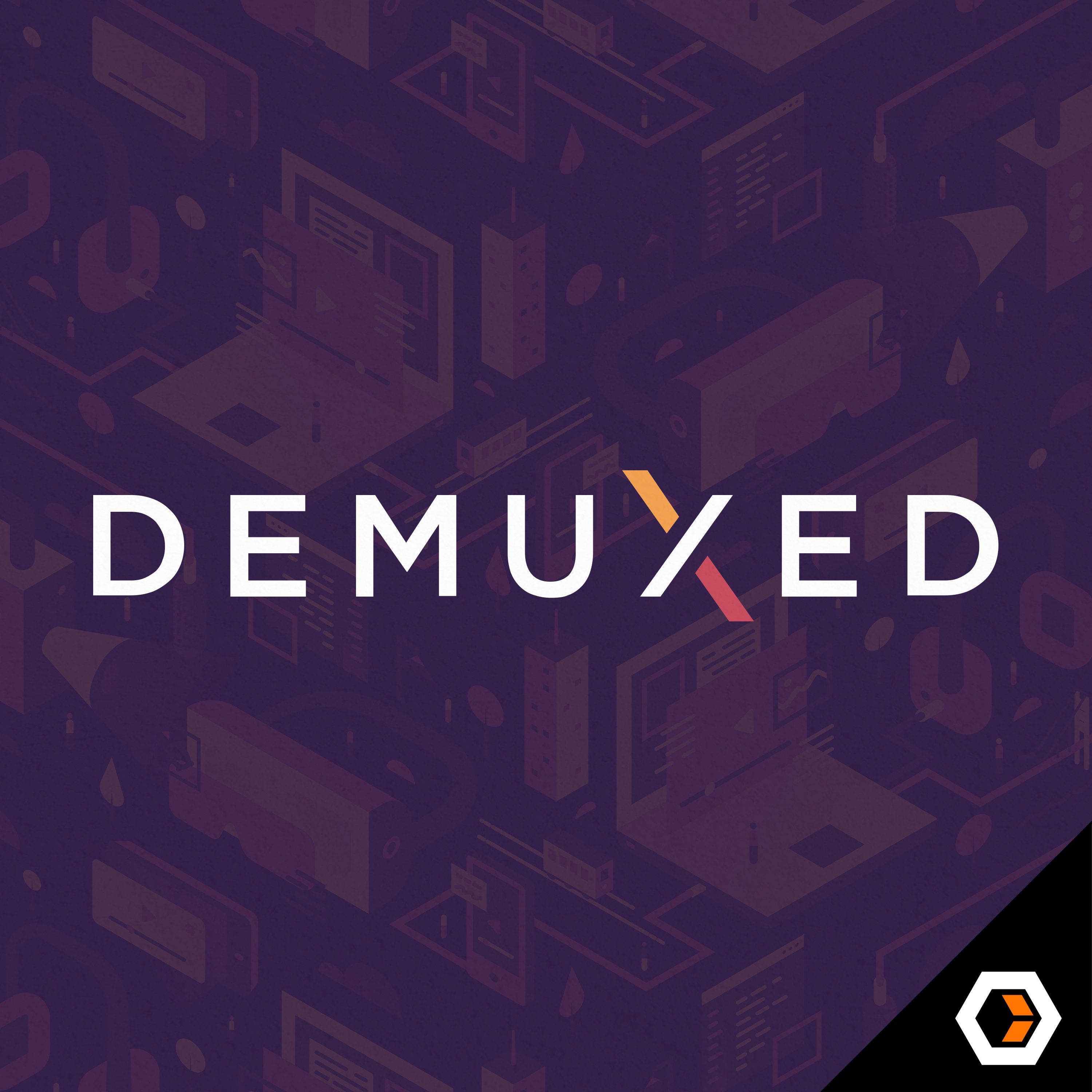
Ep. #18, AI Powered Video Editing with Anastasis Germanidis of RunwayML
In episode 18 of Demuxed, Matt, Phil, and Heff are joined by Anastasis Germanidis of RunwayML. They unpack AI powered video editing tools in the browser and share many invaluable insights gained from the machine learning community.
Anastasis Germanidis is Co-Founder & CTO of RunwayML, a platform for artists to use machine learning tools in intuitive ways without any coding experience for media ranging from video, audio, to text.
In episode 18 of Demuxed, Matt, Phil, and Heff are joined by Anastasis Germanidis of RunwayML. They unpack AI powered video editing tools in the browser and share many invaluable insights gained from the machine learning community.
transcript
Matt McClure: Hey, everybody.
Welcome to the Demuxed podcast.
Unfortunately, this is only the second podcast of the year.
The year of the podcast hasn't gone quite as planned, but we are looking to record more of these.
So, I just want to say it up front, if you or someone you know would be an interesting guest, reach out.
We'd love to chat with you.
Today we have an awesome guest, Anastasis from RunwayML, and we're going to be talking about awesome machine learning, video editing, all that sort of stuff in the browser.
It's great. Before we jump in, just some quick updates around Demuxed.
So, you've probably seen the news at this point that we've gone online only.
So, we were always planning on doing this hybrid event.
Last year went really, really, really well.
People really appreciated the online event that we put together for 2020, which we were humbled by.
And we really appreciate everybody coming together for a new and different experience.
And so this year we wanted to maintain that, especially given the travel situation for a lot of the folks, especially our friends in Europe and even domestically.
There's a lot of fear and concern and safety issues.
So, we wanted to make sure that everybody could attend.
And so we always wanted to do this as really owning that online, making sure that we were really investing and embracing that online side of things and also having people in person so we could see each other.
And unfortunately, the in-person thing just isn't going to happen this year.
Steve Heffernan: It's just going to make the online one even better.
Matt: Yeah, yeah. We're just doubling down our efforts and really focusing.
We went transparently went back and forth on what this should look like.
And really what we ended up coming down to is we were having to plan two events anyway, having to think about that online only, what that looked like for in-person, what we were going to do if in-person had to go, and especially given all the uncertainty.
People were confidentially coming to us with just general concerns and it felt better just to take a step back, really embrace going online again this year and putting together the best event we possibly can for as many folks as we can.
So, dates, if you haven't seen them yet. October 5th through 7th online.
Tickets on tickets@demuxed.com. The ticket ordering is going to be much more normal this year.
So, we're saving ourselves a bunch of time and effort around trying to figure out how the--
Never again on the donation-based ticket. I really appreciate everybody that donated more.
That was awesome. We were able to raise a ton of money for great charities, but it made everything else about the ticket process, managing and buying, just awful.
So, flat rate, 49 bucks to come.
Phil Cluff: Where are we in the selection process at the moment, Matt?
Matt: At this point in time, we've gotten all of the submissions in. Submissions are closed.
And now we're going through this full anonymous review cycle, which is a really critical part of this.
Phil, do you want to talk through how all of this works from here?
Phil: Yeah. Once we got all submissions in, we asked someone independent to redact them.
So, that removes anything that identifies a company someone works for, gender, race, all those sorts of things that might introduce bias into a review process.
All those redacted submissions then get sent to a committee.
We have this great little web app.
I say that because I built it, not because it's horrible or anything, but we have this great web app that people log into and get shown random submissions in a random order that are fully redacted.
And people review them on a scale and look at things like, are they original, is it possible to cover this amount of information in the time allowed, all those sorts of things, and give us just a general rating, as well.
And we collect all that data, run a bunch of statistics on it and effectively produce a list of talks.
I think this year we invited the largest committee we ever have done.
So, the initial committee was, I think we sent out 35 invites across the video tech community.
Across the world, as well. There's people in there in Europe, Australia, America, obviously, Southern America as well.
And of those, I think we had well over 2000 reviews this year.
At one point I glanced at it and it was 2048. I did wonder if something had broken. It's true.
I was like, "Oh, is this? Okay, no, it's fine. We just literally do have 2048 reviews in that exact moment."
And yeah, a lot of people get through all of reviews as well.
So, do review every single talk, and that is massively appreciated.
And we invite anyone who reviews 70% or higher, the bar is usually, to come and participate in a final selection committee where we then look at the talks, ordered, we categorize them, still retaining anonymity through most of this process, and pick, effectively, the best talks from each subject area.
And then we'll start to build a schedule around that. It's really exciting. It's one of my favorite things to do every year, get some pizza and really get stuck into it.
And it's this Sunday.
Steve: So, without saying any of the top titles, how excited are you with what you're seeing compared to previous years?
Phil: So, typically Matt, myself and Heff, we don't actually vote.
We don't participate in the initial review process.
It wouldn't be fair if we did, because a lot of the times we've seen them coming in.
And in some cases we've actually given feedback on the talks as well. I am pumped.
I really am pumped. I was looking down the top 30 talks the other night and there isn't a talk in there I'm not desperate to see.
So, I'm genuinely really happy. I don't want to say it, but I'll say it.
I think it might be the best top 30 talk submissions I've ever seen, genuinely.
Matt: Yeah. It's really good. The things above the fold this year are--
And by fold, I mean, typically basically we pick a cutoff point in those anonymous reviews, like Phil mentioned, and anything below that doesn't typically make it to the committee.
There some exceptions.
We will put our finger on the scales sometimes in the sense of we'll know a little bit more about the speaker or the topic or whatever else that might mean that it's like, "This is something that we need to cover this year, and actually this one below the fold could be good."
And so we'll at least pose that to the committee oftentimes.
So, it's not a lost cause for the things below, but it makes it tougher.
And the things above that fold are just, top to bottom, really, really good. Okay.
So, anyway, October 5th through 7th online. Tickets at demuxed.com.
See you there. So, if you came to SF Video last month, Anastasis gave a talk about RunwayML and what they're doing there.
So, if you came to that talk, you're already familiar with some of what they do.
If you want to check it out, it's on youtube.com/sfvideotechnology. I think that just works. Anyway.
Welcome to the podcast, Anastasis. Thanks for joining us.
Anastasis Germanidis: Thank you for having me.
Matt: Yeah. Why don't you tell us a little about yourself?
Anastasis: Yeah, absolutely. So, I'm a co-founder and CTO at Runway.
We built a video editing tool on the web that's powered by machine learning technologies.
So, the basic idea of what we're doing is to take a lot of existing workflows around video editing, and then using the latest in computer vision and computer graphics, transform some of the process that video editors are used to, and introduce new workflows that are just becoming possible with new techniques that are emerging from research.
A lot of what we do is we solve a lot of challenges that span across cutting-edge machine learning research, video engineering challenges of making this thing work on the web, on the browser, which it's just become recently possible to do with some of the emerging web standards around video decoding on the browser.
And also building a really complex application that includes both video editing--
Normally you have video editing primitives that people are used to from existing video editing software, but also introducing new kinds of functionality that wasn't possible before.
So, one example of that is a Green Screen.
This is a tool that we released a few months ago and the goal of Green Screen is to solve the rotoscoping process.
So, rotoscoping, for those who are not familiar, is the process of masking out the subject from the background in a video.
That process is traditionally really labor-intensive.
You have to go through each frame and then manually mask out the subject tracing with splines.
And we do that with a few clicks. So, you can select your subject and track the subject through the video.
And we do that through a combination of machine learning models.
But this is just the beginning of what we're working on.
We're working on some more extensive video editing functionality, automating some parts around sound editing and environmental sound creation to removing certain objects and video to other functionality that's coming up.
Steve: Yeah, that's really awesome.
Tell us about the journey to get to where you guys are today.
Anastasis: Yeah, so it's been a long journey. So, the company started around three years ago.
All of us co-founders, we were three of us, we met at a Master's program at NYU called ITP, or Interactive Telecommunications Program, which is not exactly the most descriptive name for a program in the world.
But basically the goal of the program was to explore the recently possible, and so emerging technologies like machine learning or AR/VR.
The goal was to play fully engaged with those technologies, create projects that try to imagine new interface around those or critically investigate them and understand how they're going to be used in the future.
And me, Chris and Alejandro, the two other co-founders, were bonded over our interest in ML and our interest in bringing ML to creatives and building tools that make it a lot faster to work with ML, especially if you don't have the technical background to use those technologies.
So right now, and this is getting better over time, but to use machine learning requires a lot of technical know-how that people coming from the creative domains, if I'm a filmmaker or an architect or illustrator-- A lot of those technologies are very relevant to what I do.
So, we have techniques for synthesizing images, which an architect can train on their architectural plans or an illustrator can train on their illustration, but they're really hard to use.
And that's the problem we set out to solve initially.
So, Runway came out of Chris's, my co-founder's, thesis project.
And initially it was, we built a platform that made it really easy to use open source machine learnings through a visual interface.
So, creatives would upload their footage or their assets, and then have them processed by open source machine learning models without having to write a single line of code.
And after that, we also built out the training functionality so you could also train an image synthesis model.
So, you could upload 100 images or 1000 images and how they're modeled can generate infinite more based on those, as examples.
Slowly we realized that video was becoming one of the main use cases in the platform.
So, we didn't really have good support for video initially and it wasn't really a focus of the product, but we saw our users working with video in all kinds of ways.
So, we had the depth estimation model where people could just upload a video, process it, predict the depth and then bring it back to something like after effects or resolve, and then use that depth information to enhance their effects in their video, or get automatically a segmentation mask or the optical flow of a video.
And what we realized was that even though we had that ability to do that inside the model directory that we built, it wasn't easy to do, and it wasn't what the product was originally meant for.
And so we set out to build a tool that's specifically for video editing, and that's become the focus of the company in the past year.
Steve: That's great. So, whereas before they had to pull this information into after effects or something, now they can do it directly in the browser in your application. Is that right?
Anastasis: Right.
So, we basically had the very generic interface that allowed the interacting with all kinds of models, where you just have any good image and you get an output image.
But this is not necessarily suited for giving you control over how the output would look or allowing this more extensive editing aspect of it.
So, it was more like you could use Runway to process your assets, but you couldn't have any control over how the final result would look.
So, what we started to build was a more interactive tool kit that allowed you to, for example, to mask the subject, you don't just input your video and then get a mask with the foreground and the most prominent subject in the footage.
But rather you could pick what you wanted to segment and you could add it to the results that were coming out of the model.
And with that, that becomes from something that's just a utility to something that people will spend a lot of effort in and spend a lot of time with.
Steve: That's really cool.
How much of this has been applying user interfaces to existing machine learning capabilities and how much of it has been actually you all needing to evolve the machine learning capabilities itself?
Anastasis: It's a combination of both.
So, a lot of machine learning research tends to be not really well thought-out in terms of user interaction.
So, what we set out to build was on the one hand, improving their efficiency and accuracy of the models to better fit the kinds of videos that users were uploading or the level of accuracy that they were expecting.
But on the other hand was also introducing this human-in-the-loop aspect, which is a big part of the research that we do at Runway.
So, if I take backend model, for example, you have models that basically automatically separate foreground and background, or you have models that can automatically segment from a number of fixed categories, but you don't have as much research on how to involve the user in this interactive loop.
And so in some cases, this can be an afterthought in the way you develop the model, but it tends to be better when you involve the user input and the human guidance in the training of the model.
So, you incorporate that input as one additional input to the model beyond just the frame content that you provide.
Steve: That makes sense.
Matt: This is a specifically interesting topic for this podcast specifically because we typically focus so much more on the video technology side of things, and very rarely do we get into production.
And this is a really fascinating combination of production and that side of things that we typically don't touch on at all, and deep video tech and weird emerging ML and all this sort of stuff.
So, I think this is fascinating, I'm curious, not to get too far into the product side of things, but as you're thinking about the people that are using this thing, they're starting to play with it and experience it, are you seeing more uptick with the folks that don't come from this background, that aren't already living in Premiere?
How much of this is opening up the quality production and doing interesting things with video on that side of things to a larger audience versus one more tool in the tool set of your power users already?
Anastasis: Yeah. That's a good question.
The way we see it is there is a spectrum of video editing tools ranging from casual video creation, which is something like mobile apps that basically let you do something like simple video editing, maybe add some simple effects.
And then on the other hand, it's very powerful video effects tool, very low level.
One example is Nuke, from that side of things.
And also, After Effects is a bit easier to use than something like Nuke, but it also requires a lot of knowledge, a lot of training to get to understand how the interface works, to understand how to use it on a daily basis.
We are positioned in the middle.
But a lot of what we're trying to do is bring a lot of the functionality that takes a lot of effort and a lot of knowledge to doing something like after effects and make it really easy to do for video editors who don't necessarily want to spend the time to learn the secret arts of the effects.
And so the goal of Runway is to make it very easy to do really magical, complicated video effects things without necessarily needing to spend a lot of time, spend a lot of effort making those happen.
Matt: Yeah. There's a piece of this where it's like democratizing video editing, to some extent.
Anastasis: And also from the other side of things, we also want to become an entry point for folks who are doing some video creation but in a more casual capacity, by having a more easy to use and more inviting interface, make it possible for them to be introduced to some of those more advanced capabilities that they might not even know existed.
So, for rotoscoping, for example, this is a process that very often requires actually outsourcing and hiring a whole team to do that process.
And the moment you can do it automatically and iterate very fast on it, it also allows improving, when working with clients, to make possible to make quick edits, send them to the client, get some feedback, then make additional edits.
So, for people who are not coming from the VFX world, it allows them to move faster with an approach instead of working with external clients.
Steve: Yeah. How long until we see this type of tool exposed to somebody creating a TikTok video directly on their mobile device and just trying to record it there and upload it right back?
Anastasis: Yeah. That's a process we constantly are trying to simplify.
TikTok, as an example, provides a lot of editing functionality built in.
But if you're a professional TikTok creator you usually end up using something like After Effects or a combination of After Effects and Premiere.
And we want to build something that allows you to take advantage of the collaboration aspects of the web to make it very easy to create new projects and also share them with other people through the interface of Runway.
Steve: Thanks.
Matt: So, I think you answered this somewhat tangentially in that, but at first blush it would appear to me that you added a lot on your plate by embracing the browser being your primary interface. Yeah. Why?
Anastasis: We think the browser allows a level of collaboration that it's really hard to achieve with a more desktop-based tool.
That's the first point. The second point is that we are entirely, in terms of how we process assets and we apply machine learning, we're entirely cloud-based.
So, in a sense, by not being tied to the user's local compute, that's a big part of our mission to democratize some of those technologies that require normally having access to really high end consumer GPUs.
And on the other hand, it also allows us to say, if you want to have a really long rendering job, we can scale up, like spin up a lot of GPUs to process that task specifically, and then scale down.
And this kind of elasticity is really hard to achieve if you're working with very fixed number of compute that you already own.
So, by being cloud-based, the web felt like the natural fit.
Being on the web allowed us to achieve the goals of making it easy to collaborate on videos and video projects.
And at the same time, it was a natural feed for the way we process everything on the cloud and allowed us to scale our infrastructure faster.
Also, try more experiments, being able to push new versions of the app, try out how people would use new models that were released and our iteration speed was faster.
And also, it was a natural fit for processing things on the cloud.
Matt: Getting a little more tactical, I'd love to hear weirdest issue you've run into so far.
I mean, you're talking to a group of people that have spent a lot of time working with video in the browser and there's some rabbit holes in there.
So, I have to assume, in the work you're doing, you've seen some shit.
So, what does that look like?
Anastasis: Yeah. I'll be frank. I'm not a video engineer in any way. I had to learn.
I went through video streaming, video compression 101 when we decided to build those video tools because I had to.
So, I was thrown into that world and it was a lot more complex than I imagined originally, I have to say.
Phil: Welcome to everyone ever learning video's experience. "Oh, this is hard."
Anastasis: The labyrinth, the maze of possible formats, of possible ways.
Even expectations you have as a user of when, a time-stamped video, every player would play a specific video in the same way and would display the frames at the same time.
Even those expectations were not-- Everything seemed more complicated than I imagined.
I didn't realize that frame rate is not a real thing, for example. So-
Phil: "Every video is technically a variable frame rate," as someone very close to us says.
Matt: Exactly. Yeah.
Anastasis: So, as you brought up, we're on the video production side of things, and in some ways what we're doing is a super set of, we have to solve both the playback and consumption problems, but also an additional set of challenges.
So, for example, building a video editor, it's not just playing the video from beginning to end or streaming it.
It's, you have to account for user experience when the user is constantly syncing forwards and backwards and you need to make syncing to be really fast.
And you also need to account for having multiple clips in the timeline.
If you're building a professional video editing tool, you need to make sure that the timing of those clips is perfect. What we realized very early on was that existing obstructions on the web for video playbook were not a good fit for what we were doing. So, something like the video element, an HTML file video element, did not have precise timing if you wanted to display multiple clips at once.
So, that was one of the basic problems that we faced very early on when we were building Green Screen.
We needed to show the mask that was coming from the machine learning model at the same time as the content.
And even if there was a 30 millisecond delay between the two, the whole experience is ruined for the user.
And because of that, we basically decided very early on to build our own decoding logic on the browser and not rely on the HTML5 video element or media source extensions.
And that was a whole journey. That, again, was another display of how complicated it would be.
We initially just shipped a WASM H.264 that was doing that work.
We faced a lot of runtime issues where it was just not as fast, obviously, as the codex that shipped with the browser.
So, that's how we encountered WebCodecs, and WebCodecs seemingly solved all of our problems.
The main issue was that it was very early in its development.
It's still on origin trial. So, things are constantly moving.
There were some issues around incompatibilities between different operating systems in the way, for example, memory copies of frames are handled.
So, small issues like that. But overall, our experience with WebCodecs was that it allowed us to achieve things on the browser that we couldn't before.
And it's a much better solution than shipping your own codec as part of the app, which is also bandwidth intensive, it's conversational intensive, and so on.
Phil: So, for those of our viewers who are maybe not as much of an expert as we all are, could you give us a 30-second overview of what WebCodecs are in comparison to media source extensions?
Anastasis: Yeah, of course.
The way I like to described WebCodecs, and maybe this is not the most accurate description, but it's basically F Fmpeg in the browser with the codex that the browser is already shipped with.
So, you have access to any codec that the browser already supports when you play video in a video element or with media source extensions, you can use via WebCodecs, but you also have control over how you're doing the decoding.
So, you can implement logic for, say, buffering in a more custom way for your own use cases.
Or if you're playing multiple videos at once, which is something that we're doing on our editor, you need to make sure that you're displaying the correct frame timing-wise from each of those videos as you're playing through a timeline.
And this can only be achieved with WebCodecs because exactly which frame you're decoding at a given point, unlike with media source extensions or with video elements where each of the elements that you may be managing has its own buffering logic, and you don't have any control over how far ahead you're buffering, things like that, that basically make it really hard to make a performance critical application.
Phil: Amazing. And I think you mentioned it, but WebCodecs are currently still in an origin trial.
So, how many people have you got WebCodecs turned on for, at this point?
Anastasis: Right now we have enabled WebCodecs for all our users.
So, we have a set of different video tools and for our main video editor that we released recently, it's called Sequel, we only use WebCodecs at this point.
The performance difference is so big that it was a natural choice.
Of course, we keep an open ear of updates within the WebCodecs world.
It seems like from what I've read, that it's going to be shipped and stable in Chrome very soon, in which case it seems like we're going to be continuing with WebCodecs.
But if plans change, then we will fall back to around Wiseman decoder solution.
Phil: Nice. Super cool. And is there an intent to implement across the rest of the browser world, or do we think it's only going to be a Chrome-only thing for a while?
Anastasis: I know that Firefox and Edge have declared that they're planning to implement it.
There is a lot of back and forth as usual with every web standard, some debates around whether to only run in the web workers or not, things like that.
But overall it seems the response from both Firefox and Edge is positive.
Phil: That's a fascinating topic because I got down a bit of a rabbit hole a couple of nights ago understanding the performance differences of Canvas when it's on the main thread versus in a worker.
And I presume that the exact same thing is true of WebCodecs.
Anastasis: Right.
There is a hundred common thread in the WebCodecs standards repo, going back and forth to different arguments of whether to only allow WebCodecs on the worker or not.
So, it's a fascinating view into the process of which different standards are decided upon the interfaces.
Matt: Nice.
So, shifting gears a little bit, one of the things that you talked a lot about at the meetup, and again, if anybody's interested in seeing, some of these demos really help, so if you're interesting in hearing more about this, I'd suggest checking out his talks so you can see him demonstrating some of this stuff, but one of the big use cases of Runway that I've seen and that you talked about was that Green Screening, and the stuff you can do there.
And I'd be interested to hear technically, how's that working? What can you tell us about how you're doing that?
You mentioned it a little bit earlier, but I'd be curious to dig in a little bit more.
Anastasis: Yeah. So, just for context, Green Screen is a interactive segmentation tool.
So, what that means is it allows you to give an input video that you upload to Runway, you can mask out the specific subject that you choose and track that over the whole video.
And the use cases for that would be, you might want to separate the subject from the background to bring the subject somewhere else, or you might want to apply specific effects on that subject or a specific color correction.
So, the way Green Screen works, which is entirely in the browser, by the way, is the user first clicks on the subject that they're interested in segmenting, and they can add more and more key frames.
So, they can go to other places on the video, click again on the subject if the masks are not perfectly accurate.
And once they're ready, they can preview how the mask would look on the entire video.
So, behind the scenes, we ran two different models.
The first model is the interactive segmentation model that basically takes the user's click as input, and then generates an initial mask.
So, the moment you click a few times on the subject you want to segment, we basically generate the initial mask just on that frame that you clicked.
And the way we trained that model was that we simulated how users would interact with this interface.
So, we assume that there is a range of users, from users who don't have a lot of time and they want to, with a few clicks, make a really good mask, and users were very detail-oriented and maybe come from the more hardcore rotoscoping world, and those users really care about accuracy and really care about the edges being perfectly aligned around the subject.
So, we trained them all to incorporate a variable amount of clicks and a variable amount of detail of the final mask.
So, in some cases, we had rounds of clicking where the user only made two clicks.
And in some cases we had 10 rounds of clicking involving 100 clicks.
So, by doing that, the idea was to simulate how users would interact with this final interface.
This model is separate than the most common segmentation models, which are either saliency-based, by saliency meaning the most prominent thing in the screen, or semantic segmentation models.
Semantic simulation models are trained to detect a fixed number of categories. So, person, car, dog, cat, et cetera.
But in the case of Green Screen and the kind of uses that we wanted to see in a tool, we wanted to allow arbitrary combinations of objects or objects that a model might not have seen before.
And that was what necessitated this interactive element to the model.
So, that's the first model.
The second model that we call the propagation network is maybe more of interest from a video engineering perspective in that this is applied on every frame of the video.
And it takes the key frames that you've created with the original model into account in basically segmenting every frame in the middle.
So, say you've masked the first frame of video and the last frame of the video, the propagation ends were responsible for generating masks all the way from the first to the last frame, all the frames that you haven't manually segmented.
And the idea being that, unlike traditional video editing and VFX work where you have to operate on every frame of the video, and it's a really manual, really tedious process, we want to allow you to operate on very few frames of the video and have the rest of the process taken care of for you.
And that's not just Green Screen, but all the other tools that we'll be building.
So, in some other tools that we're working with, you want to erase a subject in just one frame and then have the selection you made propagate throughout the rest of the video and have the subject erased throughout the rest of the video.
Or you want to apply some manual retouching on one frame and then have that retouching be correctly tracked through the rest of the video.
So, this is the job of the propagation network.
And in this case, we also face the data solution unlike in the interaction segmentation model.
In this case, the challenge was just generating a sufficient number of examples for the model.
The labeling video is very costly because this is a per pixel annotation.
So, you need every pixel and it has a binary value. Is this in a mask or not?
And it's really expensive to do for, even if you're creating an image segmentation data set, let alone if you're segmenting, say, a 10 second or one minute video at 25 frames per second.
So, the challenge there was, how do we create this video segmentation data set?
And the approach we chose, and it's a combination of different approaches, but first of all, we pursued some collaborations with VFX studios that had their own rotoscoping data.
So, we wanted to serve professional rotoscoping artists.
The way to do it is to basically simulate how that process would look by working directly with VFX studios and collaborating on data sets by receiving assets to some rotoscoping data, and then in return helping them by bringing our models to improve their processes.
Phil: This is amazing, that you're answering the exact questions I was going to go down the path of.
But is there a negative bias there where they want to give you bad data?
Because I think you've just answered it, but they want to give you bad data because they actually care about it being a human manual job?
You don't want a robot to replace your job, right?
But you're saying if you're giving the data back to them then to improve the algorithm. Is that right?
Anastasis: Right. So, the way we see it is that we're not trying to replace creators.
We're trying to augment some of the processes.
And consistent feedback that we hear from, we engage in the user research and as we're trying out new functionality for video tools, is that roughly the video editing can be divided in two parts, the creative part and the manual part.
And the manual part ends up taking 90% of the time, very often. There's very little creativity that goes into it.
So, something like rotoscoping is not something that a lot of people feel very strongly about not being automated because nobody really likes rotoscoping.
Phil: Okay. That makes a lot more sense. I don't want to dig too much into your proprietary information.
What's the order of magnitude scale of the data sets you're looking at or that you trained on specifically?
Anastasis: Yeah. So, that was the biggest challenge that we faced, was generating a large enough dataset.
Because as I mentioned, it's a very expensive process.
I think the collaborations we're pursuing with VFX studios allows us to get very high quality data, but in terms of the amount of data, it's not always enough to get really good results and have the model learn variety of different cases.
So, what we ended up doing was creating synthetic datasets.
And I guess, just to explain the term synthetic data sets in the machine learning community, it's basically creating a data set that might be based on some real examples, but it's basically taking those real examples and creating new examples from them that combine those original examples in some way, or even creating entirely--
One good example is for self-driving research, there is a dataset that uses GTA V, the video game, to generate additional data to train the self-driving algorithms, or even having reinforcement learning models that operate inside the game and learn how to drive a car inside a GTA V. So-
Matt: I want no part of my self-driving algorithm to come from people's driving behavior in GTA V. That's fascinating.
Anastasis: And so one insight we had was that by taking a large number of Green Screen stock footage, where in some ways the mask was already there, it's just applying a chroma key shader to remove the background, and then composing that footage to random videos, we could basically generate a really large number of combinations that were completely made up and created some absurd examples sometimes.
But it allowed us to train the model and get it to a much better level of accuracy because it increased the data we had by one or two orders of magnitude by just finding--
Say if you have 1000 Green Screen footage videos and then 1000 random videos, and then every single combination between the two you can provide as a training example for your model.
Phil: That is absolutely amazing. My mind is blown right now. This is amazing.
You can tell who didn't attend SF Video Tech this month because it starts at 3 AM for some of us, but this is amazing. It's so cool.
Steve: The part that feels like magi is that propagation piece. That feels amazing.
And the classic example is the lightsaber painting, right? Is that the type of thing that would apply here, essentially?
Anastasis: I'm not familiar with the lightsaber painting.
Steve: So, the understanding of in the original Star Wars movies, they would paint every single frame.
They would paint the lightsaber low onto the sword every single frame, right?
Is that something that can be done today or could be in the future with the same type of propagation technology?
Anastasis: Right. I think that's a really good example because also what everyone was using in place of the lightsaber when they were shooting Star Wars is not something you would find in the existing dataset of real world videos.
So, you need to create a real large number of examples to cover cases that might not be covered by a smaller set of examples.
The idea is that, yeah, you would, in that case, segment the lightsaber, and then you could generate an alpha mask that separates that object and then bringing it to something like After Effects and then proceed with creating more effects around it.
Matt: That's so cool.
And I totally hear you because when I think about They Took Our Jerbs type stuff, it's like, "Who does want to just sit and look frame by frame and replace a stick with a lightsaber?"
That'd be really cool for about three frames and then just be miserable from then on.
So, yeah. I mean, I can totally see how quickly this would open up people's time to be able to build more interesting things as opposed to some schlub having to go frame by frame through a two hour long video.
Phil: I think the fascinating thing is, in the specific example Heff was referring to, that was physical painting on the frame.
That wasn't even pixel painting. That's physical paint on an overhead on the frame.
Steve: We've come a long way.
Phil: Matt's mind is blown now.
Matt: Last thing I wanted to pick your brain on a little bit here is, during the meetup talk you mentioned that ultimately what gets delivered to the editor's client in the browser is HLS, which is fascinating.
Can you talk a little bit? How?
Anastasis: Yeah.
So, one of the things we're trying to do at Runway is we want to create those new views of a video.
So, be able to take one video that the user has uploaded to the platform and then be able to automatically generate the depth information from the video, optical flow information, segmentation of different objects in the video.
In all those different streams we want have a framework that can make it very easy to be able to request them from the client in order to build some effects on the browser or to further process them for other tasks.
For example, to understand the content of the video, different types of video.
And in order to build a unified framework so we don't reinvent the wheel, on every model that we bring to the platform we created this more general streaming STK, as we call it internally, which is a way to wrap the functionality of different machinery models into a common interface that makes it possible to request them as HLS streams.
So, the way you request the main content of the video's HLS stream is very similar to the way you will request the depth information for that same video.
And instead of having everything statically available, very often this is processed on the fly.
So, we need to make sure that the models that generate the depth information or the segmentation run quickly enough that we can get the results back to the client to be played in segments, as is common with HLS, fast enough so that we don't break the video playback experience.
So, this is something that we worked on for some time. It's to use HLS maybe not in the way it was intended.
As I mentioned, I came to the video engineering world very recently and I thought, "Oh, HLS is a protocol for requesting segments of a video."
And maybe those segments don't already need to be available when you request them.
Maybe if you have a machine learning model, you can intervene on the fly to process them in different ways and return that processed data to the user.
And maybe on the client, if you request all those different streams, you can combine them into interesting ways to apply new effects on them.
So, if you have the depth information, for example, you can add a box effect that adds a better blur effect, basically.
If you have the optical flow information, you can re-time the video.
So, you can get intermediate frames between two frames of the video and create a slow motion effect.
And instead of having those be separate functionalities and end points that we have to deploy in a separate way, HLS provided this common interface that made it possible to process the video in all kinds of ways with machine learning models.
Matt: How hacked is this?
Because I assume, in this idea, you probably really don't care about the adaptive nature of HLS.
You probably care more about the segmented stuff.
So, are all your child manifests actually all these different variants or are each of these its own-- You see what I'm saying?
Anastasis: Yeah. We still handle adaptive bitrate because we might have clients with different kinds of bandwidth profiles.
So, they might have a slower connection.
And so we still try to generate them at the bitrate where they would be delivered to the client faster.
But yeah, the main concern really, I guess, the bottleneck, unlike in more common video engineering use cases where the bottleneck is maybe the bandwidth of the client.
In this case, the bottleneck is usually the machine learning inference part.
So, we try to get that as low as possible, so then we only have to deal with that traditional video engineering challenges.
Matt: Got it. Awesome. Well, thanks, Anastasis. This was fantastic conversation.
Again, if anybody's interested in seeing more of this stuff, he walked through with images and animations for how some of this stuff worked in the SF Video talk, so youtube.com/sfvideotechnology, if you want to go see that one.
It was from the July SF Video meetup. Again, reminder that Demuxed is online. Yeah.
This weekend I'm definitely going to take some of my videos of my two-year-old daughter and do the whole thing where she's jumping across lava or whatever else.
She'll love it. It'll probably freak her out, honestly, but I'm excited to try.
Cool. Well, it's runwayml.com, right?
Anastasis: Yeah. Go to runwayml.com. Yeah. Please try it out and tell us what you think.
And we're growing our team, especially on the video engineering side, so if you're interested and intrigued by the challenges that I just described, definitely feel free to reach out.
Matt: Cool. And as always, again, we're trying to schedule more of these conversations maybe a little bit more often than once every six months.
So, if anybody knows of topics they want us to cover or people that want to hop on to have a chat, reach out.
You can find us on @demuxed on Twitter, and MCC, PHIL, HEFF on video-dev.
Just all caps stuff. video-dev.org is where that is.
Phil: Info@demuxed.com.
Matt: Info@demuxed.com is another one.
Steve: I think we have given them enough.
Matt: All right. Thanks everybody.
Subscribe to Heavybit Updates
Subscribe for regular updates about our developer-first content and events, job openings, and advisory opportunities.
Content from the Library
Demuxed Ep. #20, Demuxed 2023 Conference Preview
In episode 20 of Demuxed, Matt and Phil share a special preview of the Demuxed 2023 Video Conference. Together they discuss the...
Demuxed Ep. #19, Password Sharing and Patent Conflicts
In episode 19 of Demuxed, Matt, Phil, and Steve discuss video meetups, particularly the return of IRL meetups and the...
Demuxed Ep. #17, JavaScript HLS with Rob Walch of JW Player
In episode 17 of Demuxed, Matt, Phil, and Heff speak with Rob Walch. They discuss the JavaScript library hls.js, tactics for...