subscribe.
Content from the Library
Regulation & Copyrights: Do They Work for AI & Open Source?
Emerging Questions in Global Regulation for AI and Open Source The 46th President of the United States issued an executive order...
The Role of Synthetic Data in AI/ML Programs in Software
Why Synthetic Data Matters for Software Running AI in production requires a great deal of data to feed to models. Reddit is now...
Data Council 2025: The Databases Track with Sai Krishna Srirampur and Craig Kerstiens
Heavybit is thrilled to be sponsoring Data Council 2025, and we invite you to join us in Oakland from Apr 22-24 to experience 3...